GCSA: A New Adversarial Example-Generating Scheme Towards Black-Box Adversarial Attacks
IEEE Transactions on Consumer Electronics(2024)
摘要
This paper focuses on the transferability problem of adversarial examples towards black-box attack scenarios wherein model information such as the neural network structure is unavailable. To tackle this predicament, we propose a new adversarial example-generating scheme through bridging a data-modal conversion regime to spawn transferable adversarial examples without referring to the substitute model. Three contributions are mainly involved: i) we figure out an integrated framework to produce transferable adversarial examples through resorting to three components, i.e., image-to-graph conversion, perturbation on converted graph and graph-to-image inversion; ii) upon the conversion from image to graph, we pinpoint critical graph characteristics to implement perturbation using gradient-oriented and optimization-oriented adversarial attacks, then, invert the perturbation on graph into the pixel disturbance correspondingly; iii) multi-facet experiments verify the reasonability and effectiveness with the comparison to three baseline methods. Our work has two novelties: first, without referring to the substitute model, our proposed scheme does not need to acquire any information about the victim model in advance; second, we explore the possibility that inferring the adversarial features of image data through drawing support from network/graph science. In addition, we present three key issues worth deeper discussion, along with these open issues, our work deserves more studies in future.
更多查看译文
关键词
deep learning,adversarial examples,black-box adversarial attack,transferability
AI 理解论文
溯源树
样例
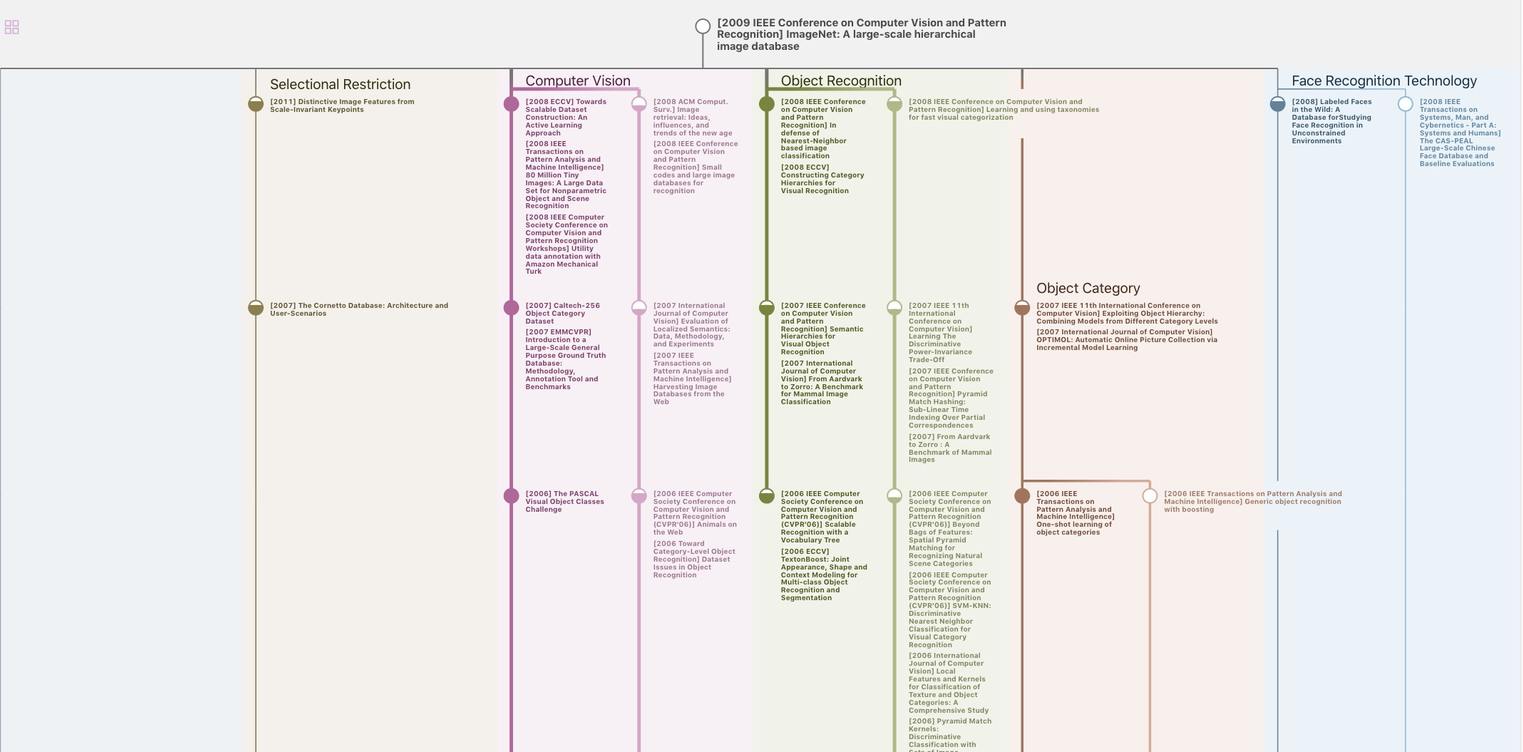
生成溯源树,研究论文发展脉络
Chat Paper
正在生成论文摘要