From Satellites to Fields: Machine Learning Applications for Prediction of Corn Production Using NDVI, Precipitation and Land Surface Temperature for Large Producer Countries.
2023 IEEE International Conference on Big Data (BigData)(2023)
摘要
In this work-in-progress study, we aim to determine the predictors of corn production in Iowa (United States), Heilongjiang (China), Mato Grosso (Brazil), Cordoba (Argentina), and Poltava Oblast (Ukraine). The effectiveness of predicting annual corn production using precipitation and land surface temperature (LST) values obtained from the Earth Engine tool, and Moderate Resolution Imaging Spectroradiometer (MODIS) Normalised Difference Vegetation Index (NDVI) values obtained from the National Aeronautics and Space Administration (NASA) Global Inventory Monitoring and Modelling Studies (GIMMS) Global Agricultural Monitoring System, is examined. A comparison is conducted between multiple linear regression, ridge regression, and lasso regression models. The highest adjusted R
2
values are found in order to identify the optimal model in which the corn yield variance is explained by a combination of variables including the year, NDVI sample and anomaly values, precipitation, and LST. The results show that corn production values in Iowa, Heilongjiang, Mato Grosso, Cordoba, and Poltava Oblast can best be predicted using lasso regression models with adjusted R
2
values of 0.841, 0.933, 0.847, 0.854, and 0.860 respectively.
更多查看译文
关键词
Agriculture,Machine Learning,Yield estimation,Earth Observational Data,NDVI
AI 理解论文
溯源树
样例
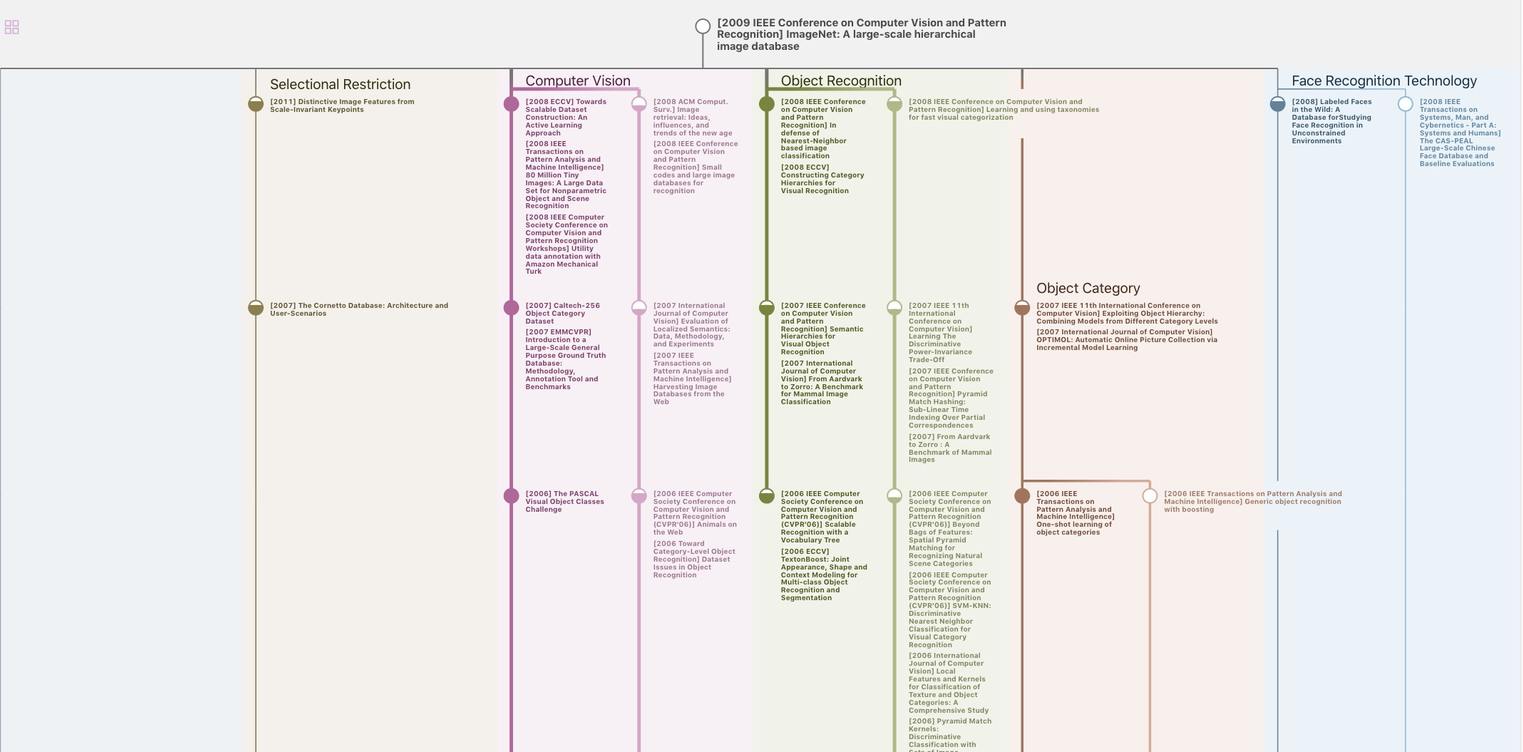
生成溯源树,研究论文发展脉络
Chat Paper
正在生成论文摘要