LAFED: Towards robust ensemble models via Latent Feature Diversification
Pattern Recognition(2024)
Abstract
Adversarial examples pose a significant challenge to the security of deep neural networks (DNNs). In order to defend against malicious attacks, adversarial training forces DNNs to learn more robust features by suppressing generalizable but non -robust features, which boosts the robustness while suffering from significant accuracy drops on clean images. Ensemble training, on the other hand, trains multiple sub -models to predict data for improved robustness and still achieves desirable accuracy on clean data. Despite these efforts, previous ensemble methods are still susceptible to attacks and fail to increase model diversity as the size of the ensemble group increases. In this work, we revisit the model diversity from the perspective of data and discover that high similarity between training batches decreases feature diversity and weakens ensemble robustness. To this end, we propose Latent Feature Diversification (LAFED), which reconstructs training sets with diverse features during the optimization, enhancing the overall robustness of an ensemble. For each sub -model, LAFED treats the vulnerability extracted from other sub -models as raw data, which is then combined with round -changed weights with a stochastic manner in the latent space. This results in the formation of new features, remarkably reducing the similarity of learned representations between the submodels. Furthermore, LAFED enhances feature diversity within the ensemble model by utilizing hierarchical smoothed labels. Extensive experiments illustrate that LAFED significantly improves diversity among submodels and enhances robustness against adversarial attacks compared to current methods. The code is publicly available at https://github.com/zhuangwz/LAFED.
MoreTranslated text
Key words
Adversarial example,Adversarial defense,Ensemble model,Robustness
AI Read Science
Must-Reading Tree
Example
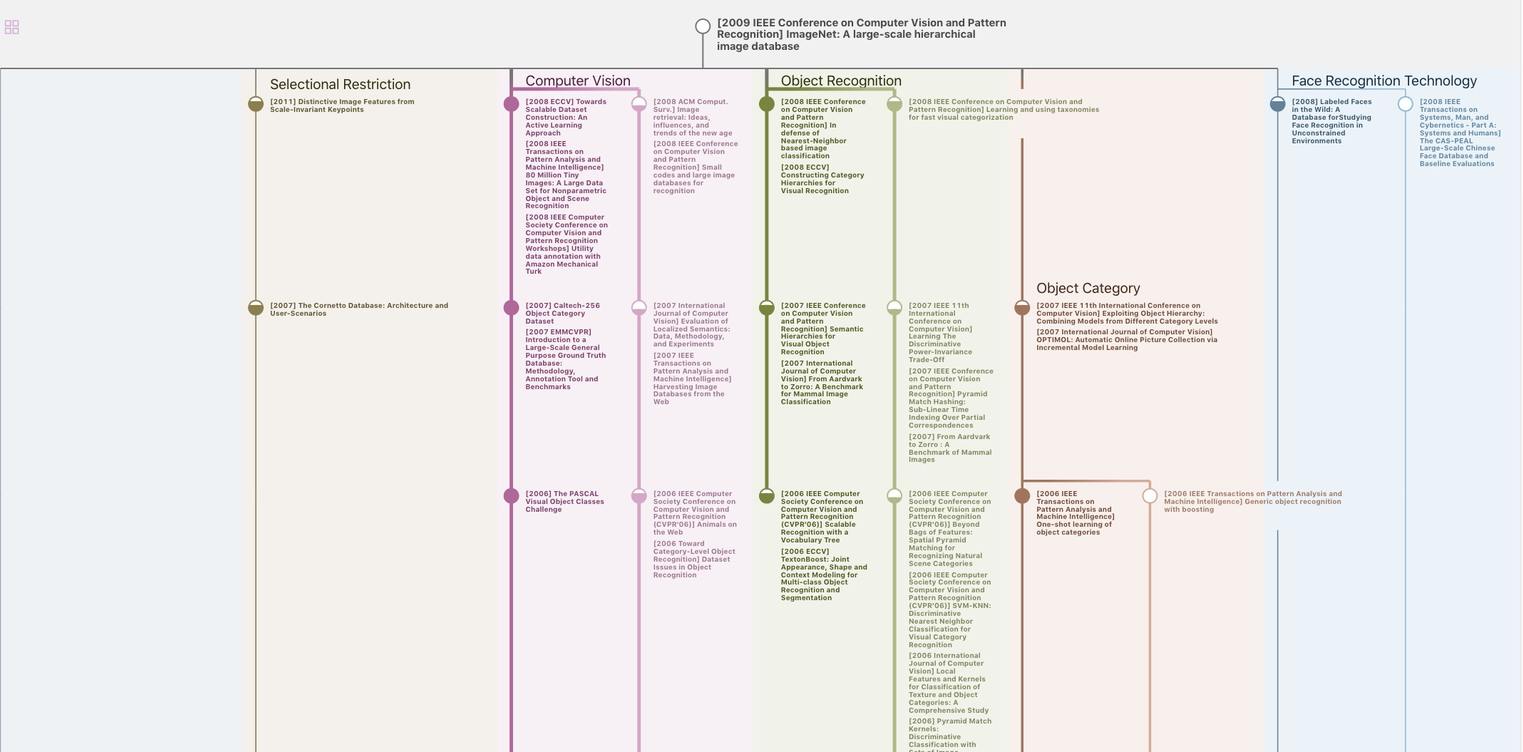
Generate MRT to find the research sequence of this paper
Chat Paper
Summary is being generated by the instructions you defined