Defending Federated Learning Against Model Poisoning Attacks.
2023 IEEE International Conference on Big Data (BigData)(2023)
摘要
Federated Learning (FL) is a machine learning framework that allows multiple clients to contribute their data to a single machine learning model without sacrificing their privacy. Although FL addresses some security issues, it is still susceptible to model poisoning attacks where malicious clients aim to corrupt the main learning model by sending poisoned updates. Byzantinerobust methods are defenses that aim to prevent corruption of the main learning model by tolerating a certain number of malicious clients. However, they can only resist a small number of malicious clients. This proposed method uses Gap statistics to determine the optimal number of clusters to cluster clients. This will improve the detection accuracy of malicious clients while preventing the mislcassification of honest clients in a Federated Learning setting. Our experiments so far show us an improvement over the base method.
更多查看译文
关键词
Machine Learning,Federated Learning,Clustering,Privacy and Security,Model Poisoning Attack.
AI 理解论文
溯源树
样例
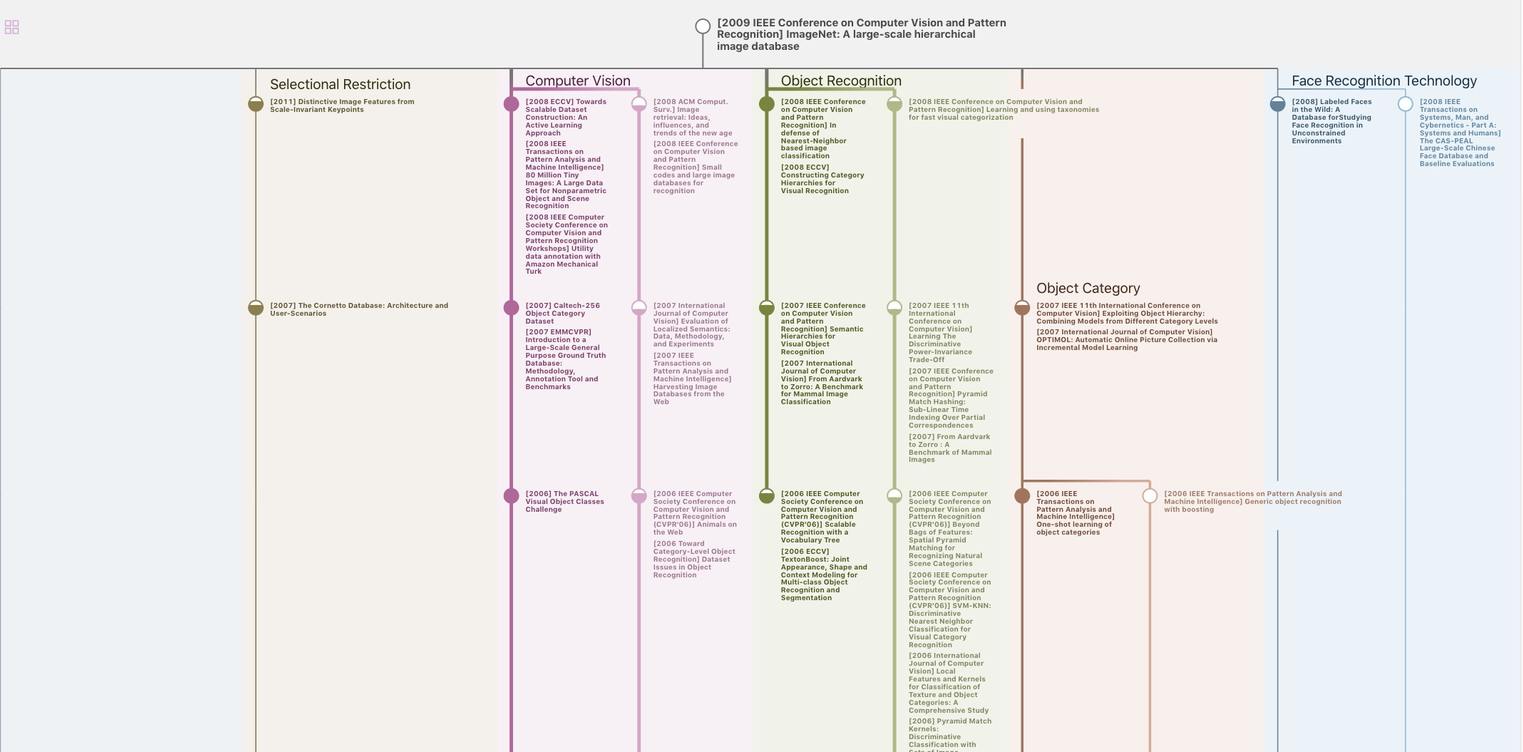
生成溯源树,研究论文发展脉络
Chat Paper
正在生成论文摘要