GLEm-Net: Unified Framework for Data Reduction with Categorical and Numerical Features.
2023 IEEE International Conference on Big Data (BigData)(2023)
摘要
In the era of Big Data, effective data reduction through feature selection is of paramount importance for machine learning. This paper presents GLEm-Net (Grouped Lasso with Embeddings Network), a novel neural framework that seamlessly processes both categorical and numerical features to reduce the dimensionality of data while retaining as much information as possible. By integrating embedding layers, GLEm-Net effectively manages categorical features with high cardinality and compresses their information in a less dimensional space. By using a grouped Lasso penalty function in its architecture, GLEm-Net simultaneously processes categorical and numerical data, efficiently reducing high-dimensional data while preserving the essential information. We test GLEm-Net with a real-world application in an industrial environment where 6 million records exist and each is described by a mixture of 19 numerical and 7 categorical features with a strong class imbalance. A comparative analysis using state-of-the-art methods shows that despite the difficulty of building a high-performance model, GLEm-Net outperforms the other methods in both feature selection and classification, with a better balance in the selection of both numerical and categorical features.
更多查看译文
关键词
data reduction,feature selection,neural network,categorical features,embeddings
AI 理解论文
溯源树
样例
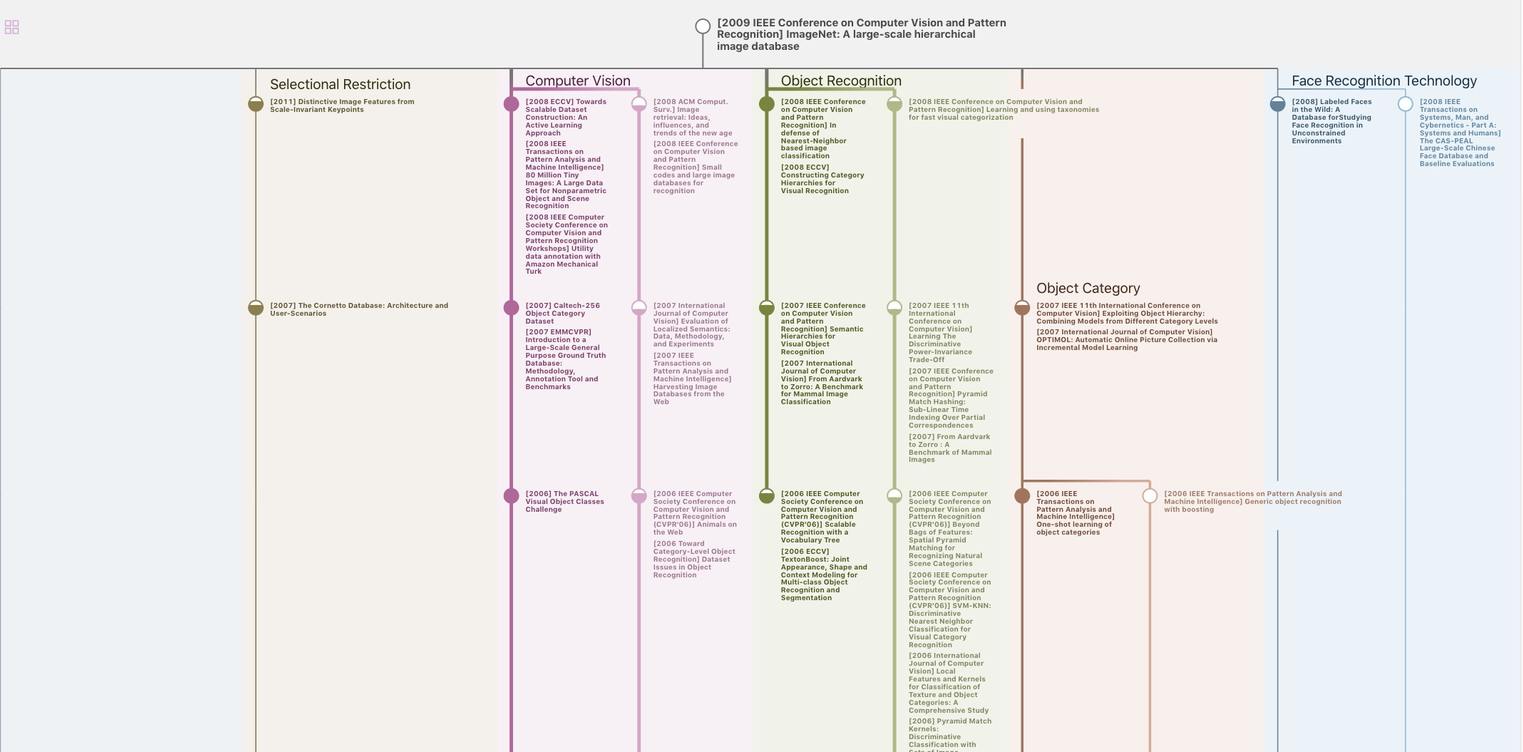
生成溯源树,研究论文发展脉络
Chat Paper
正在生成论文摘要