HyperMLP: Superpixel Prior and Feature Aggregated Perceptron Networks for Hyperspectral and LiDAR Hybrid Classification
IEEE TRANSACTIONS ON GEOSCIENCE AND REMOTE SENSING(2024)
摘要
Hyperspectral images (HSIs) have excellent spectral combining capabilities and light detection and ranging (LiDAR) images have fine stereoscopic elevation information. Therefore, the multimodal fusion classification of hyperspectral and LiDAR images inevitably improves the interpretation ability of remote sensing images. In recent years, the MLP-Mixer, an image processing network based on multilayer perceptron (MLP), has flourished in the field of image processing. In this work, we propose an innovative HyperMLP network based on the deep learning framework MLP-Mixer architecture to address the lack of spatial feature construction capability and the locality of multimodal feature fusion in naive networks. Specifically, first, the adoption of unsupervised superpixel embedding provides additional shallow morphological spatial feature information for the network, reduces the pressure of the feature extraction network, and enhances feature discrimination capabilities. Second, the feature scrambling strategy improves the diversity of features and strengthens the generalization of the network by enhancing interactions between different spatial features. Finally, by implementing the bilateral modulation strategy, feature fusion is applied at every stage of the deep network, reducing semantic drift between features. On three fiducial remote sensing datasets, classification tests are performed on the proposed HyperMLP network to verify its performance, and the results are definitely impressive.
更多查看译文
关键词
Hybrid modality classification,hyperspectral image (HSI),light detection and ranging (LiDAR),MLP-Mixer
AI 理解论文
溯源树
样例
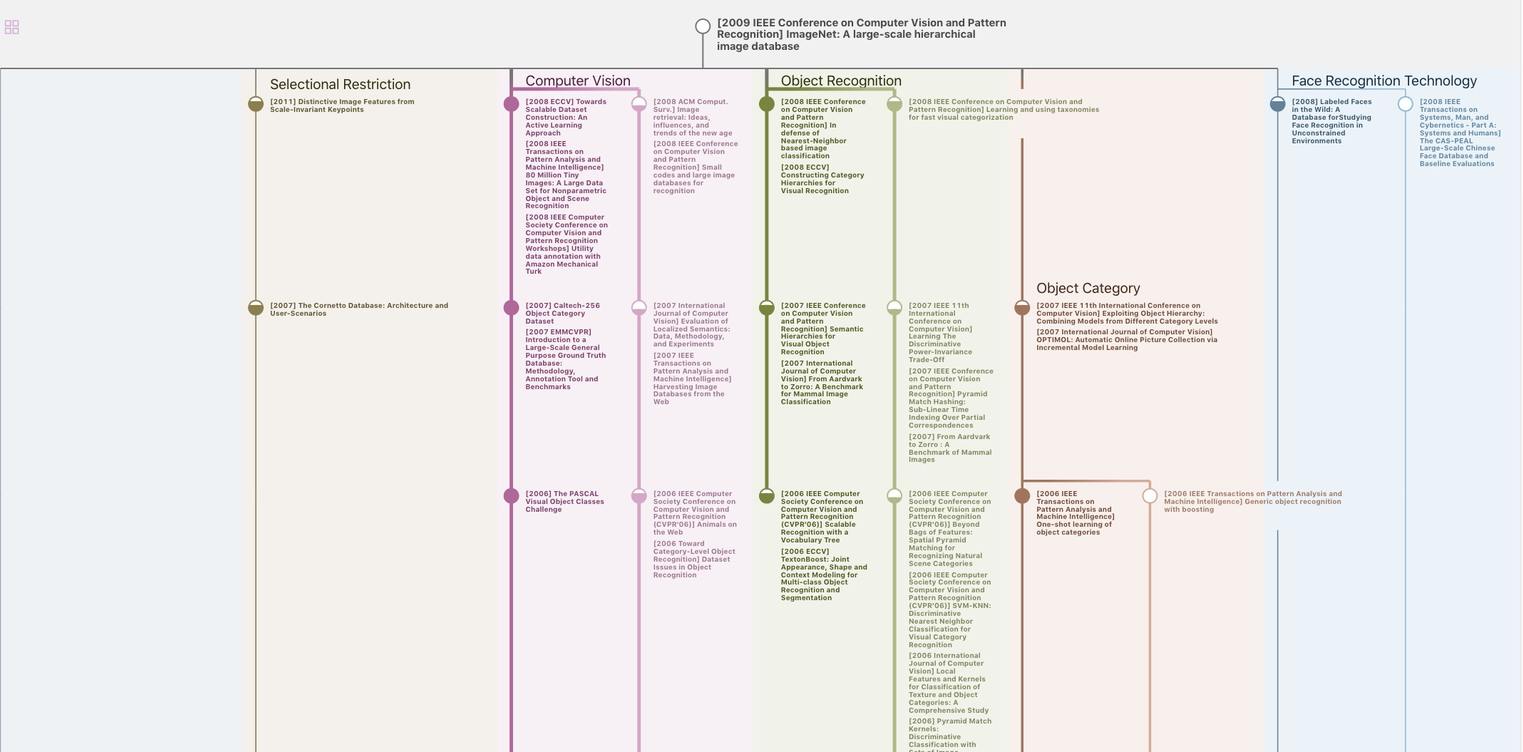
生成溯源树,研究论文发展脉络
Chat Paper
正在生成论文摘要