CDCM: ChatGPT-Aided Diversity-Aware Causal Model for Interactive Recommendation.
IEEE Trans. Multim.(2024)
摘要
In recent years, interactive recommender systems (IRSs) have attracted extensive interest. Existing IRSs are typically implemented with offline reinforcement learning (RL). They are devoted to improving recommendation accuracy by optimizing the extraction of users' inherent preferences. However, there hasn't been much attention on recommendation diversity, which could result in the monotony effect,
i.e.
, categories of recommended items are consistently fixed and unchanging. In this paper, we center on category diversification in IRSs while largely preserving or even boosting recommendation accuracy. To this end, we propose a ChatGPT-aided diversity-aware causal model (CDCM) to enhance the offline RL framework with causal inference and ChatGPT. Specifically, we first propose a diversity-aware causal user model (DCUM) to estimate user satisfaction. This model disentangles the causal effect of users' inherent preferences and the monotony effect to obtain user satisfaction with both accuracy and diversity. Then, DCUM is used to assist the RL agent in recommendation policy learning. A ChatGPT-aided state encoder (CSE) is proposed to provide user state representation for each time step of policy learning. With the help of ChatGPT, CSE incorporates multi-category information in line with users' potential preferences to promote diverse and relevant category recommendations. Extensive experiment results on two real-world datasets validate the superiority of our CDCM regarding both accuracy and diversity.
更多查看译文
关键词
Interactive recommendation,accuracy,diversity,monotony effect,causal inference,ChatGPT
AI 理解论文
溯源树
样例
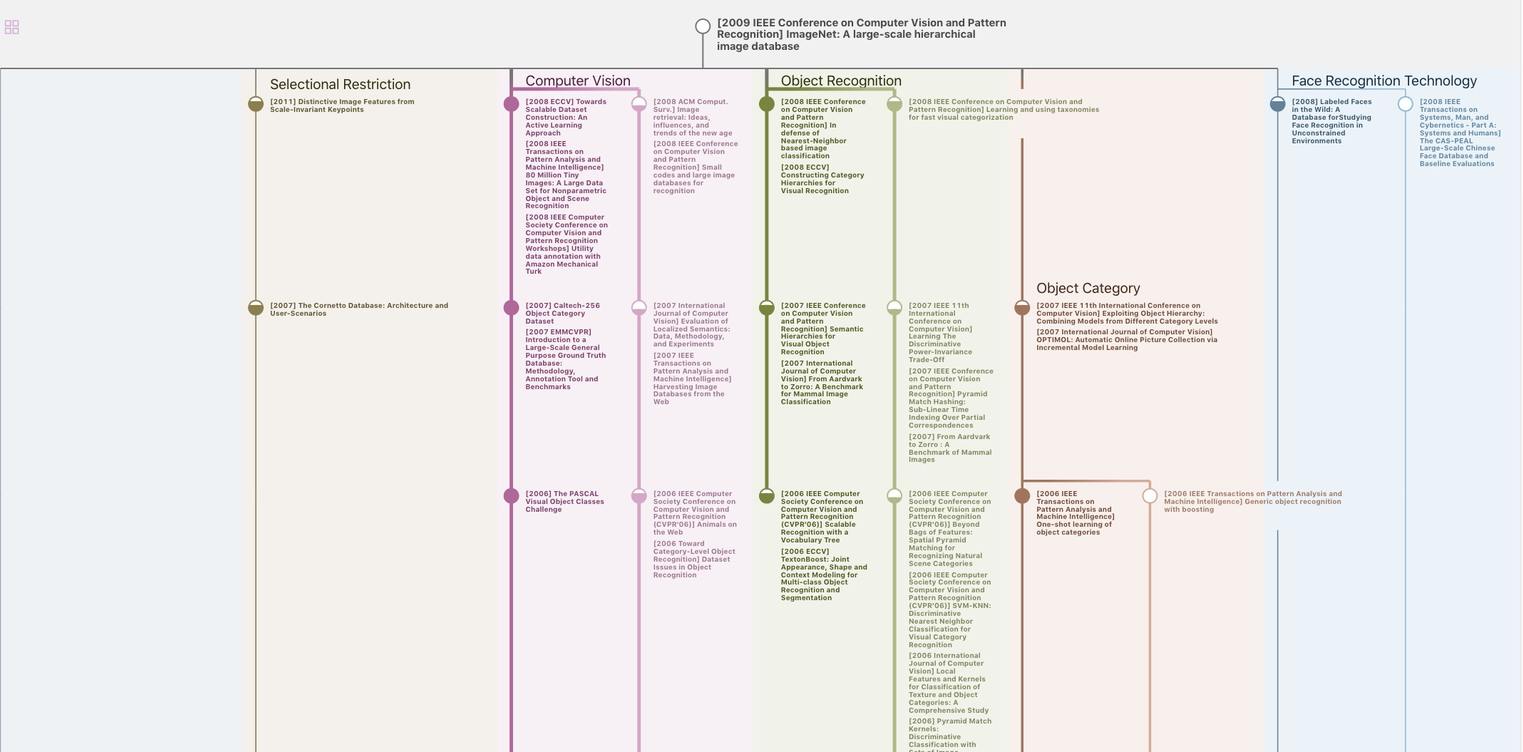
生成溯源树,研究论文发展脉络
Chat Paper
正在生成论文摘要