Classification of Cognitive-Aesthetic Responses Using Machine Learning and EEG Data.
2023 IEEE International Conference on Big Data (BigData)(2023)
摘要
Humans react both cognitively and emotionally to the built environment. With the recent advent of wearable technology such as mobile EEG devices and biometric wrist monitors that record the electrical activity of the brain and measure physiological data, we have new tools to inspect the internal states of building visitors and to determine whether a measurable, physiological response accompanies their experiences. These measures can shed light on the neural correlates of aesthetic architectural appreciation through specific electrographic signatures. They also create a promising foundation for future architectural design.In this study, we evaluated the cognitive impacts of two distinct architectural conditions—one secular and one religious—on individuals with committed religious faith. Employing machine learning algorithms, specifically the random forest and support vector machine, to analyze the EEG data, we constructed intrasubject and intersubject models to predict whether a given second of EEG data was recorded in the secular or religious building. The best intrasubject model demonstrates just under 80% accuracy on average, reaching up to 90% accuracy for some subjects, indicating a consistent and measurable response within each participant. However, the individual variability in EEG measurements poses challenges for constructing an intersubject model with a sample size of 32 subjects. To achieve success across a broader range of subjects, a larger sample size or additional features may be necessary for developing an effective classifier.
更多查看译文
关键词
Cognitive-Aesthetic Responses,Machine Learning,EEG,Random Forest
AI 理解论文
溯源树
样例
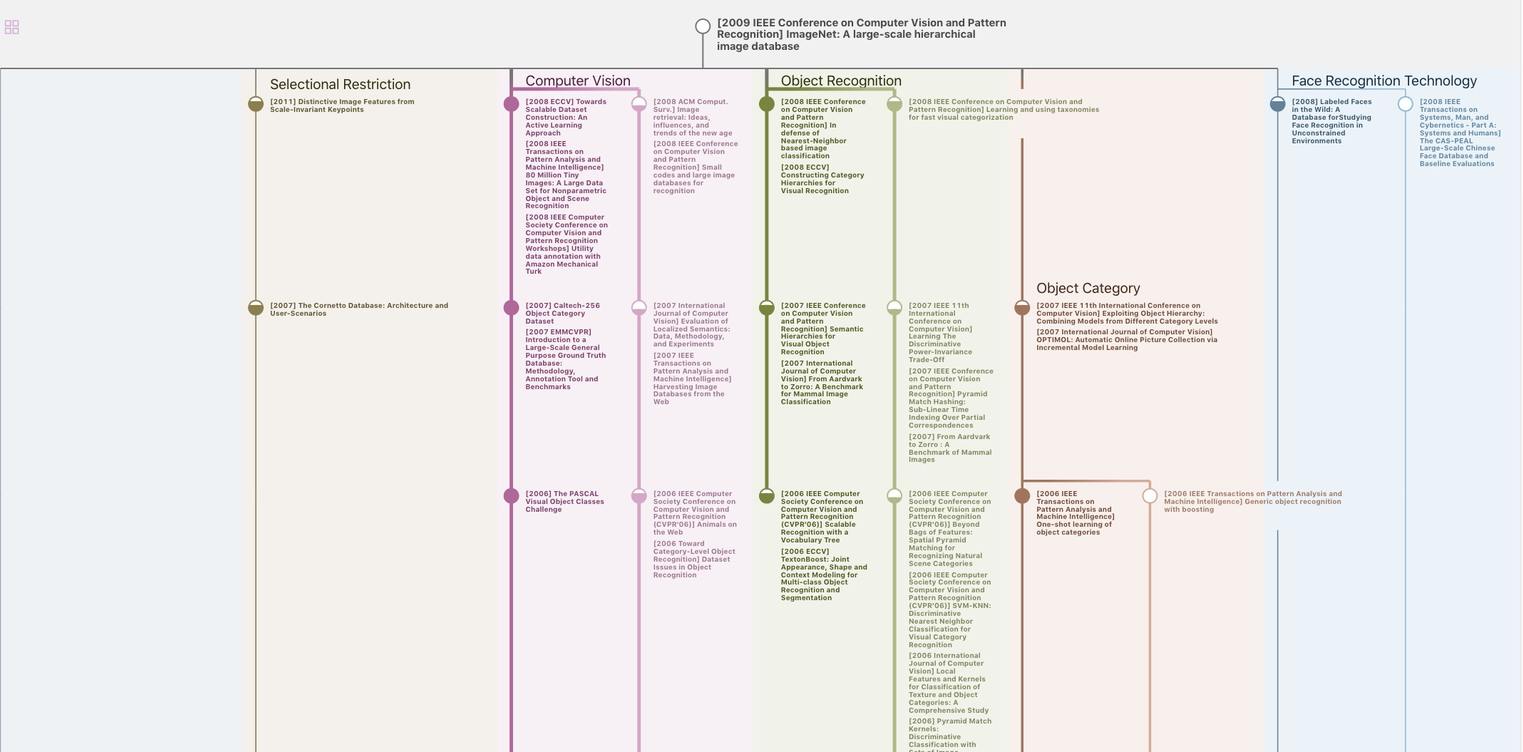
生成溯源树,研究论文发展脉络
Chat Paper
正在生成论文摘要