Autoencoders and Generative Adversarial Networks for Imbalanced Sequence Classification.
2023 IEEE International Conference on Big Data (BigData)(2023)
摘要
Generative Adversarial Networks (GANs) have been used in many different applications to generate realistic synthetic data. We introduce a novel GAN with Autoencoder (GAN-AE) architecture to generate synthetic samples for variable length, multi-feature sequence datasets as existing GAN models cannot generate synthetic data and associated labels. In this model, we develop a GAN architecture with an additional autoencoder component, where recurrent neural networks (RNNs) are used for each component of the model in order to generate synthetic data to improve classification accuracy for a highly imbalanced medical device dataset. In addition to the medical device dataset, we also evaluate the GAN-AE performance on two additional datasets and demonstrate the application of GAN-AE to a sequence-to-sequence task where both synthetic sequence inputs and sequence outputs must be generated. To evaluate the quality of the synthetic data, we train encoder-decoder models both with and without the synthetic data and compare the classification model performance. We show that a model trained with GANAE generated synthetic data outperforms models trained with synthetic data generated both with standard oversampling techniques such as SMOTE and Autoencoders as well as with state of the art GAN-based models.
更多查看译文
关键词
Generative Adversarial Networks,Medical Devices,Variable Length,Data Generation,Recurrent Neural Network,Imbalanced Datasets,Improve Classification Accuracy,Synthetic Sequences,Medical Datasets,Generative Adversarial Networks Model,Synthetic Data Generation,Generative Adversarial Network Architecture,Synthetic Inputs,Sequencing Data,Training Set,Training Data,Multivariate Data,Imbalanced Data,Anomaly Detection,Minority Class,Average F1-score,Seq2seq Model,Sequence Labeling,Unbalanced Data,Autoencoder Model,Weighted Loss Function,Straightforward Application,Synthetic Training Data,Minority Samples,Synthetic Technique
AI 理解论文
溯源树
样例
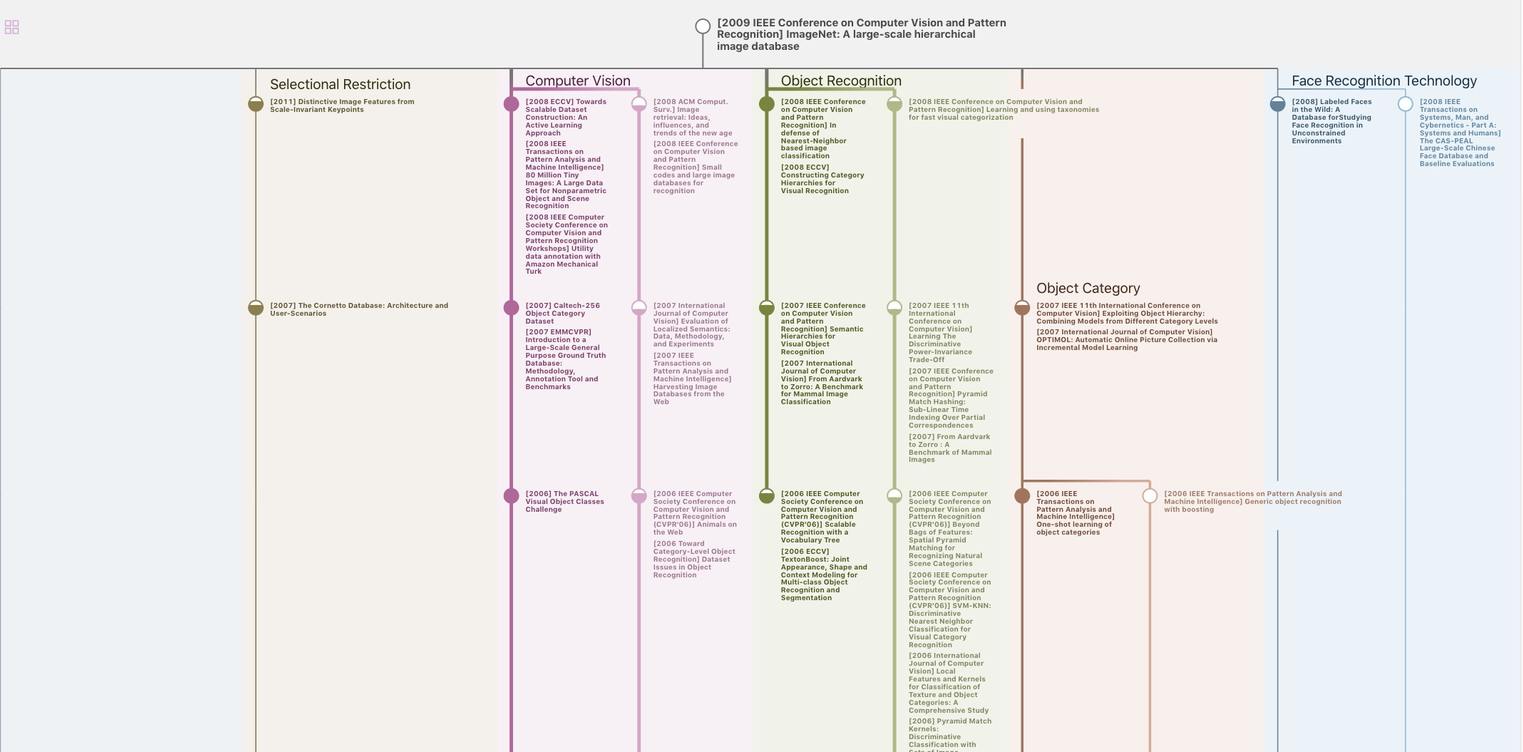
生成溯源树,研究论文发展脉络
Chat Paper
正在生成论文摘要