Detecting Cloud Anomaly via Broad Network-Based Contrastive Autoencoder
IEEE Transactions on Network and Service Management(2024)
摘要
Anomaly detection is indispensable for achieving higher availability and reliability in the cloud computing. The traditional autoencoder-based method only models the historical normal samples and then identifies the current online anomaly samples by the fixed threshold of anomaly score. Although more advances have been made in recent years, two main challenges remain: (i) ignoring the historical anomaly samples, (ii) poor self-adaptive ability for online detection. To address the above challenges, we propose a unified detector, namely BroadCAE, which integrates autoencoder with contrastive learning and broad network. Specifically, the reconstruction loss is first replaced by contrastive loss, which equally formulates both normal and anomaly samples. These samples belonging to the same class become closer in a lower-dimensional space. Conversely, different classes of samples are far away from each other. Next, we apply the anomaly-score-based pseudo thresholds to train the dynamic threshold selection, which generates the threshold according to the coming sample. The broad network in dynamic threshold selection takes the place of the deep network, which overcomes catastrophic forgetting and adapts to new online samples. Finally, validation experiments are conducted on four benchmark datasets. Our BroadCAE outperforms the comparative baseline methods by averaging over 4% of the f1-score.
更多查看译文
关键词
Anomaly detection,contrastive learning,broad network,cloud computing
AI 理解论文
溯源树
样例
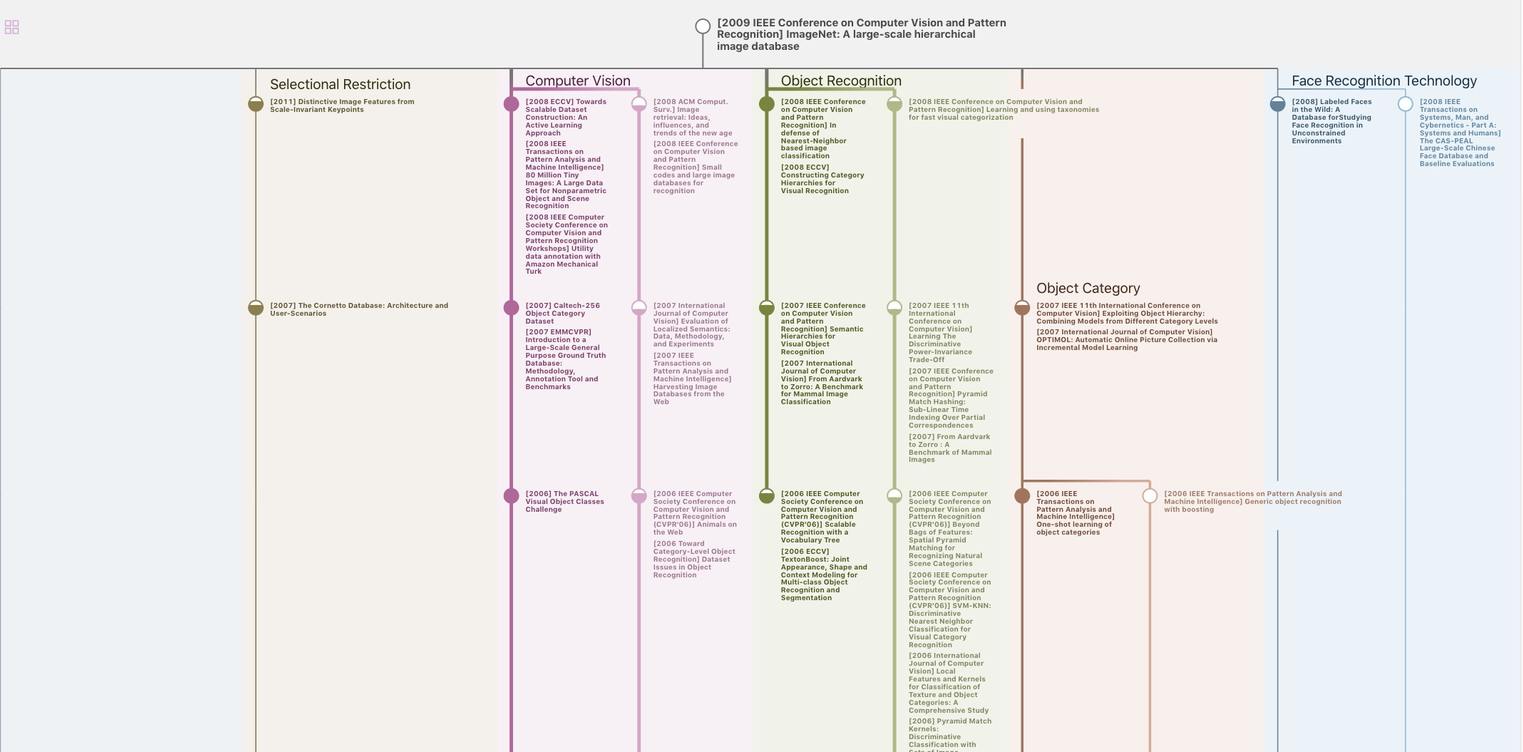
生成溯源树,研究论文发展脉络
Chat Paper
正在生成论文摘要