LoGo-GR: A Local to Global Graphical Reasoning Framework for Extracting Structured Information From Biomedical Literature.
IEEE journal of biomedical and health informatics(2024)
Abstract
In the biomedical literature, entities are often distributed within multiple sentences and exhibit complex interactions. As the volume of literature has increased dramatically, it has become impractical to manually extract and maintain biomedical knowledge, which would entail enormous costs. Fortunately, document-level relation extraction can capture associations between entities from complex text, helping researchers efficiently mine structured knowledge from the vast medical literature. However, how to effectively synthesize rich global information from context and accurately capture local dependencies between entities is still a great challenge. In this paper, we propose a Local to Global Graphical Reasoning framework (LoGo-GR) based on a novel Biased Graph Attention mechanism (B-GAT). It learns global context feature and information of local relation path dependencies from mention-level interaction graph and entity-level path graph respectively, and collaborates with global and local reasoning to capture complex interactions between entities from document-level text. In particular, B-GAT integrates structural dependencies into the standard graph attention mechanism (GAT) as attention biases to adaptively guide information aggregation in graphical reasoning. We evaluate our method on three publicly biomedical document-level datasets: Drug-Mutation Interaction (DV), Chemical-induced Disease (CDR), and Gene-Disease Association (GDA). LoGo-GR has advanced and stable performance compared to other state-of-the-art methods (it achieves state-of-the-art performance with 96.14%-97.39% F1 on DV dataset, advanced performance with 68.89% F1 and 84.22% F1 on CDR and GDA datasets, respectively). In addition, LoGo-GR also shows advanced performance on general-domain document-level relation extraction dataset, DocRED, which proves that it is an effective and robust document-level relation extraction framework.
MoreTranslated text
Key words
biomedical information mining,document-level relation extraction,graphical reasoning,graph attention mechanism
AI Read Science
Must-Reading Tree
Example
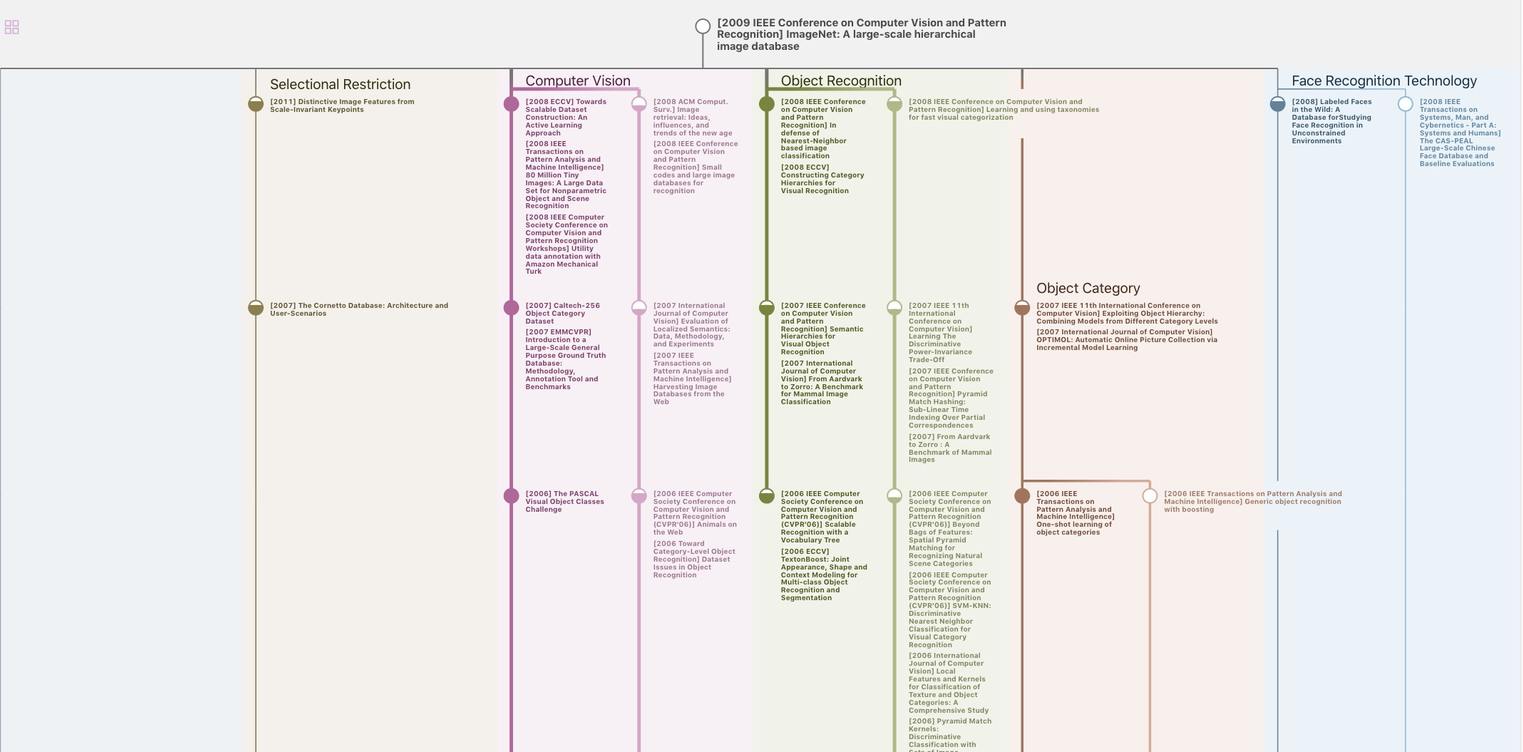
Generate MRT to find the research sequence of this paper
Chat Paper
Summary is being generated by the instructions you defined