Efficient Dynamic Federated Learning for Imbalanced Data.
2023 IEEE International Conference on Big Data (BigData)(2023)
摘要
With the advent of data driven era, new challenges have emerged that can hinder their efficient and reliable utilization. At the center of this era is data. However, public, and unrestricted access to data may pose privacy and security threats. Federated learning methods have proposed to address this problem. While the main concern in federated learning has been preserving privacy of data and maintaining the efficiency of centralized learning methods, the structure and distribution of data has not been studied sufficiently. In this paper, we present a federated machine learning approach capable of accommodating imbalanced datasets. Our method dynamically adjusts the learned parameters to prevent biased classifications. Our empirical results indicates that the proposed method managed to improve accuracy by 22%.
更多查看译文
关键词
Federated Learning,Imbalanced Data,Machine Learning
AI 理解论文
溯源树
样例
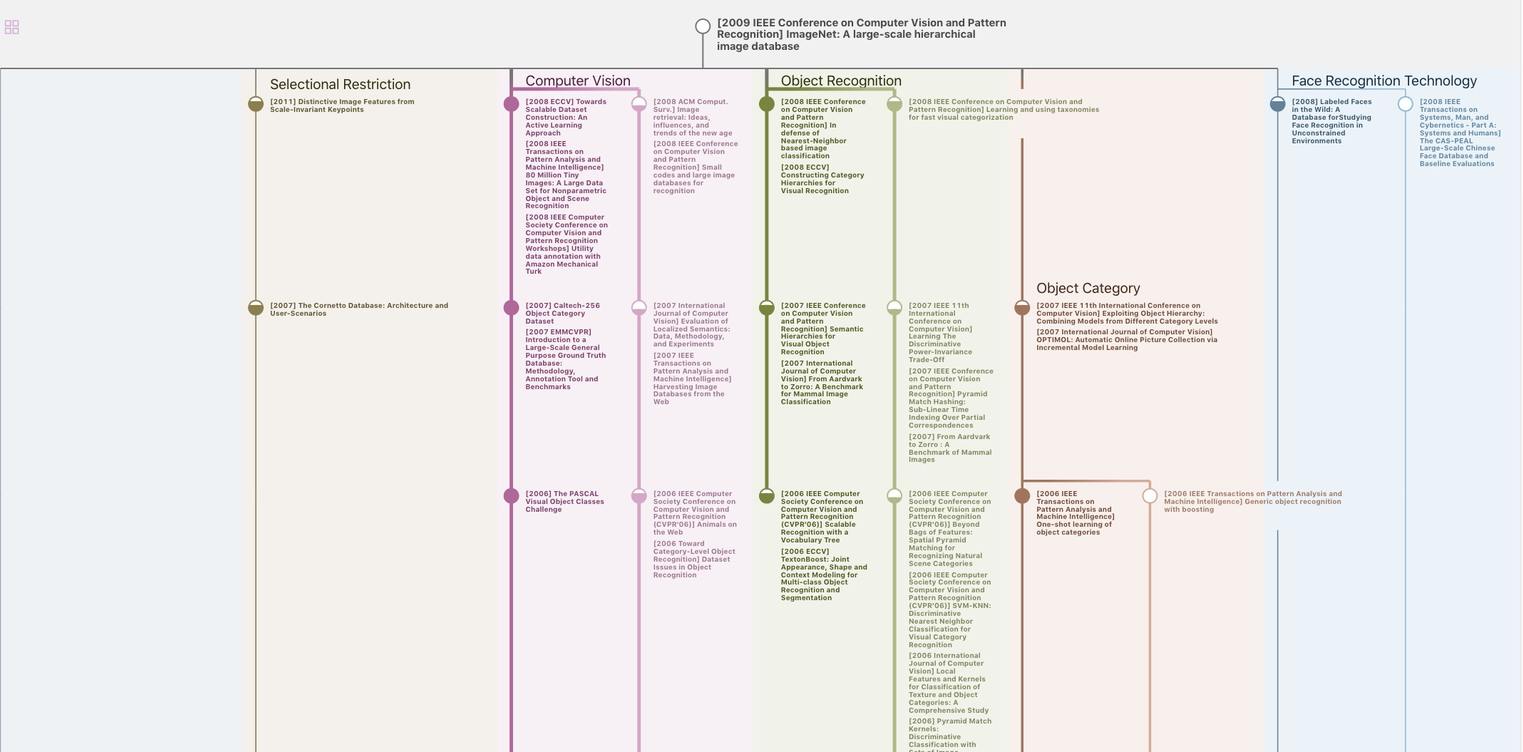
生成溯源树,研究论文发展脉络
Chat Paper
正在生成论文摘要