On Data Distribution Leakage in Cross-Silo Federated Learning
IEEE Transactions on Knowledge and Data Engineering(2024)
摘要
Federated learning (FL) has emerged as a promising privacy-preserving machine learning paradigm, enabling data owners to collaboratively train a joint model by sharing model parameters instead of private training data. However, recent studies reveal the privacy risks in FL by inferring private training data from model parameters. Therefore, differential privacy (DP) is incorporated into FL to safeguard training data. Nevertheless, DP does not provide a strong theoretical guarantee for protecting data distribution, which is also highly sensitive in the
cross-silo
FL scenarios as it may reflect the business secrets of data owners. In this paper, we develop two attack methods to investigate the potential risks of data distribution leakage in differentially private cross-silo FL. We highlight that an honest-but-curious server can successfully infer both the feature and label distributions of each party's training data without any background knowledge. Specifically, the first attack applies when models are differentiable, while the second attack caters to non-differentiable classification models. Extensive experiments on six benchmark datasets validate the effectiveness of the proposed attacks. The results demonstrate that the state-of-the-art DP-SGD algorithm is still vulnerable to the inference attack on data distribution, emphasizing the necessity of designing more advanced privacy-preserving FL frameworks.
更多查看译文
关键词
federated learning, data distribution leakage, differential privacy
AI 理解论文
溯源树
样例
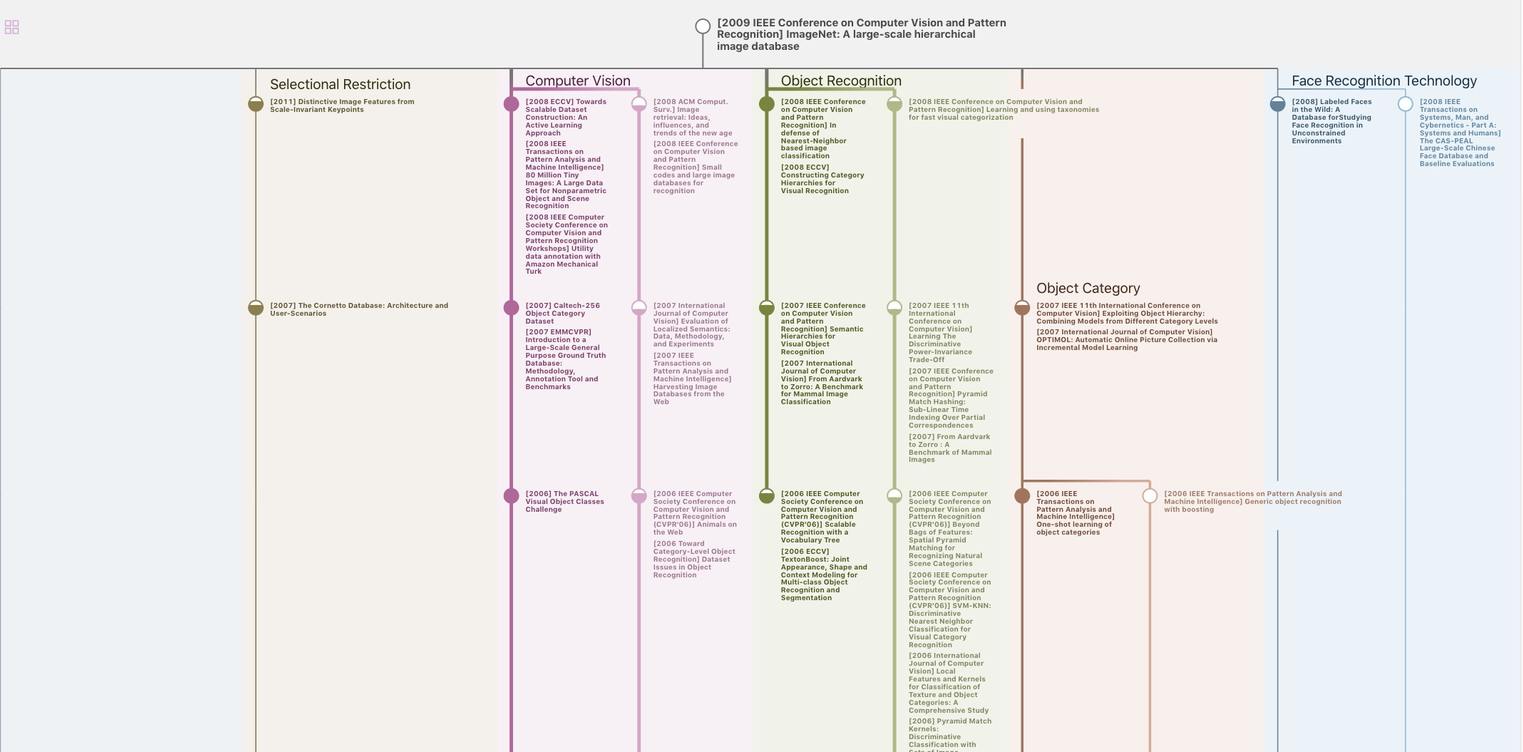
生成溯源树,研究论文发展脉络
Chat Paper
正在生成论文摘要