Multi-Interaction Trajectory Prediction Method With Serial Attention Patterns for Intelligent Vehicles
IEEE Transactions on Vehicular Technology(2024)
摘要
Accurately predicting the trajectories of vehicles in a driving environment composed of various traffic participants is very significant for the driving safety of intelligent vehicles. The critical difficulties of trajectory prediction lie in clarifying the interaction between traffic participants and describing the uncertainty of a vehicle's driving intention. This article proposes a vehicle trajectory prediction method based on the combination of a serial dual attention mechanism and a sampling generation model in graph structure mode. The serial dual attention mechanism describes the impact of different types of traffic participants on the vehicle. The edge attention mechanism in the serial structure considers the features of nodes and the direction of interaction simultaneously to analyse the vehicle's degree of attention to each agent of the same kind of traffic participant. In addition, a driving intention representation module based on a conditional variational autoencoder (CVAE) maps the aggregated features of the vehicle and its surrounding nodes into the latent variable space to describe the distribution of the vehicle's driving intention. Experimental results on the nuScenes dataset indicate that our method is superior to state-of-the-art prediction frameworks. Our method can consider the impact of traffic participants on the vehicle at different levels and reasonably predict the multimodal trajectory of the vehicle according to its driving intention. The result of the real-vehicle experiment shows that our method can guarantee high prediction accuracy in the practical application as well.
更多查看译文
关键词
Vehicle trajectory prediction,Multiple interactions,Serial structure,Dual attention,Sampling generator
AI 理解论文
溯源树
样例
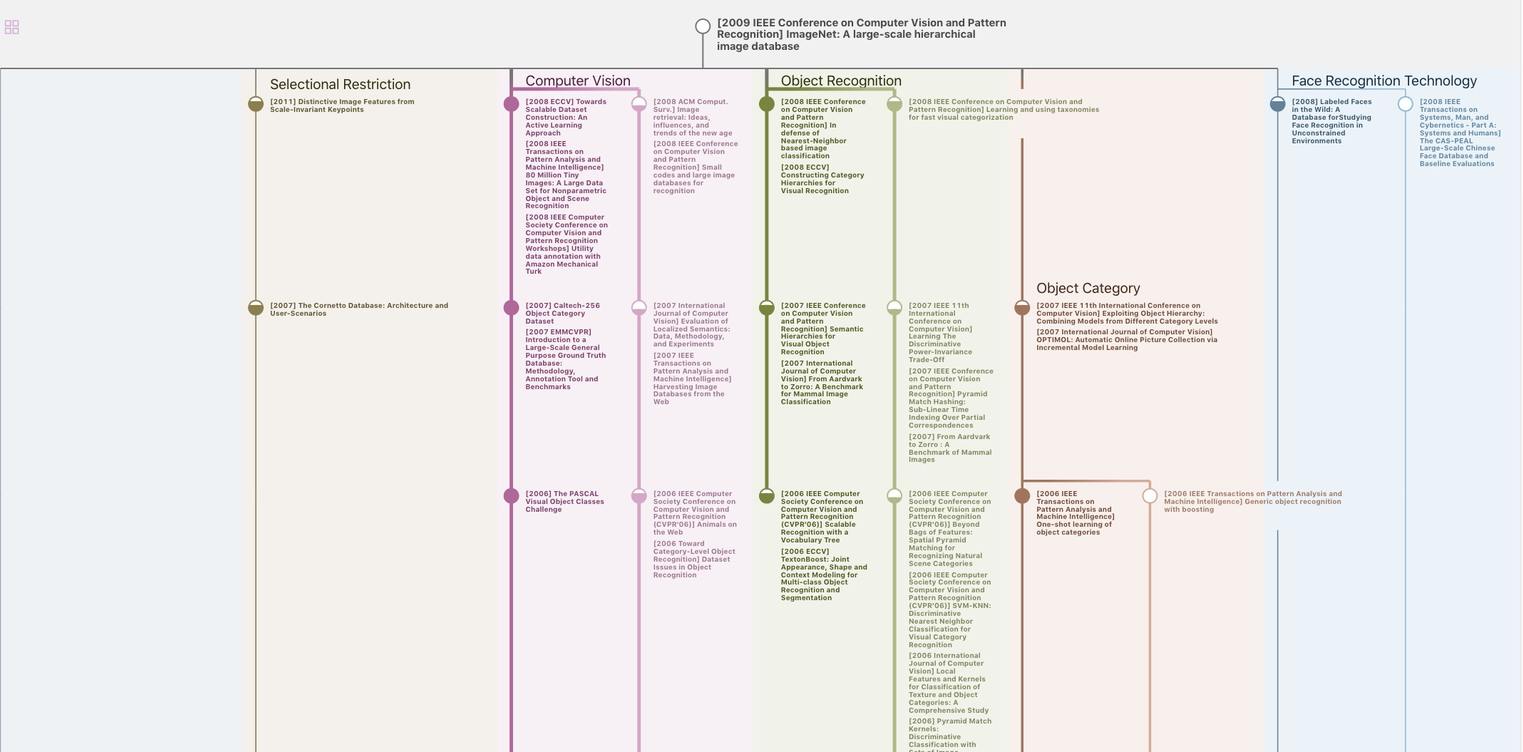
生成溯源树,研究论文发展脉络
Chat Paper
正在生成论文摘要