Energy-Aware Query Processing: A Case Study on Join Reordering.
2023 IEEE International Conference on Big Data (BigData)(2023)
摘要
Analytic processing systems have been traditionally designed to optimize time performance, leaving energy as a secondary aspect. More recently, during the past decade, there has been a growing interest in addressing the energy efficiency of analytics and in particular query processing (QP), our focus in this article. Numerous solutions, spanning both software and hardware approaches, have been proposed in database systems, but they have important limitations: (i) They were designed for old QP architectures, (ii) they do not consider emerging QP AI trends, such as learned query plans and hybrid QP, and (iii) they lack a well-defined framework with clear steps, which can be applied in a modern data science ecosystem. With such reasons in mind, we introduce a general framework that will help researchers and industry practitioners in addressing energy-efficiency challenges. Our framework, named ${SATM}^{2}V$, integrates five major steps: (1) assessing public sentiment and alerting analysts on the real impact of data science on decarbonization, (2) conducting “under the hood” energy consumption audits to identify energy-hungry components, (3) turning on/off and tuning parameters of components to understand their contribution to energy savings. (4) developing models and measurement techniques for quantifying energy consumption, (5) developing and executing tactics for energy saving. We then turn our attention to database systems and identify relational join processing as a representative energy consumption example. QP becomes particularly difficult when dealing with queries involving multiple join operations since join ordering is known to be an NP-hard problem, whose optimal solution remains an open problem. We apply our solution framework to the specific case of hybrid QPs, studying the impact of various join ordering optimization techniques on energy efficiency. Extensive experiments are conducted using the well-known Join Order Benchmark dataset to evaluate the effectiveness and tradeoffs of several query optimization techniques on time and energy consumption.
更多查看译文
关键词
Energy Consumption,Energy Efficiency,Energy Conservation,Decarbonization,Machine Learning,Deep Learning,Optimism,Learning Models,Learning Algorithms,Service Quality,Performance Of Algorithm,Data Center,Machine Learning Techniques,Training Phase,Rate Of Return,Internet Of Things,Simulated Annealing,Energy Usage,Joining Tree,Execution Plan,Monte Carlo Tree Search,Iterative Improvement,Deep Trees,Potential Of Artificial Intelligence,Enhance Energy Efficiency,European Regional Development Fund,Objective Components,Big Data,Conference Of The Parties,Monte Carlo Tree
AI 理解论文
溯源树
样例
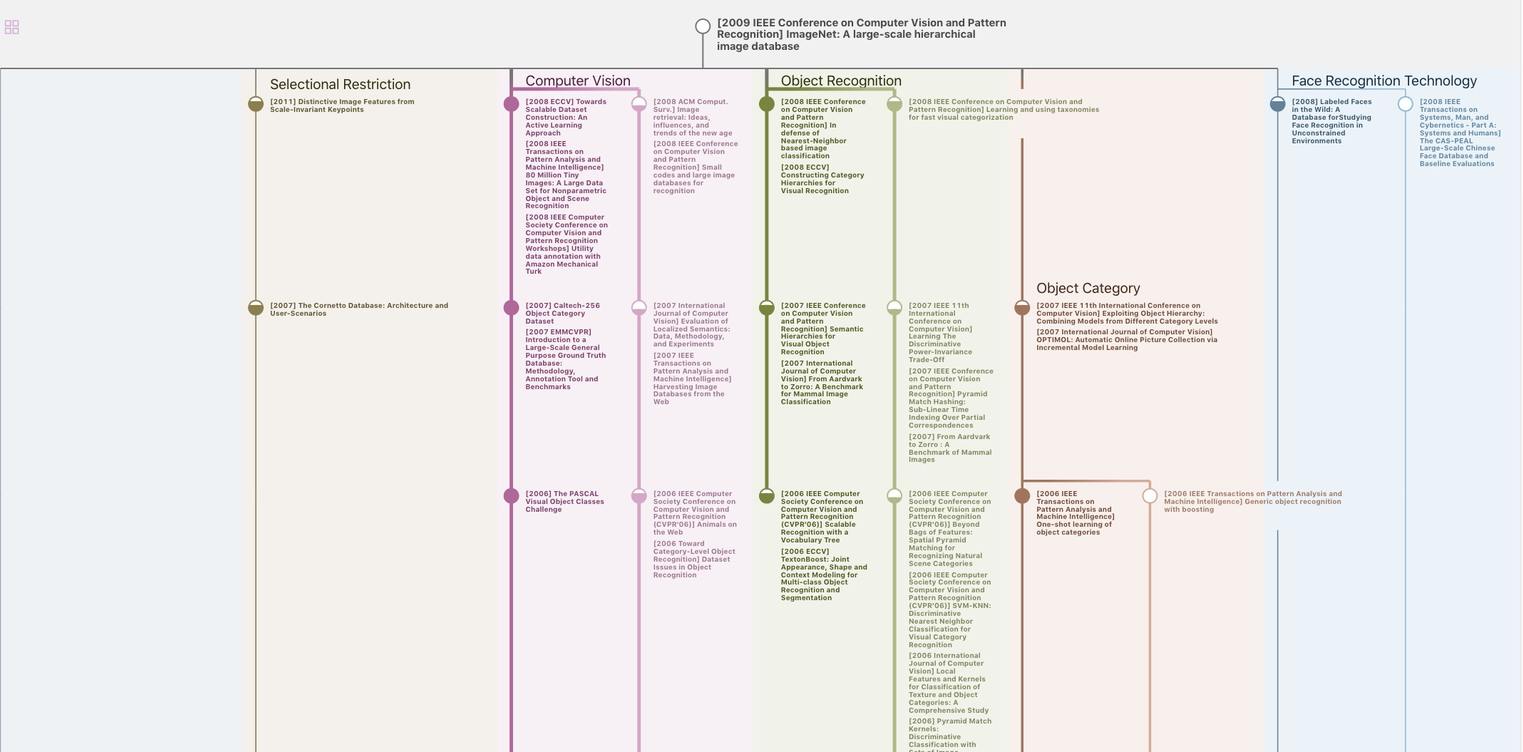
生成溯源树,研究论文发展脉络
Chat Paper
正在生成论文摘要