An Effective Point Cloud Registration Method Based on Robust Removal of Outliers
IEEE TRANSACTIONS ON GEOSCIENCE AND REMOTE SENSING(2024)
Abstract
Point cloud registration (PCR) is a vital technique in photogrammetry and computer vision. It seeks to identify optimal spatial transformation parameters for adjacent point clouds. In contrast methods based on an initial estimate, PCR based on correspondence operates without the need for an initial guess. However, these correspondences, often established through feature descriptors, can contain a significantly high rate of outliers. Existing methods struggle to balance efficiency and precision effectively. This article, building on matches, constructs an undirected graph and proposes a strategy for preferred correspondences based on the maximum cliques (MC) of reliable edges, thereby selecting potential correspondences based on the reliable edges and MC. The registration challenge is then divided into two separate components: estimating rotation and translation. The rotation matrix is calculated utilizing the adaptive Maxwell-Boltzmann (AMB) algorithm, while the translation vector is derived from the median of the confidence interval (MCI). Comprehensive tests on both simulated and real registration datasets demonstrate that our approach excels in precise PCR, even with outlier rates above 99%. The source code will be available at https://github.com/lixiaoyao0302/RoRO.
MoreTranslated text
Key words
Point cloud compression,Reliability,Estimation,Three-dimensional displays,Transmission line matrix methods,Graph theory,Cost function,Adaptive Maxwell(!-!)Boltzmann (AMB),median of the confidence interval (MCI),outlier,point cloud registration (PCR),preferred correspondence
AI Read Science
Must-Reading Tree
Example
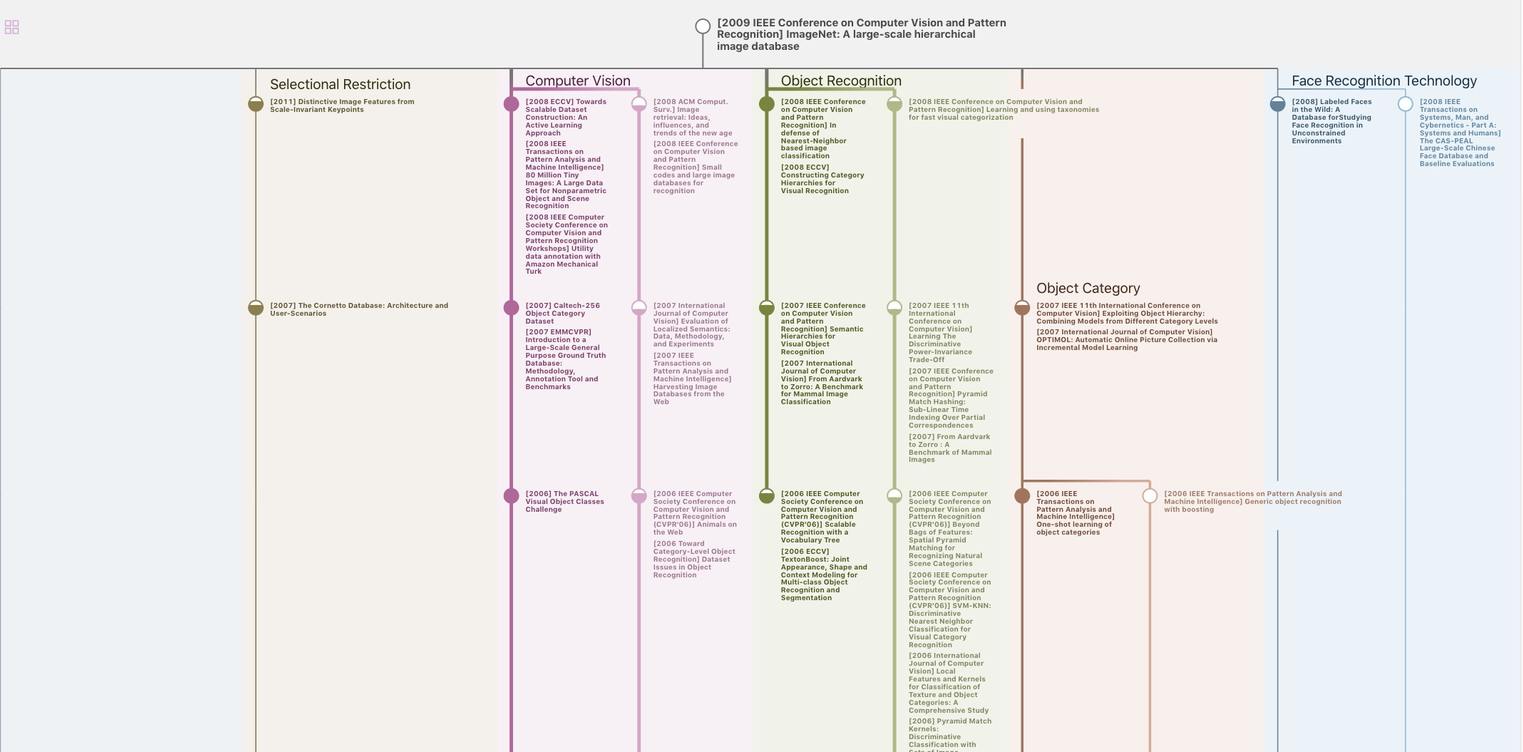
Generate MRT to find the research sequence of this paper
Chat Paper
Summary is being generated by the instructions you defined