EPS$^{2}$ : Privacy Preserving Set-Valued Data Analysis in the Shuffle Model
IEEE Transactions on Knowledge and Data Engineering(2023)
Abstract
Collecting and analyzing users' set-valued data with privacy-preserving is a common scenario in real life. However, the existing solutions in LDP are not efficient enough, where users perturbing their data locally introduces a large amount of noise. The shuffle model, which adds a shuffler in LDP to shuffle all perturbed values, can amplify privacy, then improve utility. Inspired by this, we study the frequency estimation and top-
$k$
frequent item estimation of set-valued data in the shuffle model. To solve the challenges of different item quantities of users and further improve the utility, we combine sampling and shuffling together, and propose the
Encoding, Padding, Sampling, and Shuffling
framework, i.e., EPS
$^{2}$
. Based on this framework, we propose three protocols for frequency estimation in different application scenarios, then assemble them into multi-phase protocols for the top-
$k$
frequent item estimation. Theoretically, we identify all three protocols gain dual privacy amplification from sampling and shuffling. And by setting the size of users' set to 1, we can extend this amplified bound to the single-valued frequency estimation scenario, producing a tighter privacy bound than existing works. Finally, we perform experiments on both synthetic and real-world datasets to demonstrate the effectiveness of our protocols.
MoreTranslated text
Key words
Differential privacy,frequency estimation,sampling,shuffling
AI Read Science
Must-Reading Tree
Example
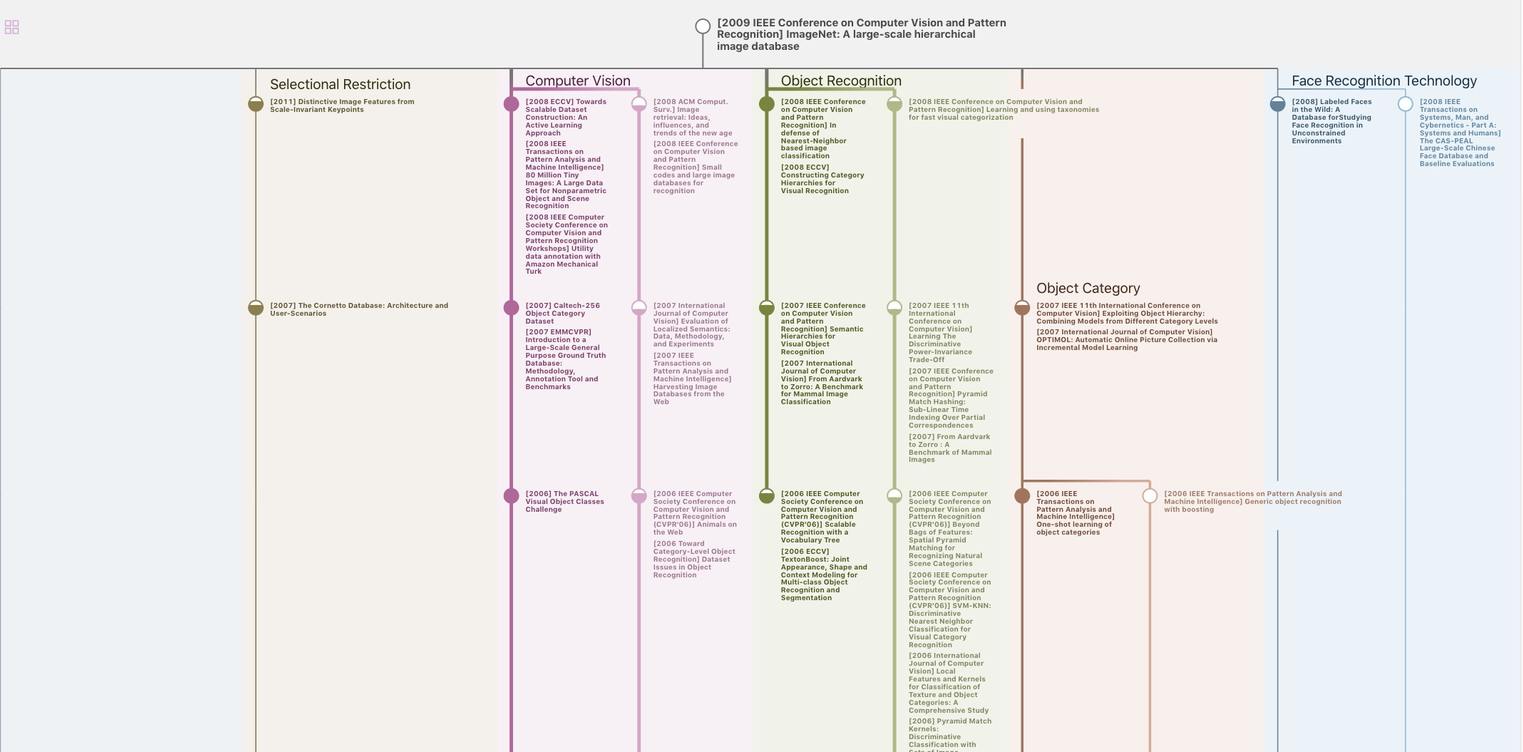
Generate MRT to find the research sequence of this paper
Chat Paper
Summary is being generated by the instructions you defined