Change Point Detection in Multi-Channel Time Series Via a Time-Invariant Representation
IEEE Transactions on Knowledge and Data Engineering(2023)
摘要
Change Point Detection (CPD) refers to the task of identifying abrupt changes in the characteristics or statistics of time series data. Recent advancements have led to a shift away from traditional model-based CPD approaches, which rely on predefined statistical distributions, toward neural network-based and distribution-free methods using autoencoders. However, many state-of-the-art methods in this category often neglect to explicitly leverage spatial information across multiple channels, making them less effective at detecting changes in cross-channel statistics. In this paper, we introduce an unsupervised, distribution-free CPD method that explicitly incorporates both temporal and spatial (cross-channel) information in multi-channel time series data based on the so-called Time-Invariant Representation (TIRE) autoencoder. Our evaluation, conducted on both simulated and real-life datasets, illustrates the significant advantages of our proposed multi-channel TIRE (MC-TIRE) method, which consistently delivers more accurate CPD results.
更多查看译文
关键词
Autoencoder,change point detection,multi-channel time series,unsupervised learning
AI 理解论文
溯源树
样例
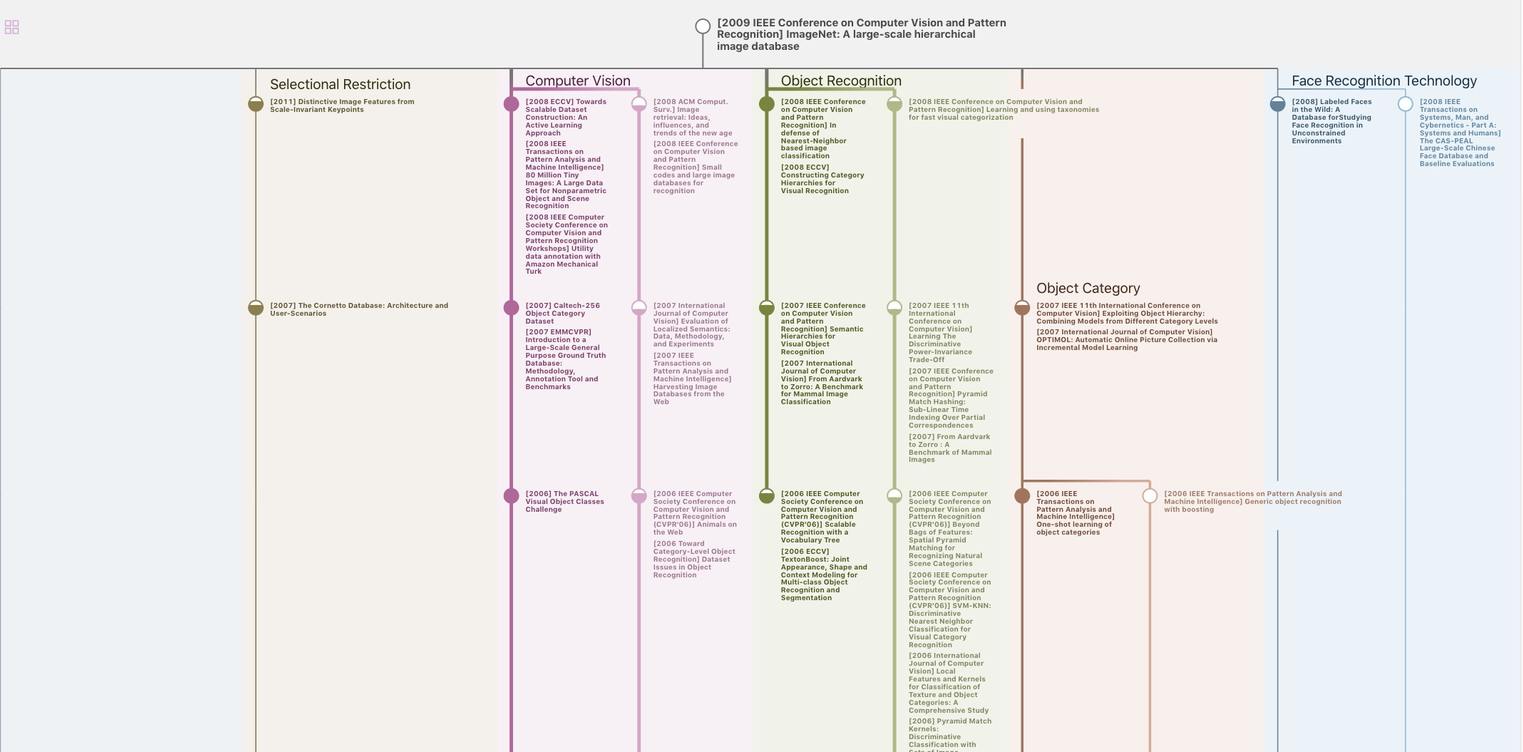
生成溯源树,研究论文发展脉络
Chat Paper
正在生成论文摘要