Improve interpretability of Information Bottlenecks for Attribution with Layer-wise Relevance Propagation.
2023 IEEE International Conference on Big Data (BigData)(2023)
摘要
Researchers have developed various visualization techniques, such as attribution maps, to understand which parts of an input contribute most to a model’s decision. However, existing methods often produce disparate results and may lack human-perceptual interpretability. In this work, we propose Relevance-IBA, a novel approach that combines the strengths of Information Bottleneck Attribution (IBA) and Layer-wise Relevance Propagation’s (LRP) method to estimate more accurate and human-perceptually interpretable attribution maps. Our method accentuates the contours and subtle details of the identified object, making the model’s decisions more intuitively understandable. Additionally, we introduce a segmentation-oriented evaluation technique, which assesses the capacity of interpretability methods by emphasizing the most important pixels within an object’s boundaries. We benchmark Relevance-IBA against various methodologies, including DeepLIFT, Integrated Gradients, Guided-BP, Guided-GradCAM, IBA, and InputIBA. Our results indicate that Relevance-IBA not only boosts attribution accuracy but also prioritizes human-perceptual clarity, making it a valuable tool for interpreting complex model behaviors.
更多查看译文
关键词
IBA,Attribution maps,interpretability,LRP,human-perceptual
AI 理解论文
溯源树
样例
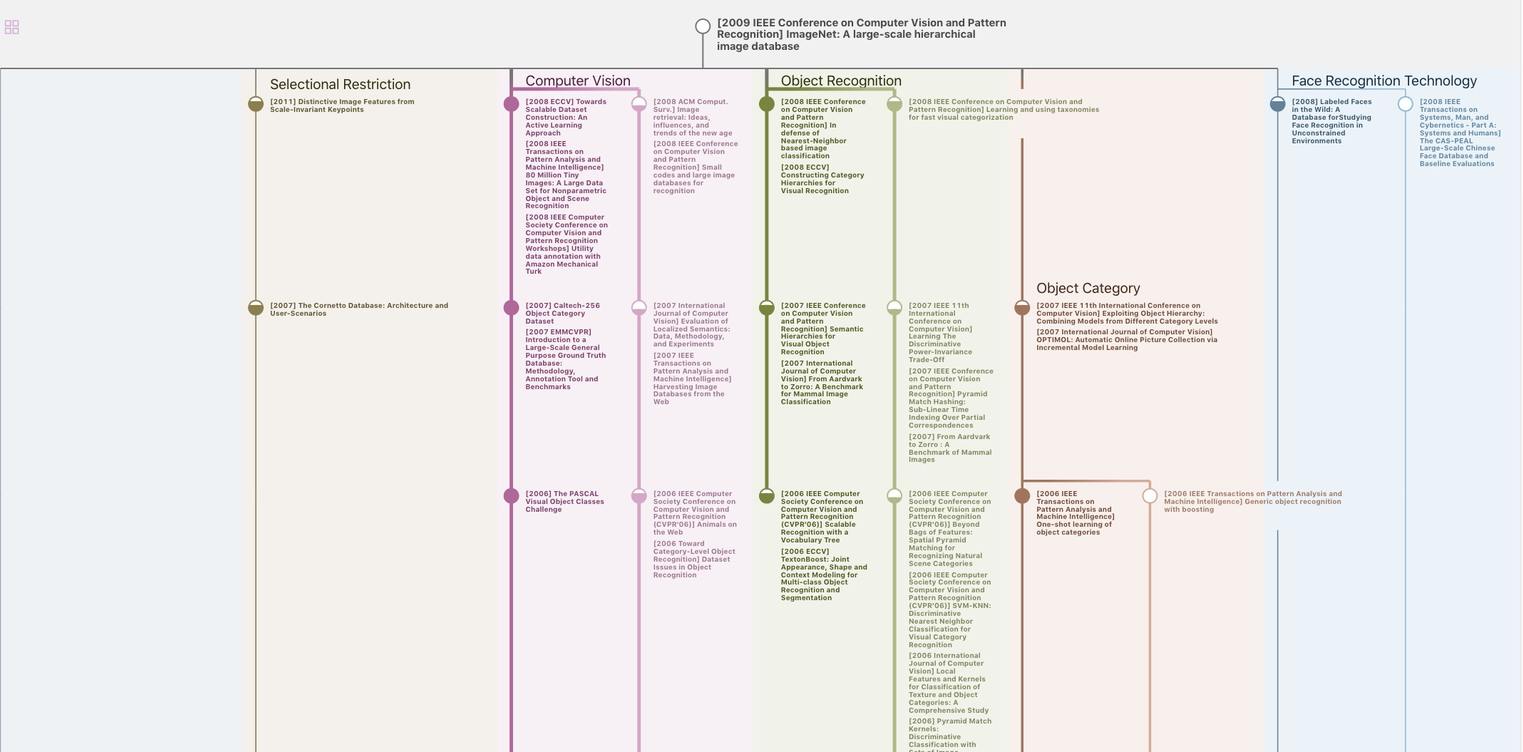
生成溯源树,研究论文发展脉络
Chat Paper
正在生成论文摘要