Feature similarity learning based on fuzziness minimization for semi-supervised medical image segmentation
INFORMATION FUSION(2024)
摘要
Deep learning has advanced the automation and intelligence levels of medical image segmentation, but the acquisition of annotations for medical images proves to be very challenging. Deep semi -supervised learning provides an effective approach to tackling this challenge. In this paper, we propose a method of feature similarity learning for deep semi -supervised image segmentation. The method performs a weighted transformation on the latent features of deep convolution network, and the objective of this transformation is designed to minimize the uncertainty of feature similarity. Based on fuzzy sets of similarity, we define the fuzziness to measure the uncertainty in the objective, and demonstrate that the optimization objective is equivalent to strengthening the discriminant property of those transformed features. This similarity learning is independent of the pseudo -labels, boosting the robustness against noisy pseudo -labels. Our method is implemented through a plug -and -play neural network layer, which can be optimized alongside the segmentation model in an end -toend manner. The model validation focuses on the left atrium segmentation which is a challenging task for atrial fibrillation treatment. Comprehensive experiments demonstrate the effectiveness of the uncertainty -guided similarity learning on semi -supervised segmentation tasks.
更多查看译文
关键词
Fuzziness,Deep learning,Semi-supervised learning,Medical image segmentation,Left atrium MRI
AI 理解论文
溯源树
样例
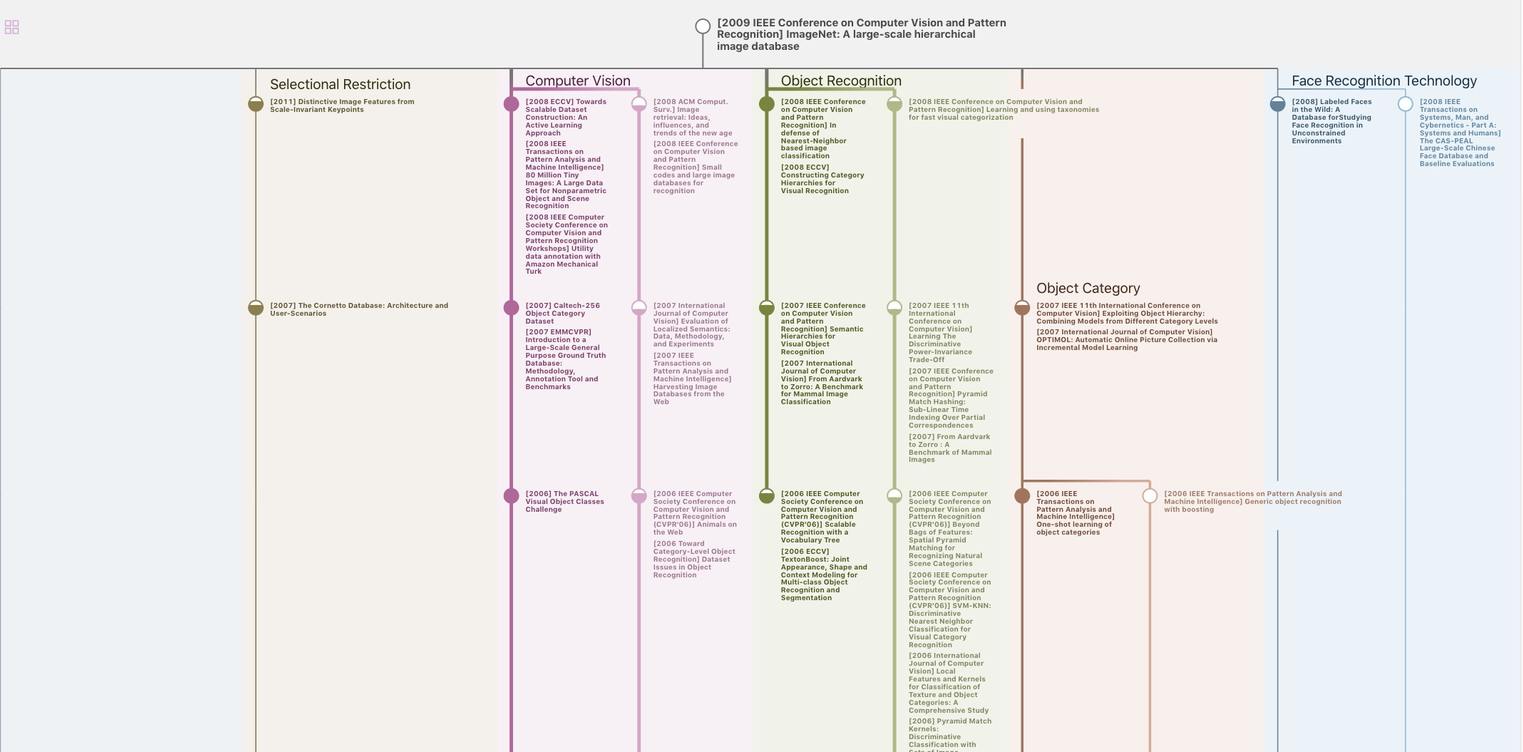
生成溯源树,研究论文发展脉络
Chat Paper
正在生成论文摘要