An Efficient Transient Electromagnetic Uncertainty 1-D Inversion Method Based on Mixture Density Network.
IEEE Transactions on Geoscience and Remote Sensing(2024)
摘要
The transient electromagnetic method (TEM) is one of the geophysical methods that can quickly detect underground space information. It obtains the resistivity structure of underground medium by inversion technology. The traditional inversion methods can only give a unique solution conforming to TEM data, but cannot solve the multiplicity problem of solutions. TEM Bayesian inversion technology can provide uncertainty information to solve this problem. However, its long calculation time makes it difficult to apply to engineering detection with high real-time performance. To solve these problems, a TEM one-dimensional inversion mixture density network (TEMIMDNet) is proposed in this paper. This method combines Bayesian theory with deep learning methods. By inputting TEM data into the trained network, the statistical parameters of the posterior probability density of the corresponding geological model can be quickly obtained. Thus, the uncertainty information of the geological model can be obtained. This method overcomes the problem of low efficiency of traditional Bayesian inversion. The simulated experiment shows that the TEMIMDNet method can not only obtain the probability density function graph of the geological model but also the average relative error between the maximum a posteriori model and the corresponding TEM response, which is less than 0.02. The field experiment shows that the TEMIMDNet method can output the statistical parameters of 52 survey points in only 4 ms, and the imaging results are consistent with the spatially constrained inversion method and OCCAM.
更多查看译文
关键词
Mixture density network (MDN),transient electromagnetic method (TEM),uncertainty inversion
AI 理解论文
溯源树
样例
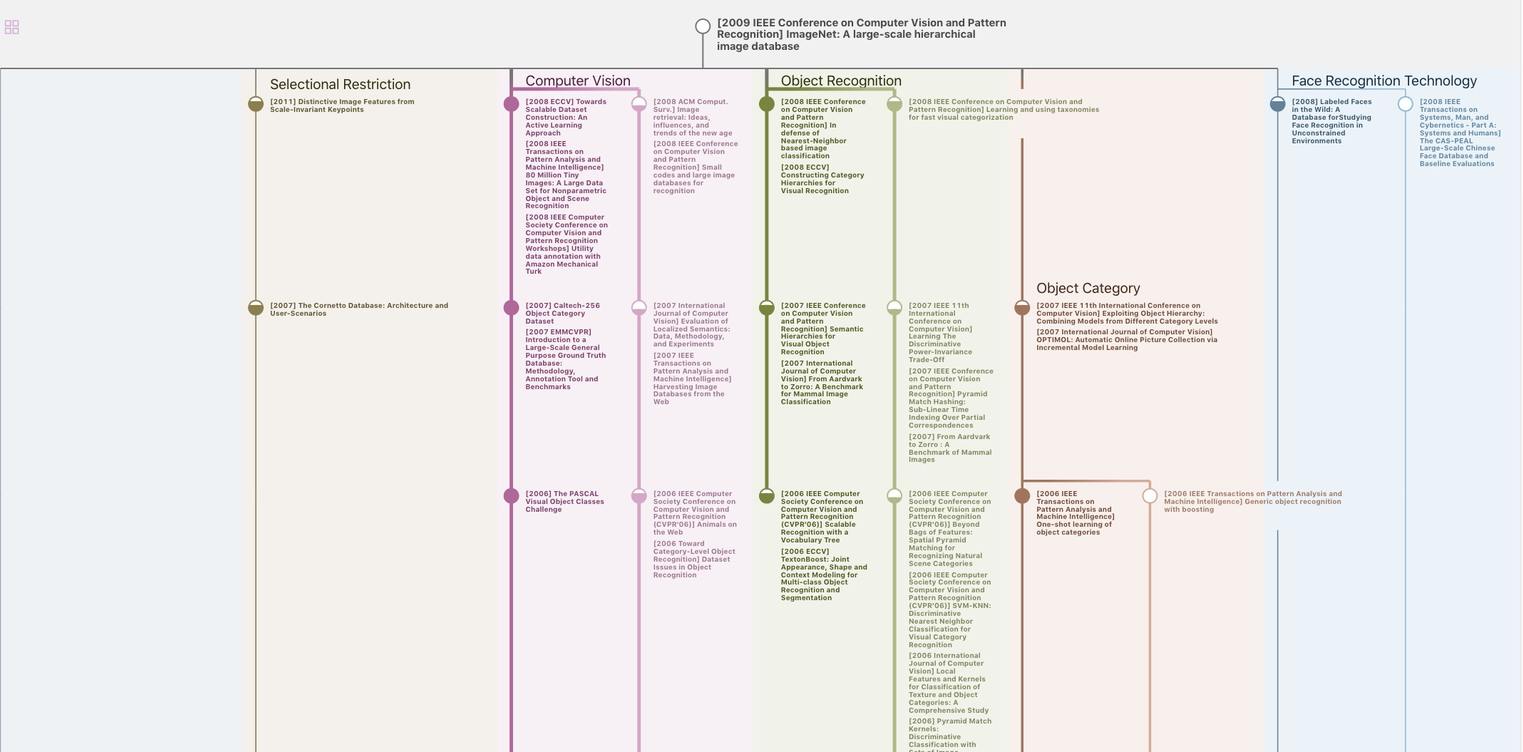
生成溯源树,研究论文发展脉络
Chat Paper
正在生成论文摘要