The Effect of Human v/s Synthetic Test Data and Round-tripping on Assessment of Sentiment Analysis Systems for Bias
2023 5th IEEE International Conference on Trust, Privacy and Security in Intelligent Systems and Applications (TPS-ISA)(2024)
摘要
Sentiment Analysis Systems (SASs) are data-driven Artificial Intelligence
(AI) systems that output polarity and emotional intensity when given a piece of
text as input. Like other AIs, SASs are also known to have unstable behavior
when subjected to changes in data which can make it problematic to trust out of
concerns like bias when AI works with humans and data has protected attributes
like gender, race, and age. Recently, an approach was introduced to assess SASs
in a blackbox setting without training data or code, and rating them for bias
using synthetic English data. We augment it by introducing two human-generated
chatbot datasets and also consider a round-trip setting of translating the data
from one language to the same through an intermediate language. We find that
these settings show SASs performance in a more realistic light. Specifically,
we find that rating SASs on the chatbot data showed more bias compared to the
synthetic data, and round-tripping using Spanish and Danish as intermediate
languages reduces the bias (up to 68
in synthetic data, it takes a surprising turn by increasing the bias! Our
findings will help researchers and practitioners refine their SAS testing
strategies and foster trust as SASs are considered part of more
mission-critical applications for global use.
更多查看译文
关键词
bias,round-trip translation,causal models
AI 理解论文
溯源树
样例
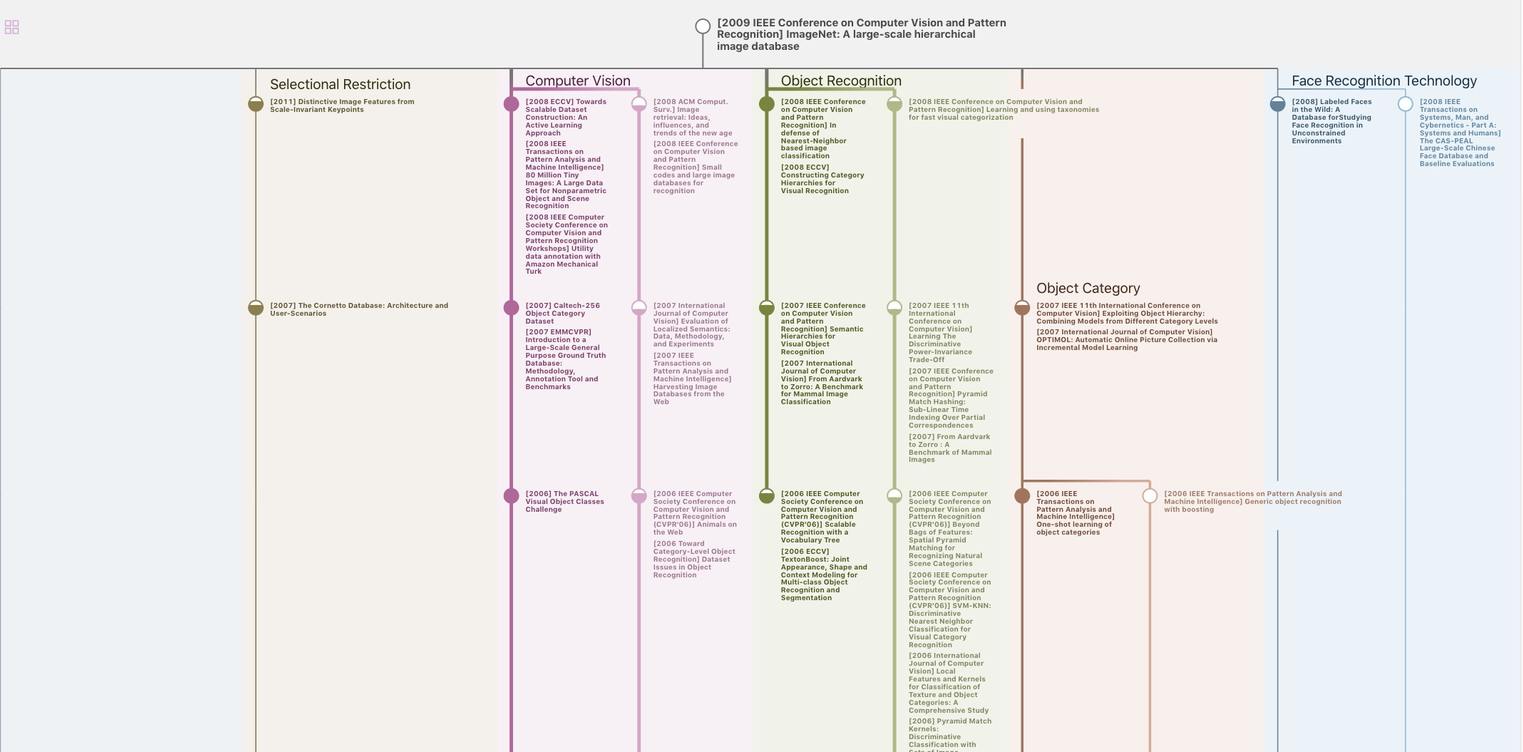
生成溯源树,研究论文发展脉络
Chat Paper
正在生成论文摘要