Object Knowledge Distillation for Joint Detection and Tracking in Satellite Videos
IEEE TRANSACTIONS ON GEOSCIENCE AND REMOTE SENSING(2024)
摘要
Existing mainstream multiobject tracking (MOT) methods can be categorized into two frameworks, including two- and one-stage ones. Two-stage ones divide MOT task into object detection and association tasks, which usually achieve high accuracy. One-stage ones train a joint model to achieve both detection and tracking. Therefore, their advantage usually lies in the high tracking efficiency. In this article, we inherit the advantages of the two types of frameworks and propose the object knowledge distilled joint detection and tracking framework (OKD-JDT) to achieve accurate as well as efficient tracking. First, the performance of two-stage methods largely depends on the highly performed detection network. Therefore, we treat the detection network as the teacher network to guide the discriminative object feature learning in one-stage methods by using knowledge distillation (KD). Then, in distillation learning, we design adaptive attention learning to learn the discriminative features from the teacher network to student network. In addition, with the similar appearance and uniform moving behavior of objects in satellite videos, we propose to use a joint center point distance and intersection over onion (IOU) to generate tracklets. Experiments on JiLin-1 satellite videos with different objects demonstrate the effectiveness and the state-of-the-art performance of the proposed method.
更多查看译文
关键词
Training,Videos,Task analysis,Satellites,Ions,Head,Feature extraction,Knowledge distillation (KD),multiobject tracking (MOT),satellite video
AI 理解论文
溯源树
样例
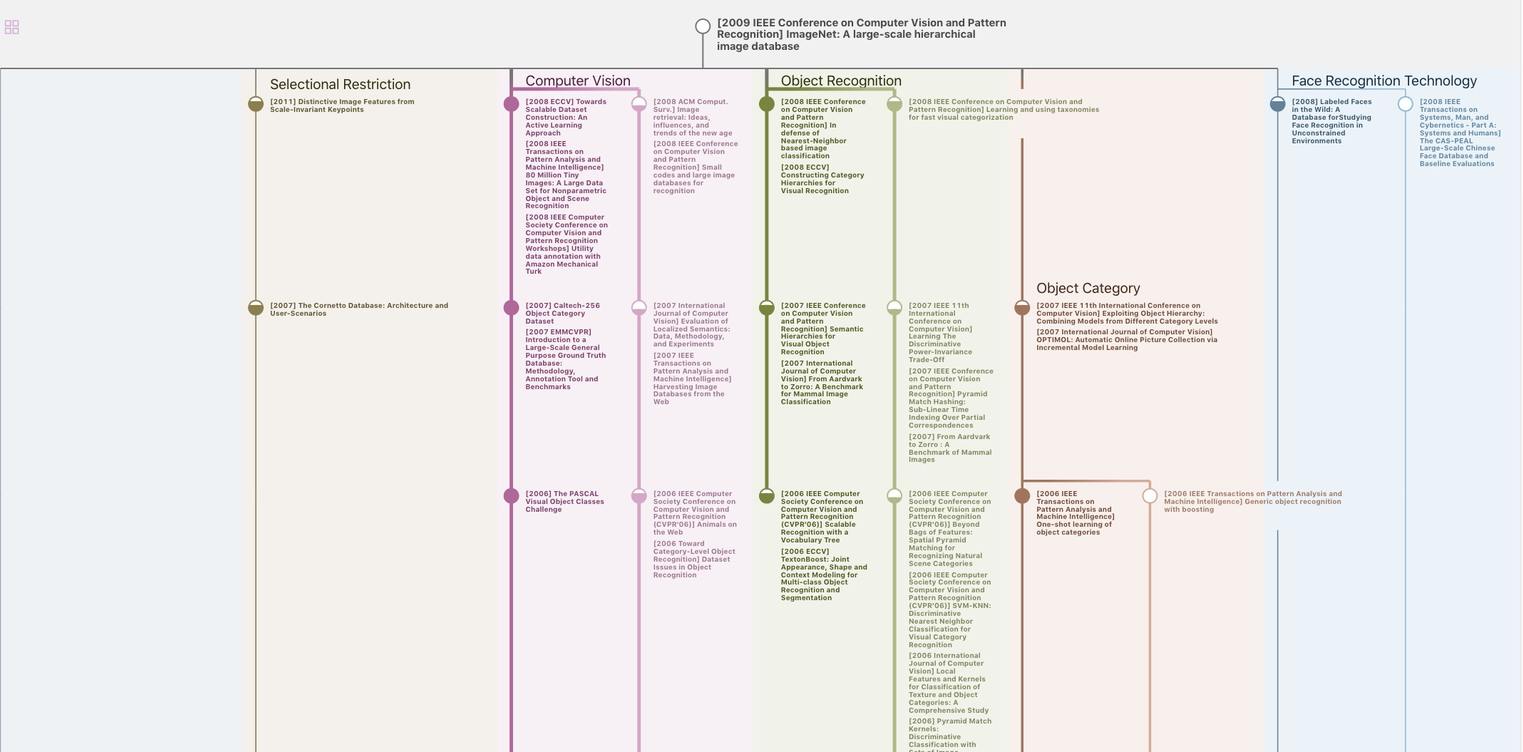
生成溯源树,研究论文发展脉络
Chat Paper
正在生成论文摘要