ITNet: Low-Shot Instance Transformation Network for Weakly Supervised Object Detection in Remote Sensing Images
IEEE TRANSACTIONS ON GEOSCIENCE AND REMOTE SENSING(2024)
摘要
Several studies of weakly supervised learning have been applied to object detection in remote sensing images (RSIs), while critical challenges like part domination and class confusion remain, which lead to poor accuracy compared with fully supervised object detection tasks (e.g., FRCNN and YOLOv4) and natural images set tasks (e.g., PASCAL VOC 2007). The model is prone to focus on the most discriminate part of the object due to the fact that image-level annotations are lack of instances' box information. Moreover, class confusion arises when the model is attempted to recognize instances of different categories that consistently coexist within a single training sample. To address the problems, we developed the low-shot instance transformation network (ITNet). ITNet is able to transform part domination boxes and misidentified confusion classes to be more accurate, which is trained with a combination of a small number of strong annotations and weak annotations. First of all, elastic cluster selection (ECS) is proposed to mine high-quality weak pseudoannotations from the output of only weakly supervised object detection (WSOD) models [e.g., online instance classifier refinement (OICR)]. Label reassignment (LRA) allows the correction of weak pseudoannotations with category noise by the recognition knowledge learned in strong annotations to alleviate two-class confusion. Then, semisupervised elastic match (SSEM) is employed to update the annotations to make full use of strong annotations. Comprehensive experiments are carried out on NWPU VHR-10.v2 and DIOR, proving that the proposed ITNet outperforms the previous state-of-the-art significantly.
更多查看译文
关键词
Annotations,Object detection,Training,Task analysis,Remote sensing,Supervised learning,Sensors,Low-shot weakly supervised object detection (LS-WSOD),remote sensing images (RSIs),weakly supervised object detection (WSOD)
AI 理解论文
溯源树
样例
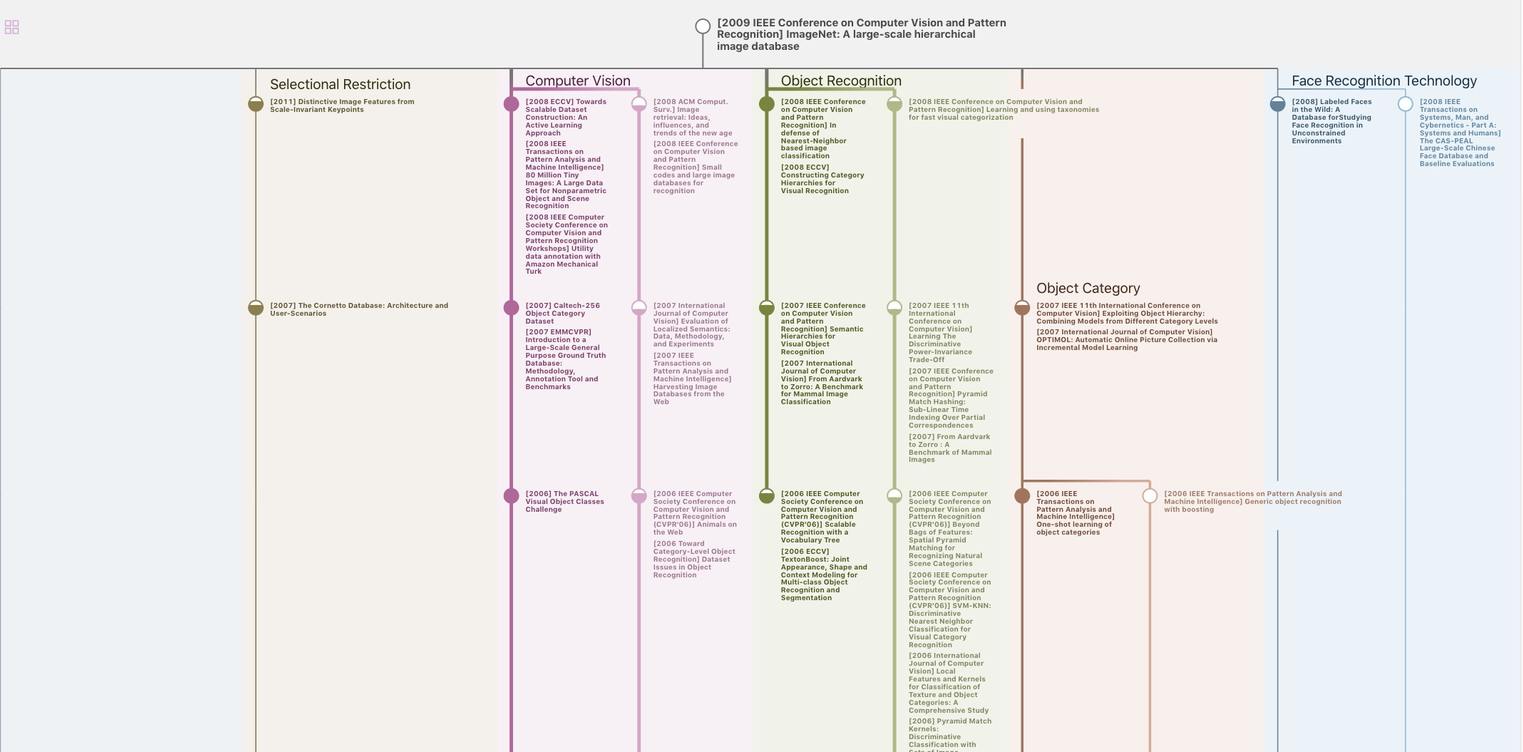
生成溯源树,研究论文发展脉络
Chat Paper
正在生成论文摘要