Semantic Prompt Based Multi-Scale Transformer for Few-Shot Classification.
2023 IEEE International Conference on Big Data (BigData)(2023)
摘要
Few-shot learning (FSL) is a machine learning method aimed at learning problem-solving models from a small number of samples. Few-shot classification is a task that utilizes few-shot learning for image classification. However, the existing models have poor classification performance when dealing with datasets with large differences in target scale distribution. In view of the large difference in the scale distribution of the sample target in the few-shot learning dataset, this paper proposes a novel few-shot classification model based on multi-scale Vision Transformer using semantic prompt. This model uses the Transformer block which has more generalization ability and long-range dependencies, and constructs a multi-scale feature fusion model on the large-scale cross-domain and cross-scale dataset Meta-Dataset which consists of 10 datasets, including natural image datasets, handwritten characters, and graffiti datasets. By using an explicit multi-scale feature fusion method, the model can obtain category features with cross scale information, thereby improving the robustness of the model to sample targets of different scales. In addition, the model improved its performance by learning additional semantic prompt, achieving a 2.43% improvement.
更多查看译文
关键词
few-shot classification,self-attention mechanism,muti-scale feature fusion,semantic prompt
AI 理解论文
溯源树
样例
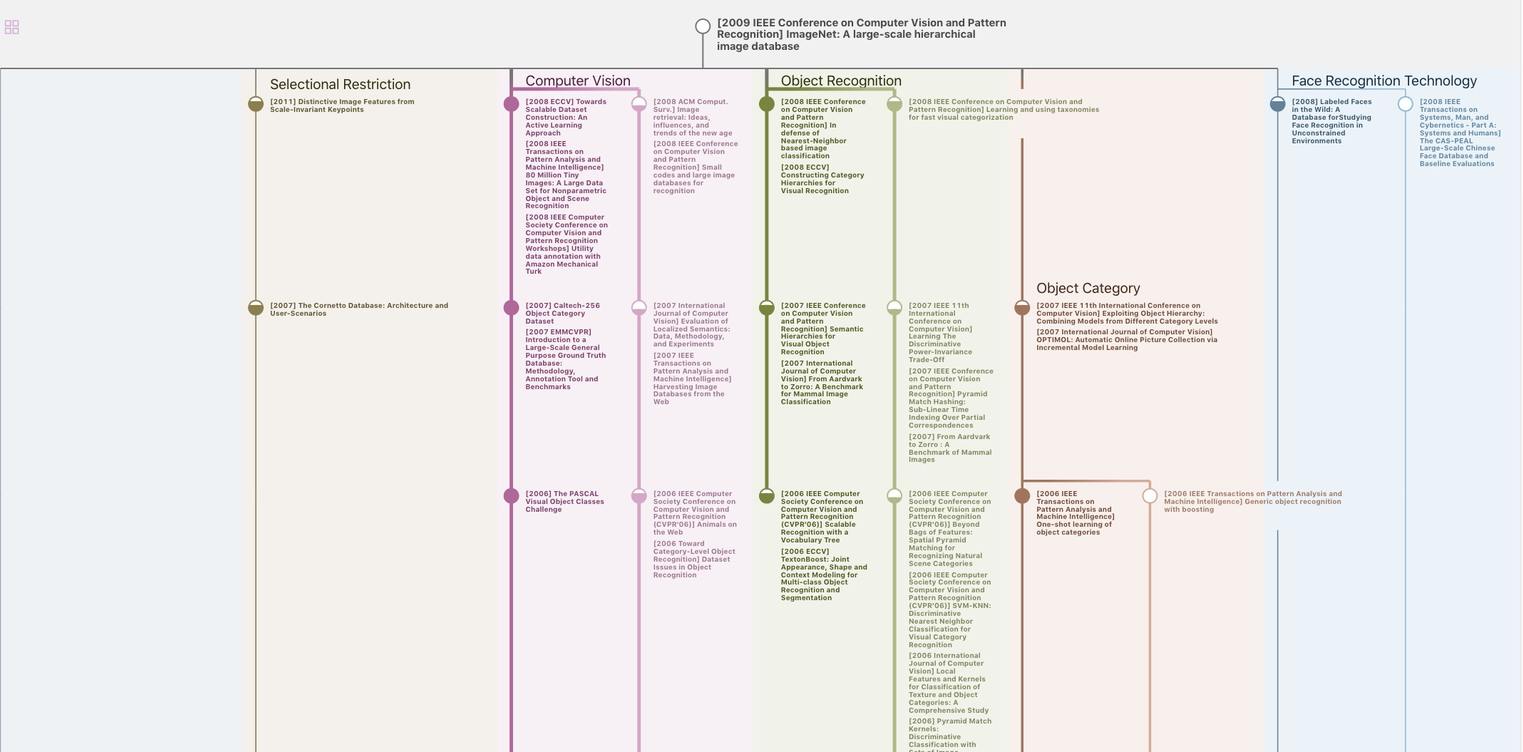
生成溯源树,研究论文发展脉络
Chat Paper
正在生成论文摘要