Graph Neural Networks for Entity Matching.
2023 IEEE International Conference on Big Data (BigData)(2023)
摘要
Data integration still remains largely rule-driven and lacks universal automation. In this work, we propose a general approach to modeling and integrating entities from structured data, such as relational databases, as well as unstructured sources, such as free text from news articles. Our approach is designed to explicitly model and leverage relations between entities, thereby using all available information and preserving as much context as possible. This is achieved by combining siamese and graph neural networks to propagate information between connected entities. We evaluate our method on the task of integrating data about business entities and demonstrate that it outperforms standard rule-based systems, as well as other deep-learning approaches that do not leverage any inductive bias towards the use of graph-based representations.
更多查看译文
关键词
Graph Neural Networks,Entity Matching,Deep Learning,Data Integration,Relational Database,Siamese Network,Business Entities,Training Set,Validation Set,Multilayer Perceptron,Latent Space,Nodes In The Graph,Exact Match,Graph Structure,Representation Of Distribution,Training Examples,Graph Convolutional Network,Node Representations
AI 理解论文
溯源树
样例
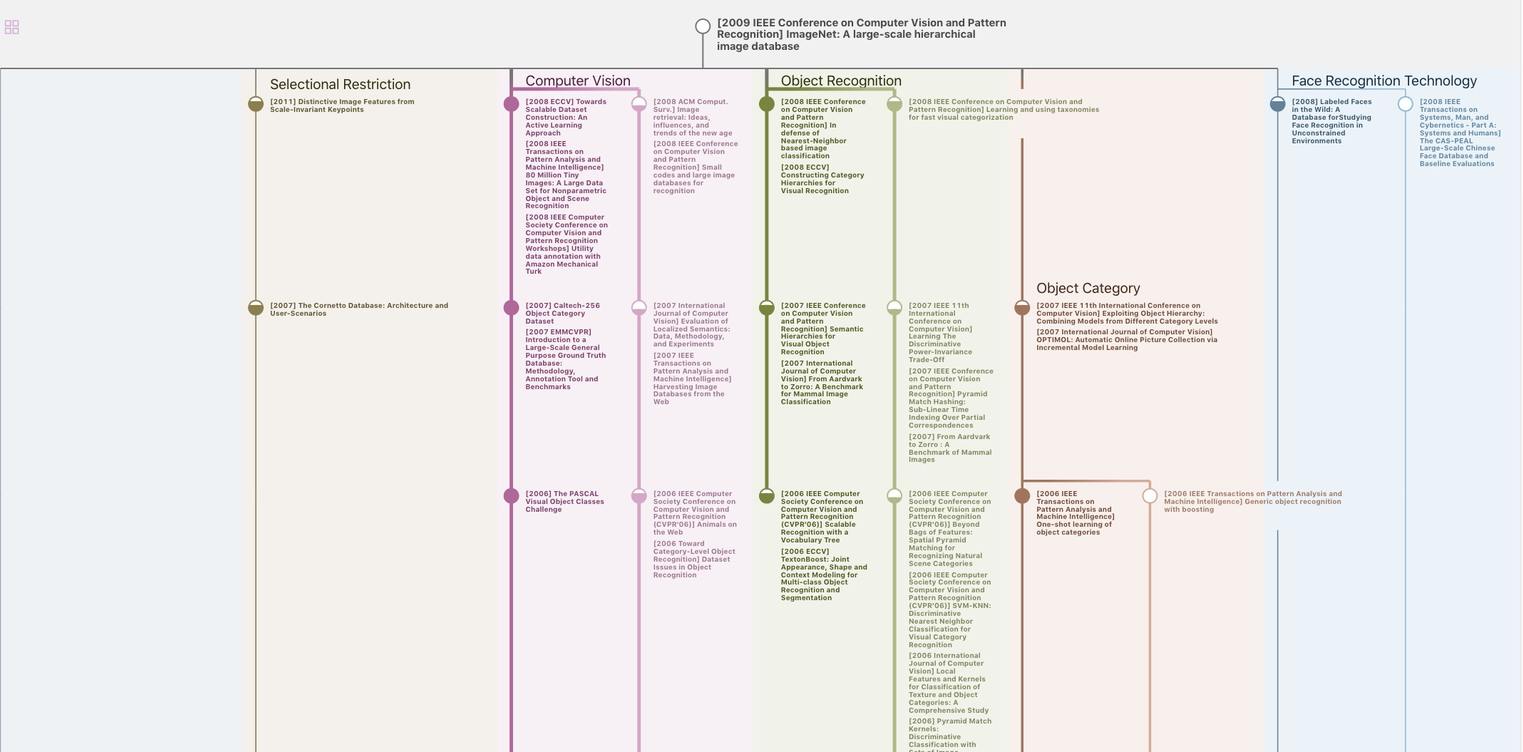
生成溯源树,研究论文发展脉络
Chat Paper
正在生成论文摘要