Community-aware explanations in knowledge graphs with XP-GNN
biorxiv(2024)
Abstract
Machine learning applications for the drug discovery pipeline have exponentially increased in the last few years. An example of these applications is the biological Knowledge Graph. These graphs represent biological entities and the relations between them based on existing knowledge. Graph machine learning models such as Graph Neural Networks can be applied on top of knowledge graphs to support the development of novel therapeutics. Nevertheless, Graph Neural Networks present an improved performance at the expense of complexity, becoming difficult to explain their decisions. State-of-the-art explanation algorithms for Graph Neural Networks focus on determining the most relevant subgraphs involved in their decision-making while considering graph elements (nodes and edges) as independent entities and ignoring any communities these graphs could present. We explore in this work the idea that graph community structure in biological Knowledge Graphs could provide a better grasp of the decision-making of Graph Neural Networks. For that purpose, we introduce XP-GNN , a novel explanation technique for Graph Neural Networks in Knowledge Graphs. XP-GNN exploits the communities of nodes or edges in graphs to refine their explanations, inspired by cooperative game theory . We characterize XP-GNN in a basic example and in terms of scalability and stability. In two relevant use cases for the drug discovery pipeline, XP-GNN provides more relevant explanations than previous techniques, being evaluated quantitatively and by domain experts. At the same time, XP-GNN presents limitations on scalability and stability, which we will address.
ACM Reference Format Andrés Martínez Mora, Dimitris Polychronopoulos, Michaël Ughetto, and Sebastian Nilsson. 2024. Community-aware explanations in knowledge graphs with XP-GNN. In Proceedings of ACM Conference (Conference’17) . ACM, New York, NY, USA, 21 pages.
This work has been funded by AstraZeneca AB, Mölndal, Sweden and AstraZeneca Cambridge. Unfortunately, due to proprietary reasons from AstraZeneca AB, the data used in this work cannot be shared .
### Competing Interest Statement
A.M.M and D.P were employees and shareholders of AstraZeneca in the past. S.N. and M.U. are currently employed by AstraZeneca, and also hold shares.
MoreTranslated text
AI Read Science
Must-Reading Tree
Example
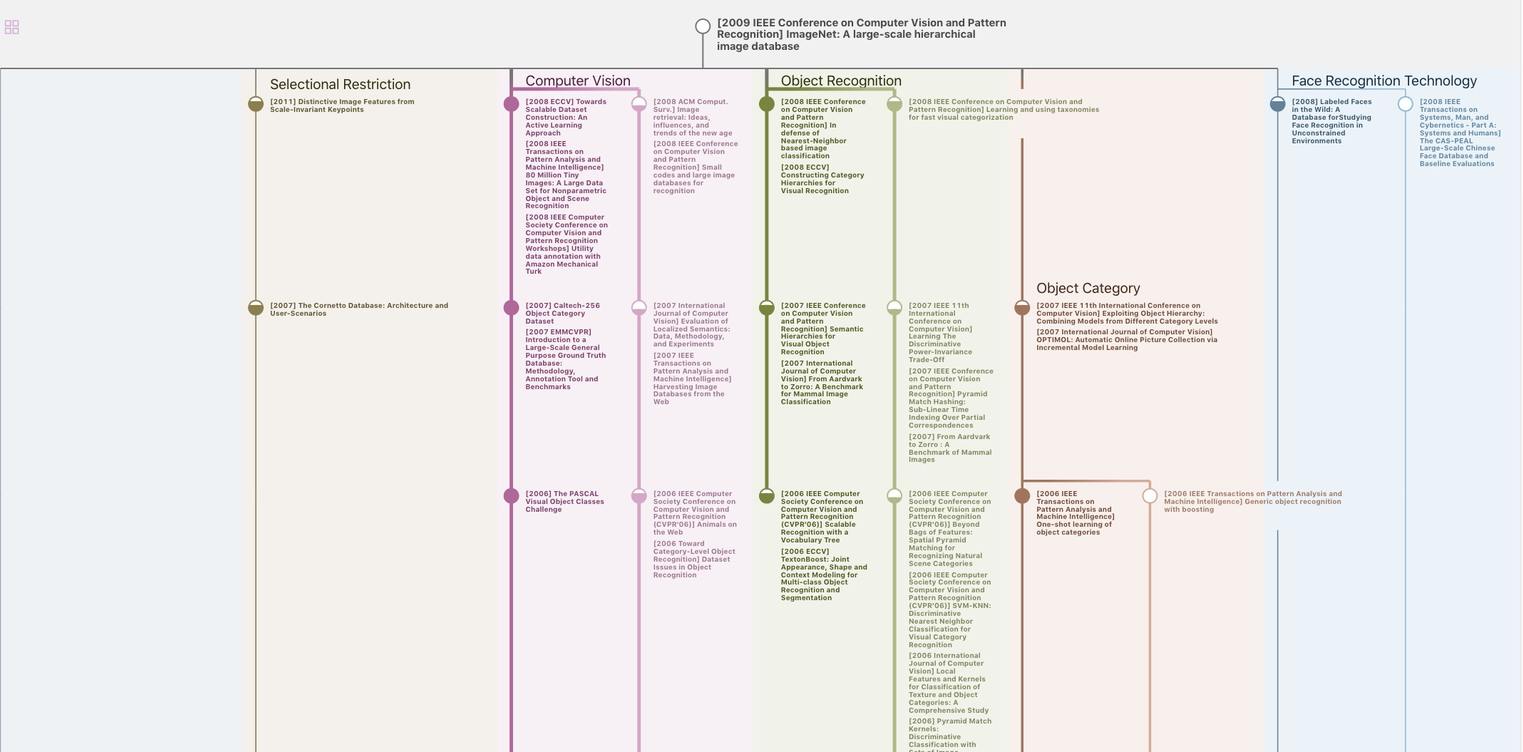
Generate MRT to find the research sequence of this paper
Chat Paper
Summary is being generated by the instructions you defined