A scalable model for on-chip spiral inductors by Space-mapping neural network
2023 IEEE International Symposium On Antennas And Propagation (ISAP)(2023)
摘要
This paper presents a scalable model for on-chip spiral inductors utilizing the space-mapping neural network (SMNN), built upon an enhanced 1-π equivalent circuit model. To address the inductor drop observed at low frequencies, a series network consisting of two pairs of inductors and resistors is integrated into the 1-π equivalent circuit model. The topology of the SMNN is optimized using the global search capability of the particle swarm optimization (PSO) algorithm, which determines the optimal weights and thresholds for the SMNN. Compared with traditional neural network models, the proposed SMNN reduces the number of neurons required for mapping the input-output nonlinear relationship, leading to enhanced accuracy and reduced modeling time, which takes a mere 10 to 15 seconds.
更多查看译文
关键词
spiral inductors,space-mapping,neural network,parametric model,Particle Swarm Optimization (PSO),scalable models
AI 理解论文
溯源树
样例
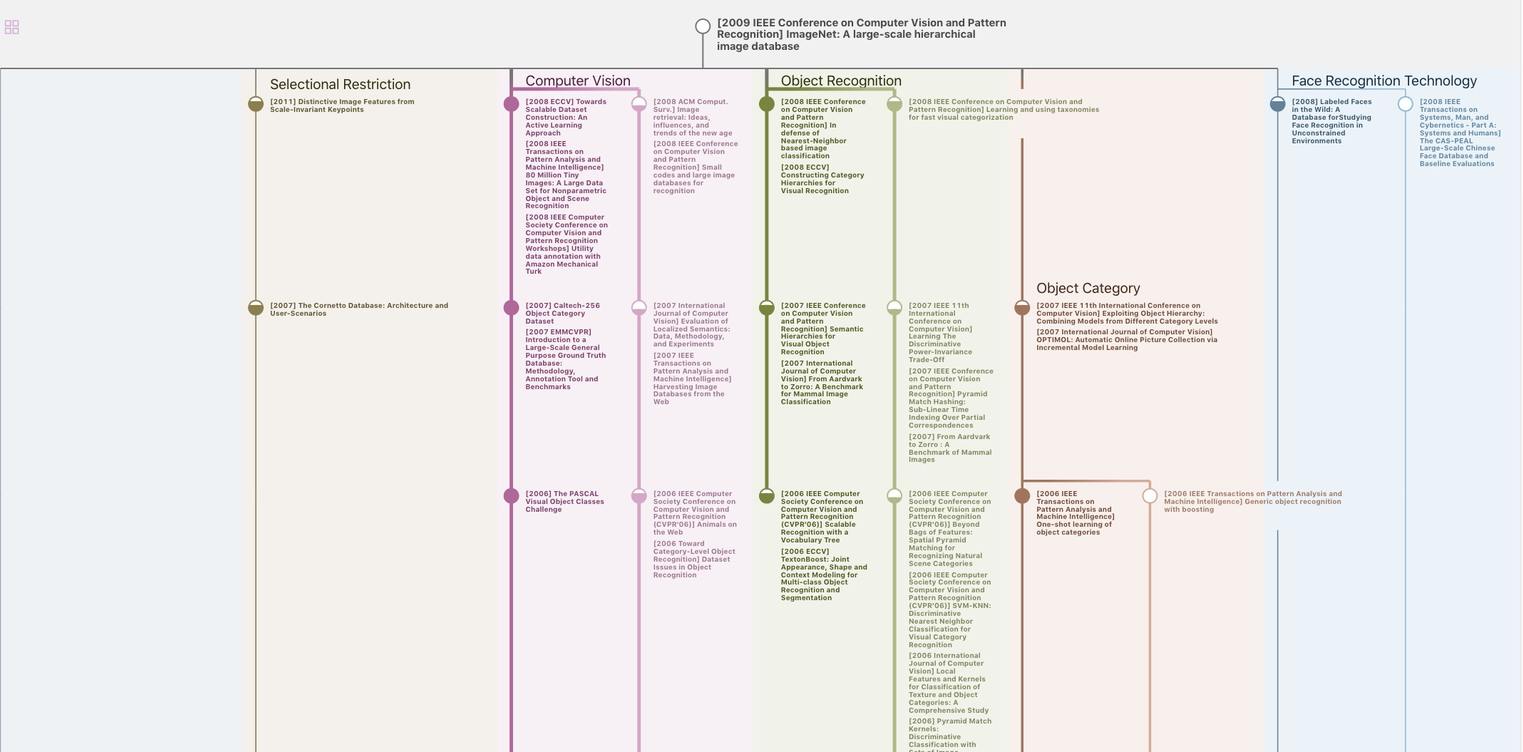
生成溯源树,研究论文发展脉络
Chat Paper
正在生成论文摘要