Seismic AVO Inversion Method for Viscoelastic Media Based on a Tandem Invertible Neural Network Model
IEEE TRANSACTIONS ON GEOSCIENCE AND REMOTE SENSING(2024)
摘要
Seismic amplitude variation with offset (AVO) inversion provides elastic parameters for reservoir identification. When processing field seismic data, conventional elastic medium AVO inversion methods typically inadequately account for the absorption of seismic waves by subsurface media, and inverted elastic parameters have accuracy upper bounds. The absorption and attenuation characteristics of subsurface media are described by quality factors introduced by viscoelastic media. The accuracy of inverted parameters will increase by studying AVO inversion methods based on viscoelastic media. Typically, low-frequency elastic parameters or conventional training samples affect how accurate conventional AVO inverted elastic parameters are. Since quality factors are typically absent from well-log data, it is challenging to produce suitable low-frequency elastic parameters and training samples. To address this issue, we propose an AVO inversion method for the viscoelastic method based on invertible neural networks (INNs) with bijective structures. We first construct a tandem INN for fitting the bidirectional mapping between elastic parameters and seismic data. Then, training parameters, which are easier to obtain than conventional training samples, are randomly generated based on the characteristics of the target work area data. Next, the forward process of the tandem INN is trained to fit the forward process from elastic parameters to seismic data. Finally, elastic parameter inversion is achieved through the reverse process of the trained tandem INN. The proposed method does not require initial elastic parameters and training samples. Model and field data tests prove that the proposed method is feasible, practical, and progressive.
更多查看译文
关键词
Invertible neural network (INN),quality factor,seismic amplitude variation with offset (AVO) inversion,viscoelastic media
AI 理解论文
溯源树
样例
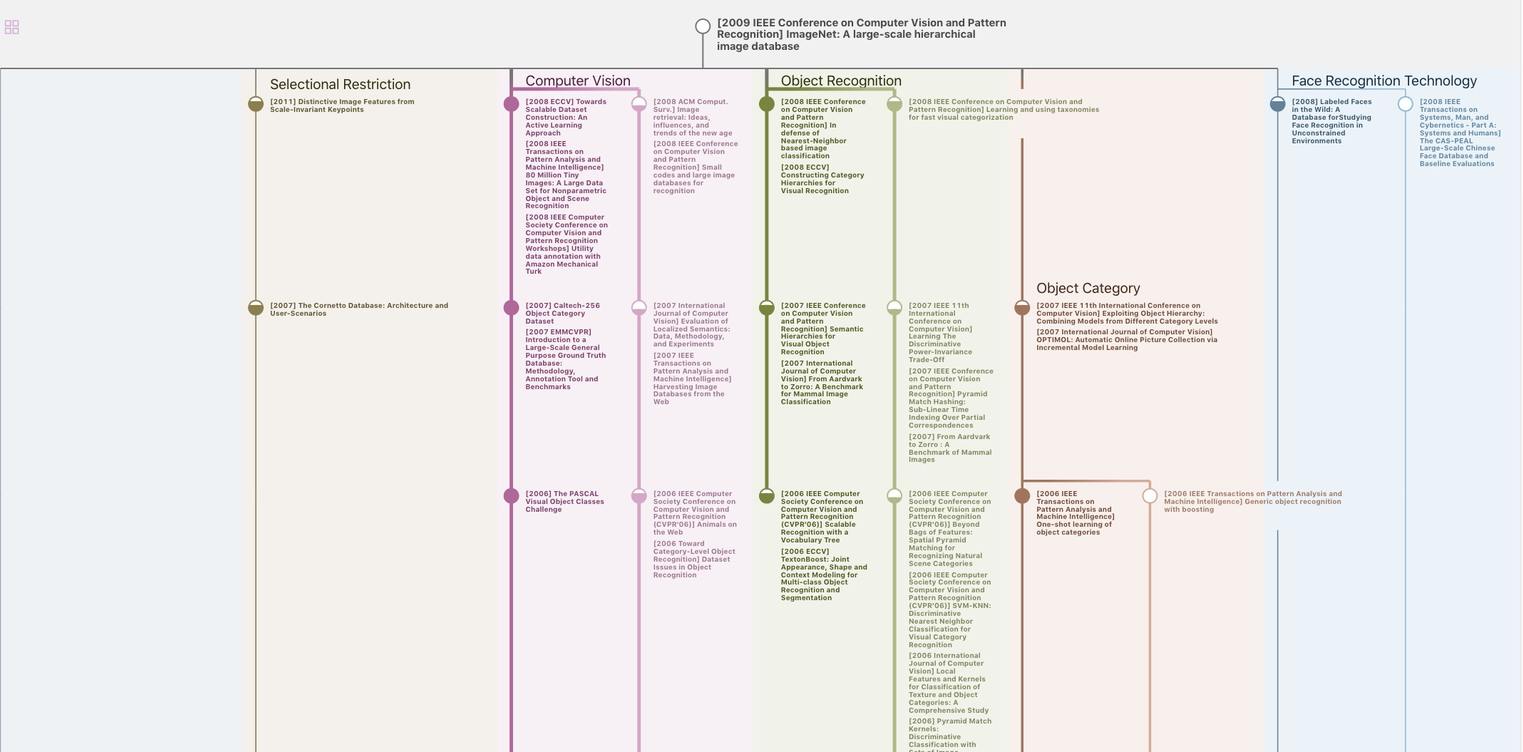
生成溯源树,研究论文发展脉络
Chat Paper
正在生成论文摘要