TRANSGNN: Towards Knowledge Enhanced Top-K Recommendation via Transformed Heterogeneous Graph Neural Network.
2023 IEEE International Conference on Big Data (BigData)(2023)
Abstract
The knowledge graph (KG) enhanced recommendation system has shown superior performance and attracted considerable attention. In recent years, researchers have attempted to apply graph neural networks (GNNs) and build end-to-end knowledge graph enhanced recommendation systems. Although several methods are proposed, it is still challenging to apply GNNs to solve the problem of KG enhanced recommendation due to the heterogeneous, redundant, and large-scale nature of KG versus the homogeneous nature of GNNs. To address the limitations of existing techniques, we propose a transformed heterogeneous graph neural network (TRANSGNN), an item-centric and GNN-powered framework that can better explore the heterogeneous, redundant, and large-scale relations among items in KG for an effective and efficient KG enhanced top- K recommendation. In particular, we propose an item-centric graph transformation module to transform the heterogeneous KG into a homogeneous item-item graph. This scheme allows us to distill the knowledge from the KG and encode the heterogeneous, redundant, and large-scale item-item relationship into a smaller, compact, homogeneous graph, better fitting the GNNs architecture that prefers homogeneous and small-scale graph input. We further introduce a collaborative graph fusion module to fuse the item-wise KG relational signals and the collaborative signals by augmenting the collaborative graph with the transformed item-item graph. Next, we propose to use a graph collaborative encoder over the augmented graph to propagate information along with high-order connectivity. The proposed framework is general and can be easily extended with advanced KG embedding and GNN models. Extensive experiments on three public datasets demonstrate the effectiveness of the proposed approach in significantly (up to 23%) improving the KG enhanced recommendation performance over the state-of-the-art methods, such as KGIN, KGAT, KGNN-LS in both regular and cold-start settings. The proposed approach is also efficient with significantly (up to 31%) fewer parameters.
MoreTranslated text
Key words
Heterogeneous Network,Graph Neural Networks,Extensive Experiments,Recommender Systems,Regular Set,Neural Network Framework,Graph Neural Network Model,Considerable Interest,Types Of Relationships,Weight Decay,Sparse Data,User Behavior,Latent Space,Pairwise Similarity,User Preferences,Similar Items,Heterogeneous Information,Collaborative Filtering,Graph Of Order,User Representation,Recommendation Task,Commonsense Knowledge,Multiple Types Of Information
AI Read Science
Must-Reading Tree
Example
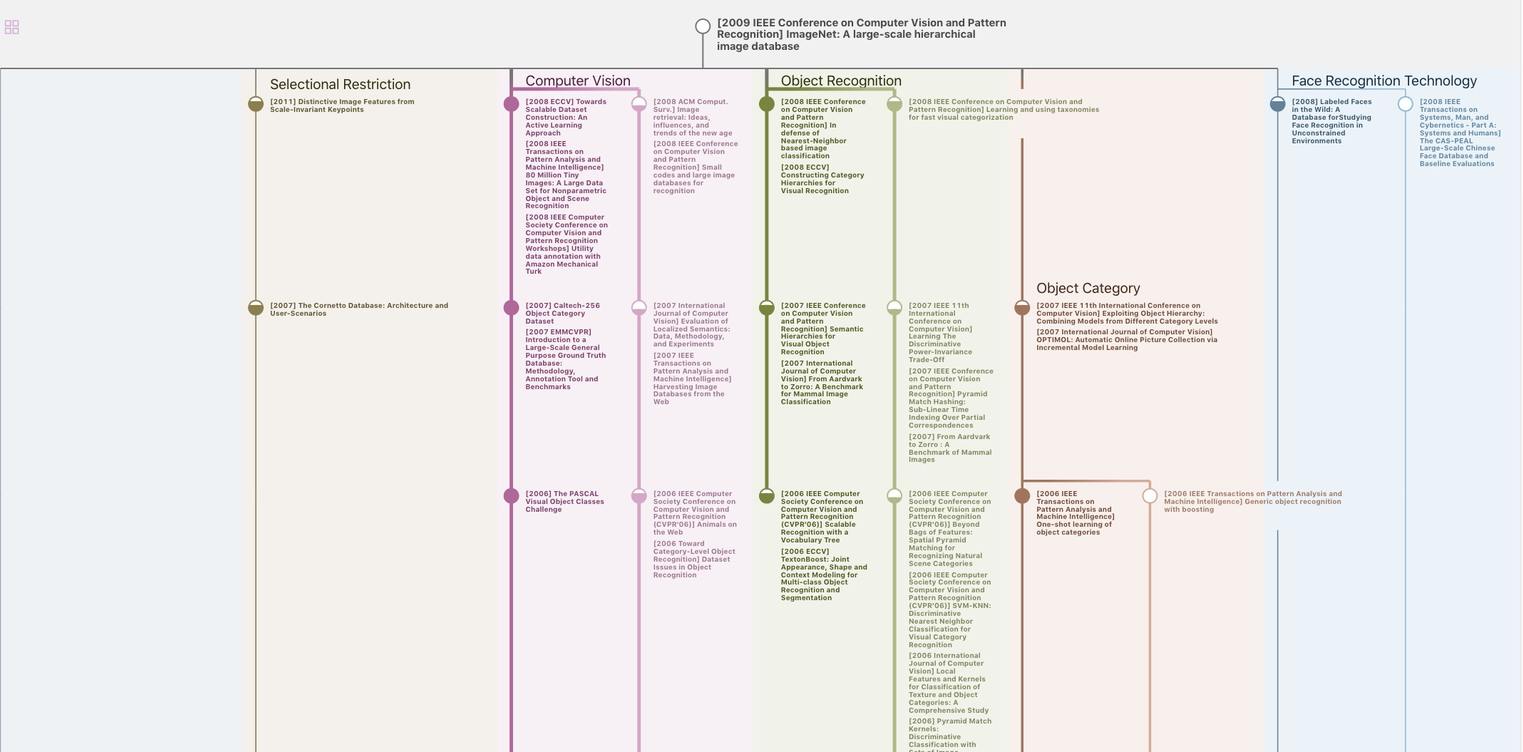
Generate MRT to find the research sequence of this paper
Chat Paper
Summary is being generated by the instructions you defined