GAME: Improving Efficiency and Effectiveness of Knowledge-Graph Rule Mining via Data Reduction.
2023 IEEE International Conference on Big Data (BigData)(2023)
摘要
Knowledge graphs have been growing in popularity in recent years, due to their successful use in many data-analytics and knowledge-discovery tasks. As the sizes of domain graphs continue to grow, they can become too large to be processed efficiently by downstream applications. It is thus indispensable in many cases to aim to address the efficiency issue by reducing knowledge-graph sizes via summarization. At the same time, to provide quality results, that is, to be effective, on the graph summaries, many downstream analytics and knowledge-discovery tasks require the summaries to retain as much information from the original graph as possible. It turns out that state-of-the-art data-reduction approaches may generate task-specific or lossy summaries, which may present challenges for the effectiveness of downstream analytics in some cases. As a result, it is important to study trade-offs between the performance and accuracy of graph summarizations for analytics and knowledge discovery.In this paper, in the scope of knowledge-graph size reduction via summarization, we consider the trade-off between the performance and accuracy of one type of knowledge-graph analytics, inference-rule mining, on the summarized graphs as compared with the original graphs. Toward addressing the tradeoff challenge, we introduce a domain- and task-independent knowledge-graph summarization approach called GAME for generating reduced abstract knowledge graphs from a given graph. Our preliminary experimental results suggest that our GAME approach can preserve as much information from the given (original) graph as possible for the purpose of rule mining, while potentially significantly improving the rule-mining efficiency. We anticipate that the knowledge-graph reduction provided by the proposed GAME approach can enable efficient, yet effective, results for diverse applications and use cases.
更多查看译文
关键词
Knowledge-graph summarization,information-preserving data reduction,knowledge-graph deduplication,abstract knowledge graphs,inference-rule mining
AI 理解论文
溯源树
样例
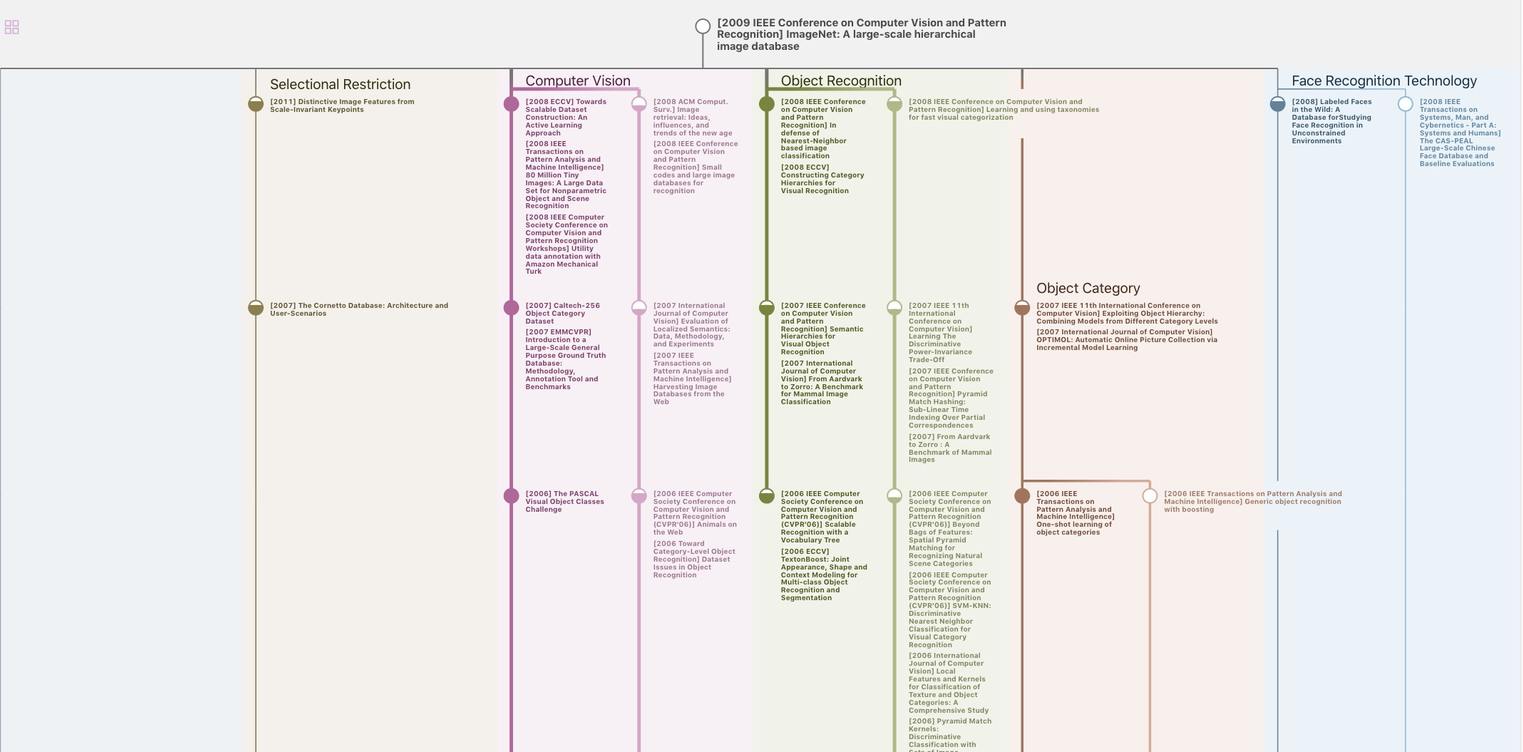
生成溯源树,研究论文发展脉络
Chat Paper
正在生成论文摘要