Cuffless Blood Pressure Estimation Model Using Clustering Techniques
IEEE Sensors Journal(2023)
摘要
This study introduces a clustered algorithm for estimating blood pressure (BP) using electrocardiographic and photoplethysmographic data obtained from 1675 subjects, corresponding to a total of 1051462 cardiac cycles. These data were sourced from the publicly accessible biosignal database, VitalDB. To enable the application of pulse wave analysis (PWA) on the recorded signals, the study extracted 29 morphological features from these signals and employed the K-means clustering algorithm to categorize them into six distinct clusters. Each of these clusters was used to train and optimize three separate BP estimation algorithms. Notably, the Shapley additive explanations analysis identified arterial stiffness and peripheral resistance as the most influential factors contributing to the clustering process. Experimental results reveal that the most accurate BP estimation model achieves deviations of 0.043 ± 6.43 mmHg for systolic BP and 0.052 ± 3.65 mmHg for diastolic BP. Remarkably, the adoption of clustering lead to notable enhancements in accuracy across key metrics, including mean error, standard deviation, and mean absolute error estimates. Importantly, these improvements align with the rigorous criteria established by three international BP standards: the Association for the Advancement of Medical Instrumentation/ISO, the British Hypertension Society, and the Institution of Electrical and Electronic Engineers. This study underscores the potential for clinical adoption of the clustering-based, cuffless BP estimation algorithm, which accommodates individual variations without necessitating a pretraining process for the subjects under investigation.
更多查看译文
关键词
Cuffless blood pressure estimation,pulse wave analysis,hypertension,clustering
AI 理解论文
溯源树
样例
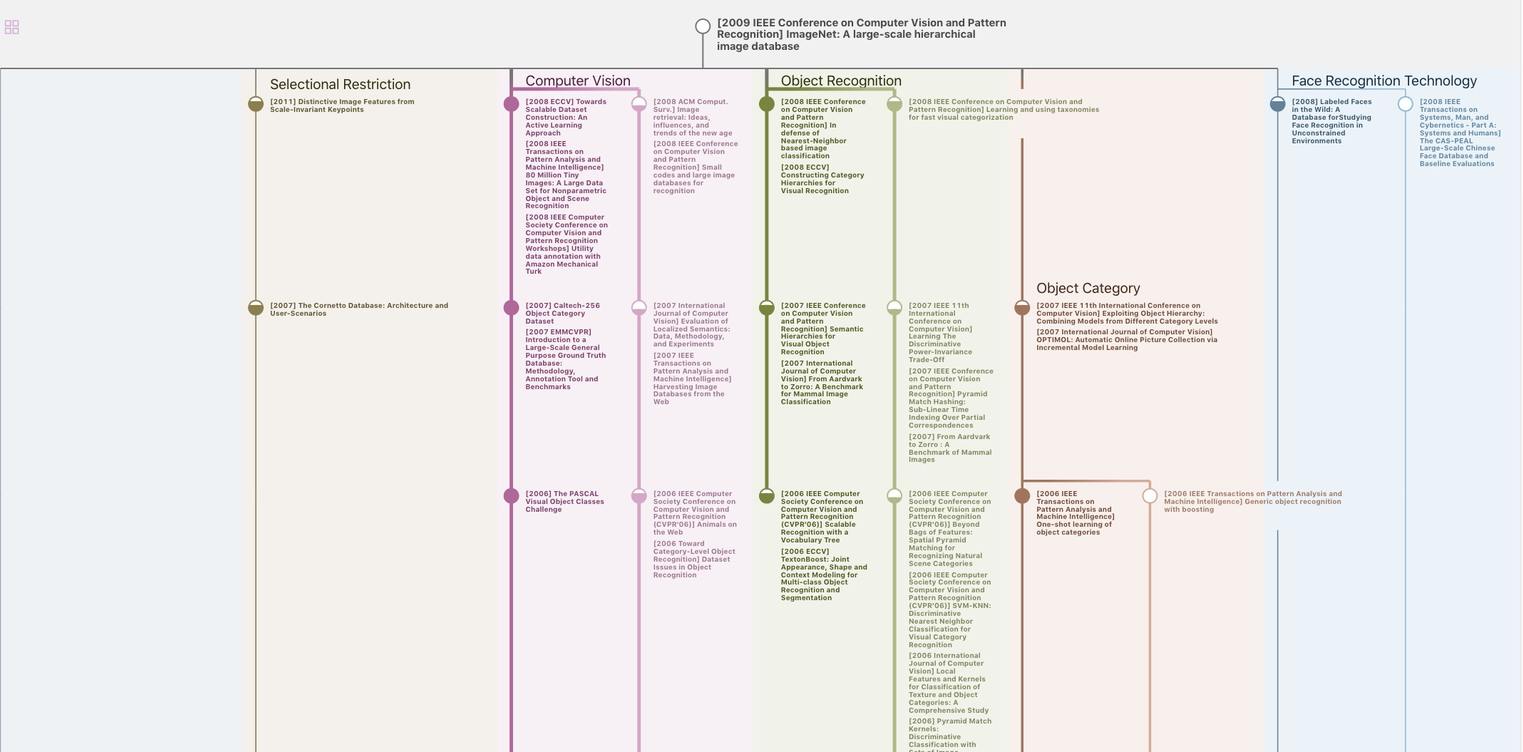
生成溯源树,研究论文发展脉络
Chat Paper
正在生成论文摘要