Radial Basis Function-based Adaptive Gain Super-Twisting Controller for Magnetic Levitation System with Time-Varying External Disturbance
IEEE Transactions on Transportation Electrification(2024)
摘要
Accurate and reliable control of a magnetic levitation (maglev) plant is challenging due to position tracking irregularities, parameter perturbations, external disturbances, and stringent dynamic requirements. In this study, a radial basis function neural network-based adaptive gain super-twisting sliding mode controller (RBF-ASTC) is proposed to achieve high-performance position control of the maglev system. The presence of unknown model parameter uncertainties and external disturbances limit the control performance of the classical and higher-order sliding-mode (HOSM) controller. Further, the fixed control gains often fail to generate optimal control responses in the presence of time-varying external disturbances. To address the above limitations, the proposed RBF-ASTC approach has been proposed in this research work. The key feature of the adaptation algorithm is that it can alleviate the problem of gain overestimation of the control input without prior knowledge of the uncertainty constraints. The proposed RBF-ASTC approach imparts immunity to the control scheme against the model parameter variations along with adaptive tuning of robust gains in the controller. Simulations and practical experiments have been performed to demonstrate the superiority of the proposed approach in achieving precise reference tracking, minimizing control effort/input, mitigating the impact of model parameter variations, and suppressing output oscillations to enhance the maglev system’s overall performance. In addition, it also show-cases a decent transient response in terms of peak overshoot and settling time.
更多查看译文
关键词
Magnetic levitation system (MLS),higher order sliding mode (HOSM) control,radial basis function (RBF) neural network,adaptive gain super-twisting control (ASTC),Lyapunov stability
AI 理解论文
溯源树
样例
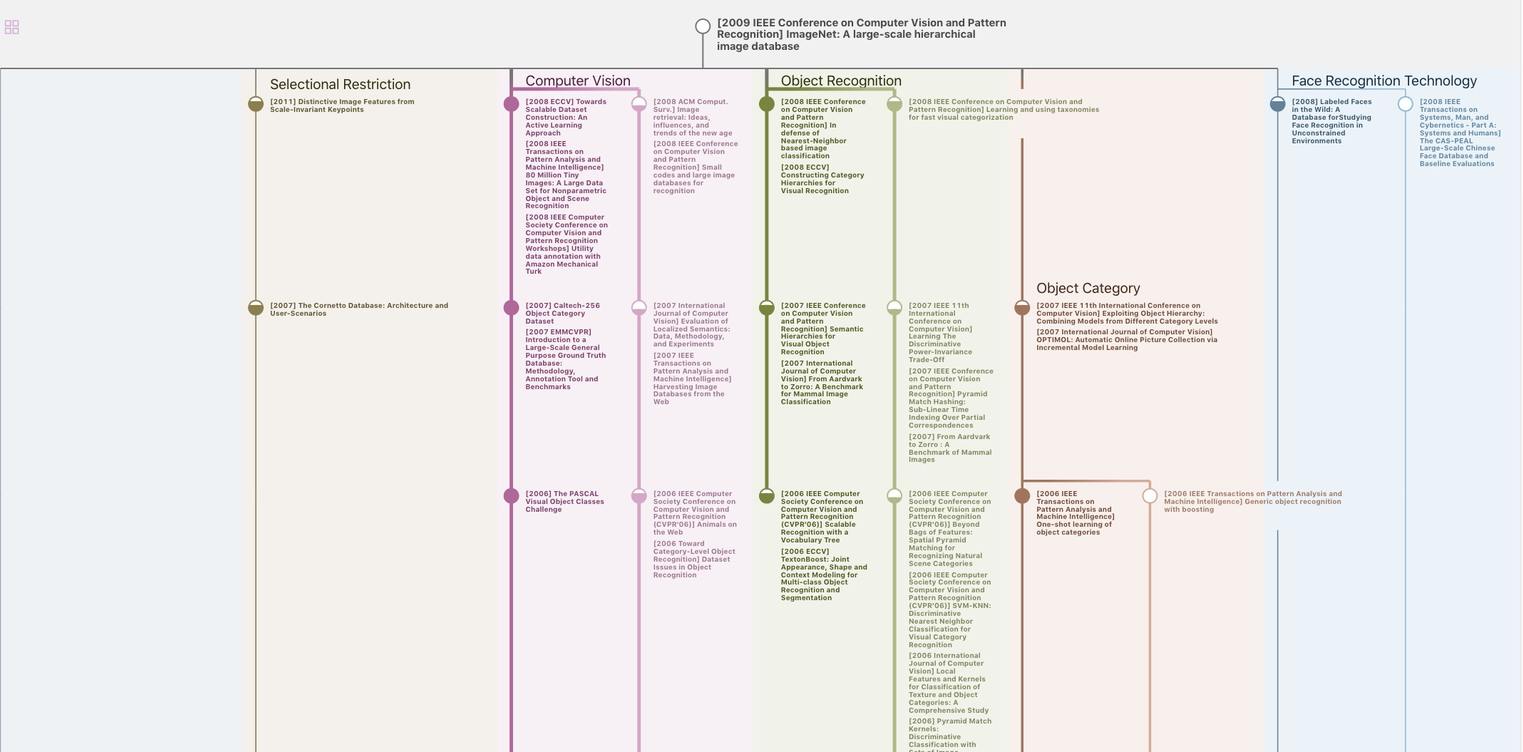
生成溯源树,研究论文发展脉络
Chat Paper
正在生成论文摘要