Satellite Early Anomaly Detection Using an Advanced Transformer Architecture for Non-Stationary Telemetry Data
IEEE Transactions on Consumer Electronics(2024)
摘要
Data-driven methodologies are indispensable for early satellite anomaly detection. However, their effectiveness in capturing temporal dependencies within non-stationary telemetry data is still not ideal. In the field of satellite anomaly detection using reconstruction theory, this limitation can make it unclear whether the distribution shifts of reconstruction errors are caused by inadequate training or the actual emergence of real anomalies. To address these challenges, we propose the Seasonal Decomposition Cross Attention Transformer (SDCA-Former), a new Transformer architecture specifically designed to effectively extract temporal dependencies in non-stationary satellite telemetry data, thereby enabling more precise early warning of satellite anomalies. Firstly, building upon the foundational architecture of the Vanilla Transformer, the SDCA-Former integrates a series of enhancements, including the Temporal Convolutional Network-based Position Encoding (TCNPE), Encoder architecture refinements, and the Decoder utilizing SDCA modules. Secondly, we developed a comprehensive satellite anomaly detection framework centered on the SDCA-Former, including technical components such as telemetry data feature engineering, anomaly scoring, and determination of anomaly thresholds. Lastly, we verified the effectiveness and superiority of the proposed method on a dataset composed of telemetry data from a Geostationary Earth Orbit (GEO) satellite. Notably, our approach achieved an early warning for anomalies up to four months in advance.
更多查看译文
关键词
Transformer,anomaly detection,reconstruction discrepancy,time series forecasting,GEO satellite telemetry data,non-stationary
AI 理解论文
溯源树
样例
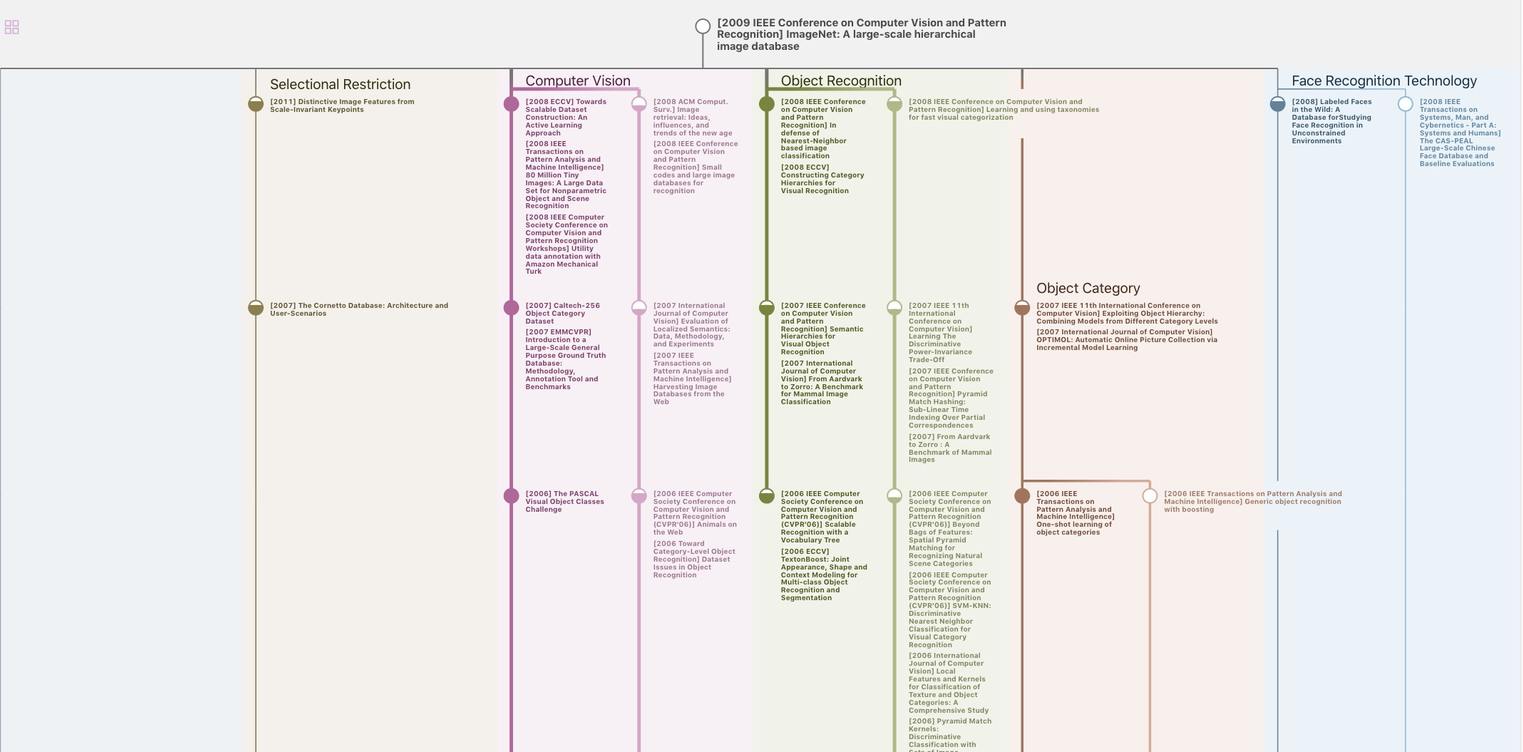
生成溯源树,研究论文发展脉络
Chat Paper
正在生成论文摘要