Discovering Consensus Regions for Interpretable Identification of RNA N6-Methyladenosine Modification Sites via Graph Contrastive Clustering.
IEEE journal of biomedical and health informatics(2024)
摘要
As a pivotal post-transcriptional modification of RNA, N6-methyladenosine (m6A) has a substantial influence on gene expression modulation and cellular fate determination. Although a variety of computational models have been developed to accurately identify potential m6A modification sites, few of them are capable of interpreting the identification process with insights gained from consensus knowledge. To overcome this problem, we propose a deep learning model, namely M6A-DCR, by discovering consensus regions for interpretable identification of m6A modification sites. In particular, M6A-DCR first constructs an instance graph for each RNA sequence by integrating specific positions and types of nucleotides. The discovery of consensus regions is then formulated as a graph clustering problem in light of aggregating all instance graphs. After that, M6A-DCR adopts a motif-aware graph reconstruction optimization process to learn high-quality embeddings of input RNA sequences, thus achieving the identification of m6A modification sites in an end-to-end manner. Experimental results demonstrate the superior performance of M6A-DCR by comparing it with several state-of-the-art identification models. The consideration of consensus regions empowers our model to make interpretable predictions at the motif level. The analysis of cross validation through different species and tissues further verifies the consistency between the identification results of M6A-DCR and the evolutionary relationships among species.
更多查看译文
关键词
RNA sequence,m6A modification site,interpretable end-to-end predictor,consensus region,evolutionary relationships
AI 理解论文
溯源树
样例
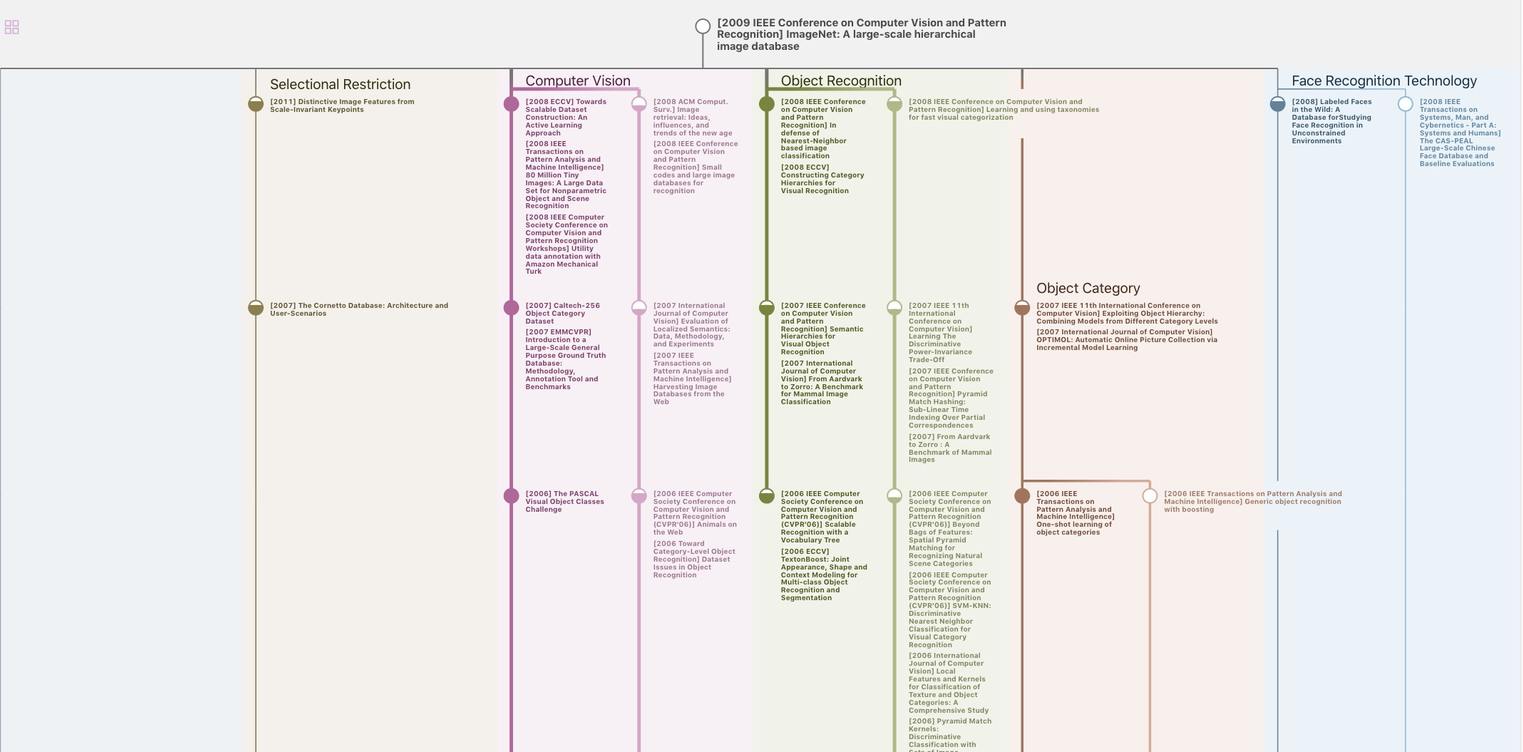
生成溯源树,研究论文发展脉络
Chat Paper
正在生成论文摘要