Deep Learning Based Adaptive Joint mmWave Beam Alignment
CoRR(2024)
摘要
The challenging propagation environment, combined with the hardware
limitations of mmWave systems, gives rise to the need for accurate initial
access beam alignment strategies with low latency and high achievable
beamforming gain. Much of the recent work in this area either focuses on
one-sided beam alignment, or, joint beam alignment methods where both sides of
the link perform a sequence of fixed channel probing steps. Codebook-based
non-adaptive beam alignment schemes have the potential to allow multiple user
equipment (UE) to perform initial access beam alignment in parallel whereas
adaptive schemes are favourable in achievable beamforming gain. This work
introduces a novel deep learning based joint beam alignment scheme that aims to
combine the benefits of adaptive, codebook-free beam alignment at the UE side
with the advantages of a codebook-sweep based scheme at the base station. The
proposed end-to-end trainable scheme is compatible with current cellular
standard signaling and can be readily integrated into the standard without
requiring significant changes to it. Extensive simulations demonstrate superior
performance of the proposed approach over purely codebook-based ones.
更多查看译文
AI 理解论文
溯源树
样例
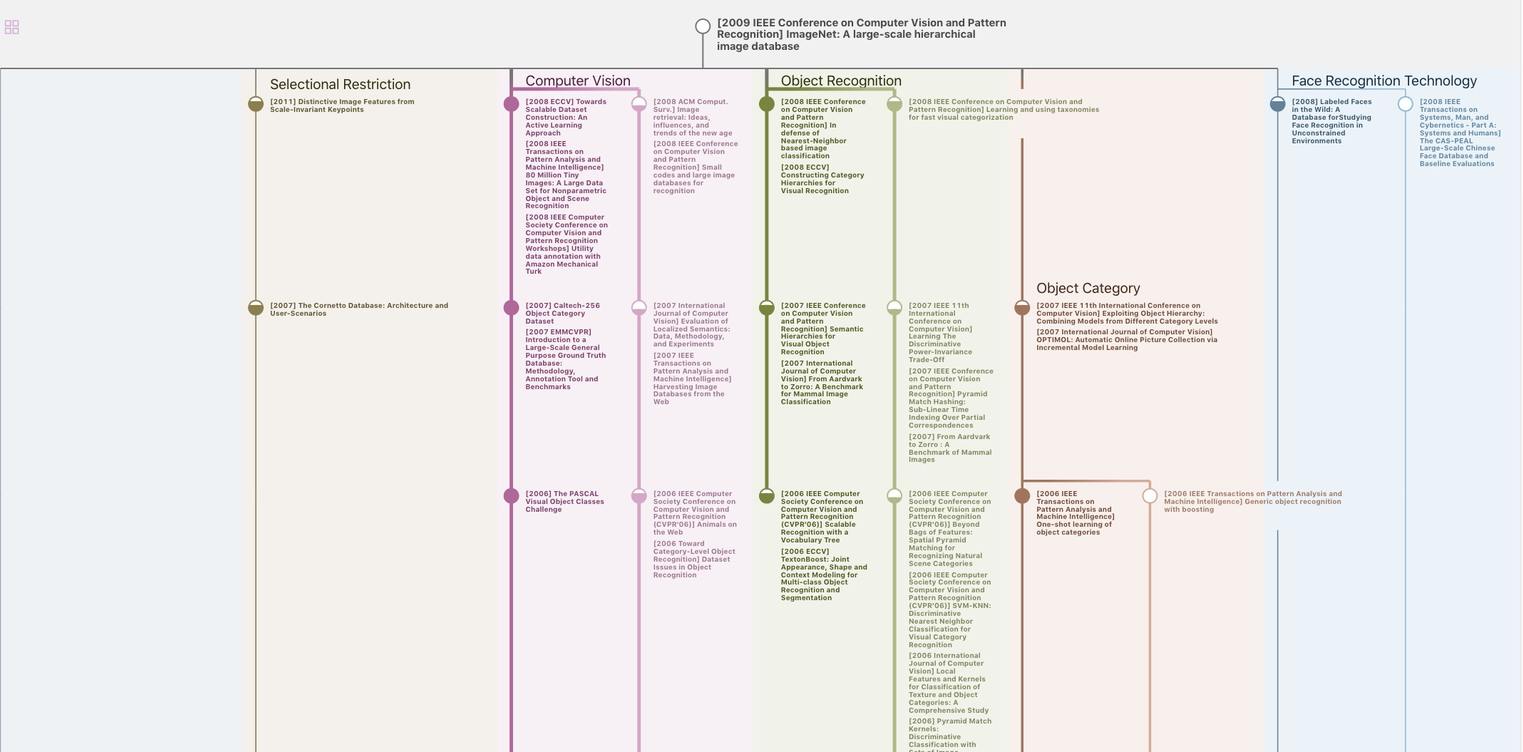
生成溯源树,研究论文发展脉络
Chat Paper
正在生成论文摘要