Integrating an LSTM framework for predicting ankle joint biomechanics during gait using inertial sensors
COMPUTERS IN BIOLOGY AND MEDICINE(2024)
摘要
The ankle joint plays a crucial role in gait, facilitating the articulation of the lower limb, maintaining foot-ground contact, balancing the body, and transmitting the center of gravity. This study aimed to implement long shortterm memory (LSTM) networks for predicting ankle joint angles, torques, and contact forces using inertial measurement unit (IMU) sensors. Twenty-five healthy participants were recruited. Two IMU sensors were attached to the foot dorsum and the vertical axis of the distal anteromedial tibia in the right lower limb to record acceleration and angular velocity during running. We proposed a LSTM-MLP (multilayer perceptron) model for training time-series data from IMU sensors and predicting ankle joint biomechanics. The model underwent validation and testing using a custom nested k-fold cross-validation process. The average values of the coefficient of determination (R2), mean absolute error (MAE), and mean squared error (MSE) for ankle dorsiflexion joint and moment, subtalar inversion joint and moment, and ankle joint contact forces were 0.89 +/- 0.04, 0.75 +/- 1.04, and 2.96 +/- 4.96 for walking, and 0.87 +/- 0.07, 0.88 +/- 1.26, and 4.1 +/- 7.17 for running, respectively. This study demonstrates that IMU sensors, combined with LSTM neural networks, are invaluable tools for evaluating ankle joint biomechanics in lower limb pathological diagnosis and rehabilitation, offering a cost-effective and versatile alternative to traditional experimental settings.
更多查看译文
关键词
Computational musculoskeletal modelling,Inertial measurement unit (IMU) sensors,Long short-term memory (LSTM),Ankle joint biomechanics,Walking,Running
AI 理解论文
溯源树
样例
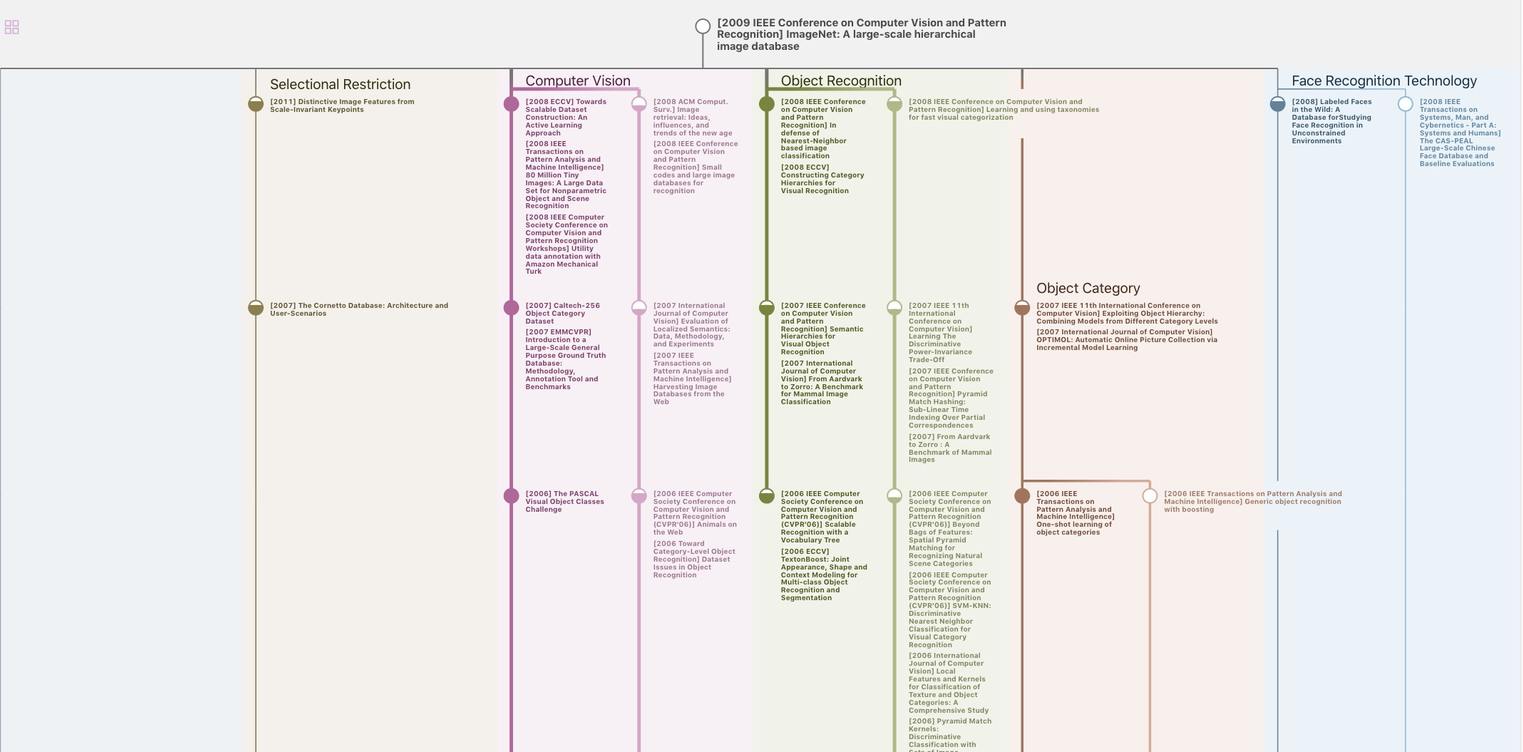
生成溯源树,研究论文发展脉络
Chat Paper
正在生成论文摘要