Multi-source domain generalization peron re-identification with knowledge accumulation and distribution enhancement
Applied Intelligence(2024)
摘要
Domain generalization person re-identification (re-ID) is a more realistic task that aims to learn a model with multiple labeled source domains and generalize its inference to an unseen domain. However, under joint training of multi-source domains, some difficulties such as domain imbalance, domain shifts and small training batches not only influence the representation learning of the model but also lead to poor generalization performance. In this work, we enhance the generalization performance by proposing two strategies including knowledge accumulation and distribution enhancement for multi-source domain generalization person re-ID. Specifically, we encourage the learning of semantically significant features globally by establishing a simple but effective knowledge accumulation feature classifier (KAFC). In which, continuous learning guided by the label information explicitly provides stable prototype matching for each source domain, directly solving the problem that unstable parameter updates due to the small-batch data and source domain shifts. Secondly, to enhance the generalization ability and learn more robust representations, a multi-mix batch normalization (MMBN) module is introduced to generate the mixture features with cross-domain distribution information, where an approach based on the exponential moving average is adopted for capturing the domain distribution knowledge. Extensive experiments on multiple widely-used benchmarks demonstrate that our method can effectively improve the generalization capability on unseen domains.
更多查看译文
关键词
Person re-identification,Multi-Source Domain generalization,Continual Learning,Data Augmentation
AI 理解论文
溯源树
样例
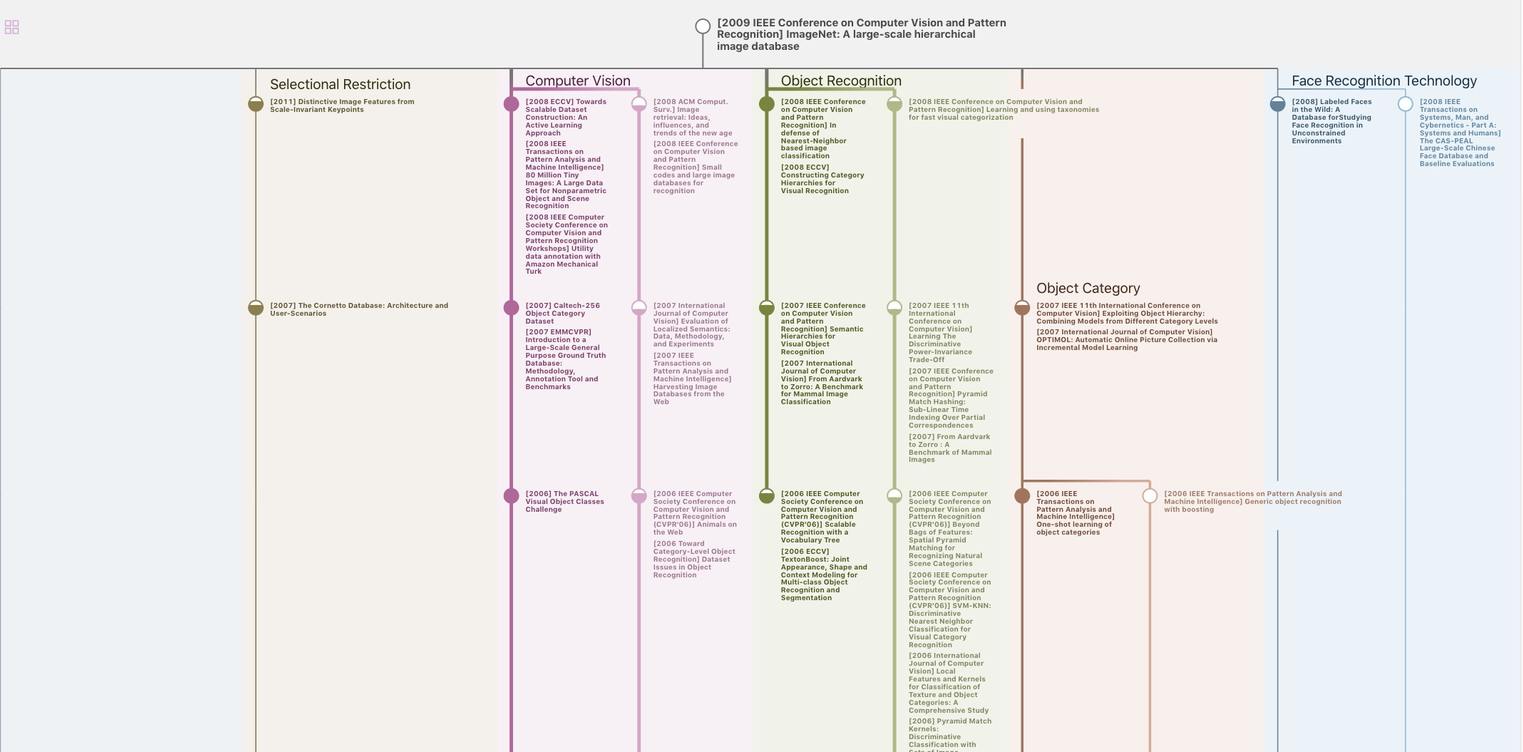
生成溯源树,研究论文发展脉络
Chat Paper
正在生成论文摘要