Graph-Based Approach for European Law Classification.
2023 IEEE International Conference on Big Data (BigData)(2023)
摘要
Deep learning, owing to its transformative influence across a myriad of sectors, has recently made its foray into the legal domain, instigated by the surge in digitization. Among the multitude of applications in this space, legal document classification emerges as a pivotal yet complex undertaking. Legal texts, characterized by unique domain-centric semantics and intricate linguistic patterns, necessitate precision-driven classification systems for numerous practical implications. This paper illuminates the challenges and opportunities in automating the classification of European Union (EU) legal documents, emphasizing the interrelationships among statutes and the hierarchical nature of legal references. In this context, we introduce a novel graph data modeling technique that adeptly marries content-centric indicators with the relational dynamics inherent among diverse legal documents. Central to our approach is a framework that melds text embeddings with graph neural networks for the classification of legal documents aligned with their subject-based directories. Empirical evaluations on the EU law dataset underline the efficacy of our model across varying granularities, from general thematic categories to intricate subtopics. This endeavor not only augments the comprehensibility and accessibility of EU jurisprudence but also holds significant implications across regulatory compliance, legal research, and policy formulation, underscoring the potential of deep learning in reshaping legal paradigms.
更多查看译文
关键词
legal document classification,natural language processing,graph neural networks
AI 理解论文
溯源树
样例
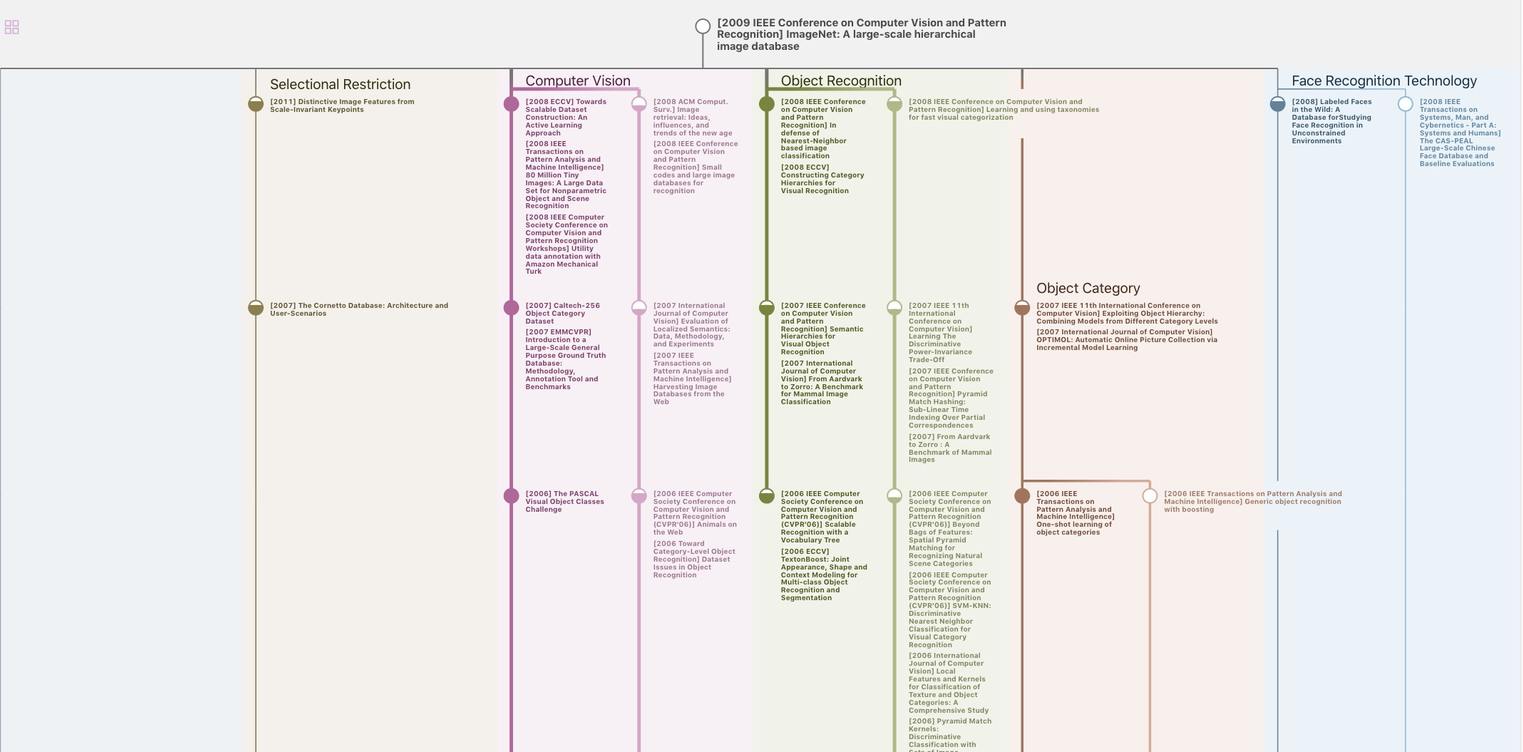
生成溯源树,研究论文发展脉络
Chat Paper
正在生成论文摘要