Fusion of Spectral and Connectivity Features to Detect Depressive Disorder using EEG Signals
2023 IEEE 3rd Applied Signal Processing Conference (ASPCON)(2023)
摘要
Depressive disorder (DD) has a high morbidity and death rate, contributing to suicide, the incidence and unfavorable effects of medical disease, and drug addiction. DD necessitates long-term monitoring, changes in symptom presentations, and subjective evaluation, which opens the door to analyze brain signals using modern neuro-signal processing and machine learning techniques. To detect depression in an automatic and noninvasive fashion, we have chosen electroencephalogram (EEG) signals from 199 DD adults and 95 healthy control (HC) adults. We have used a machine learning classification framework which uses spectral and functional connectivity features and with their feature-level fusion. Compared to the individual features, the fusion-based feature outperforms (98.06 ± 1.19 % accuracy) at a significant p-value (<0.05) for distinguishing DD from HC. Artificial neural network (ANN) performs better than different kernel-based support vector machines (SVM). In frequency band-wise analysis, using our fusion method, the delta frequency band (1-4 Hz) demonstrates the most discriminant features. Using connectivity networks and their strength, our findings reveal that the left posterior and right anterior brain regions are the most linked in depression. The improved performance signifies the clinical relevance of the work providing reliable assistance for depression detection and has the potential to find fusion-based connectivity patterns of depression.
更多查看译文
关键词
Depressive Disorder,Electroencephalography,Machine Learning,Power Spectral Density,Coherence,Feature-level Fusion,Classification
AI 理解论文
溯源树
样例
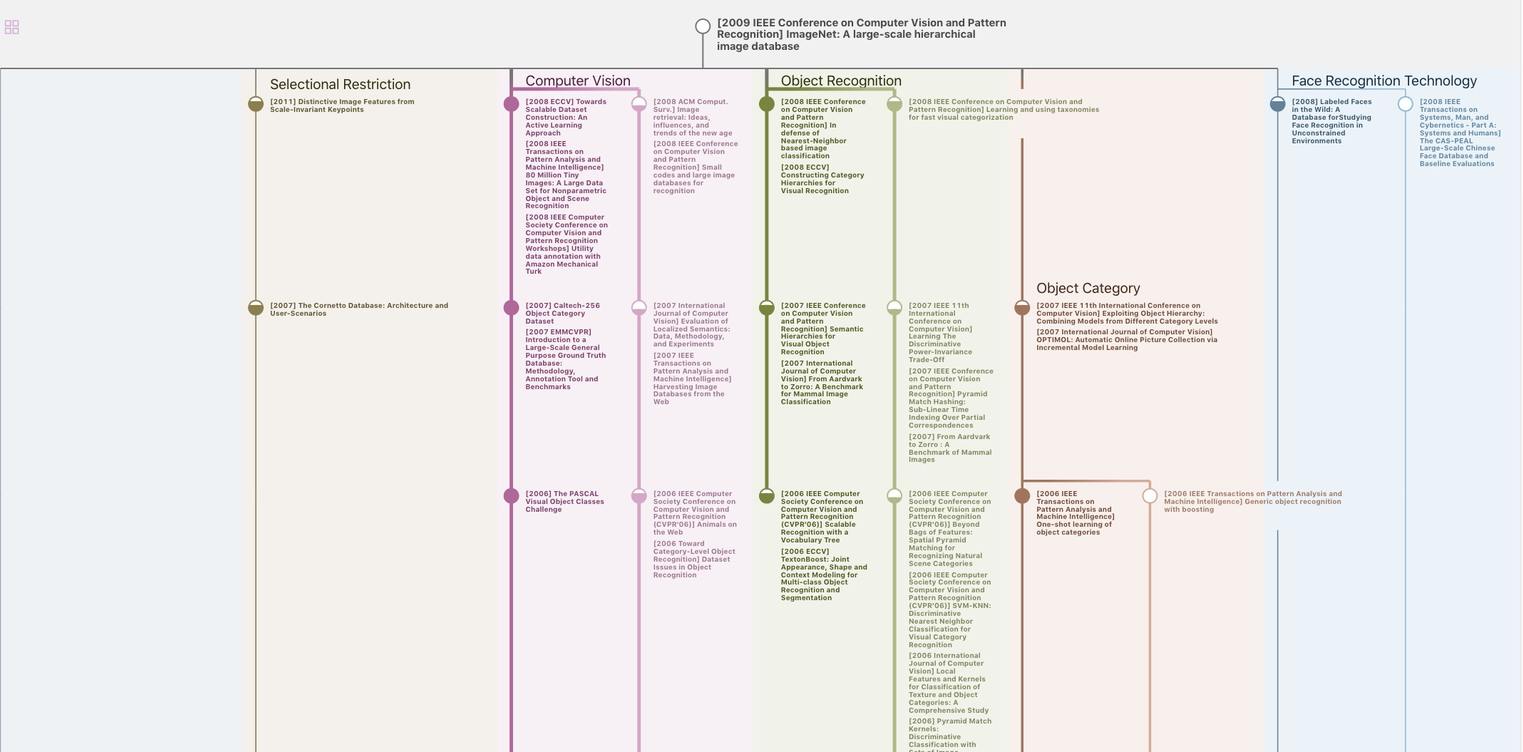
生成溯源树,研究论文发展脉络
Chat Paper
正在生成论文摘要