Analysis and Forecasting of Carbon Emission in SAARC Countries using Attention-based LSTM.
2023 IEEE International Conference on Big Data (BigData)(2023)
摘要
Climate change and global warming are urgent environmental issues demanding immediate action to safeguard future generations. The major contributor to the greenhouse effect, carbon dioxide $\left(\mathrm{CO}_{2}\right)$, primarily originates from industrial and transportation fossil fuel combustion. International agreements, like the Paris Agreement, call for a 30-35% reduction in CO
2
emissions compared to 2005 levels. This research aims to predict CO
2
emissions and raise awareness among SAARC nations and governments about the increasing trend. We introduce a novel predictive framework using Attention-based Long Short-Term Memory (A-LSTM) for CO
2
emissions analysis. The Attention mechanism assigns variable weights to input data, facilitating indirect connections between LSTM outputs and pertinent inputs. This enhances resource allocation in the A-LSTM model, overcoming computational constraints. We integrate input parameters encompassing CO
2
emissions from land-use changes, oil, natural gas, and coal combustion to forecast CO
2
emissions and correlate them with population and per capita GDP. Our comparative analysis conclusively demonstrates the superior performance of A-LSTM models over baseline LSTM models when applied to the CO
2
emission dataset sourced from Our World in Data (OWID) and World Bank Indicator database. Specifically, the LSTM model registers a MAPE of 24.968 and an RMSE of 0.34, whereas the Attention-based LSTM model showcases a marked improvement of 57% with a considerably lower MAPE of 10.5902 and an RMSE of 0.107.
更多查看译文
关键词
Climate Change,Green House Gas,Long Short Term Memory,Attention,CO2 Emission
AI 理解论文
溯源树
样例
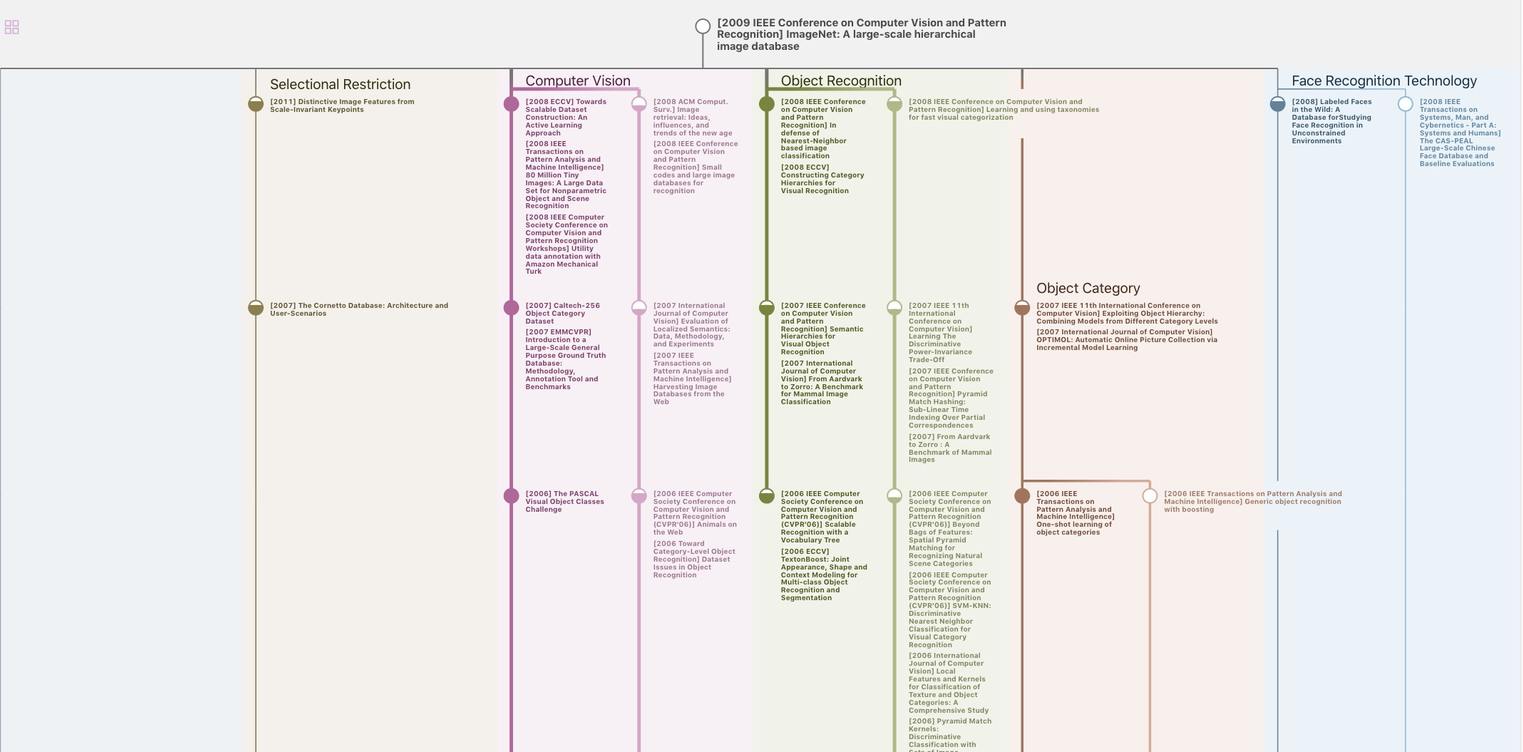
生成溯源树,研究论文发展脉络
Chat Paper
正在生成论文摘要