Dual Noise Elimination and Dynamic Label Correlation Guided Partial Multi-Label Learning
IEEE TRANSACTIONS ON MULTIMEDIA(2024)
摘要
Partial multi-label learning (PML) needs to address the problem of multi-label learning when the dataset contains redundant information. PML is more challenging compared to traditional multi-label learning, because PML needs not only to perform the multi classification task, but also to reduce the impact of noise information on the model. Existing PML methods suffer from the following problems. (1) Only single source of noise is considered. (2) Some methods ignore the label correlations. To solve the above problems, we proposes a new dual noise elimination and dynamic label correlation guided partial multi-label learning (PML-DNDC). Specifically, the hidden ground-truth label matrix is decomposed into two compressed matrices of instance and classifier, which are used to approximate the candidate label matrix, to eliminate the negative effects of label noise on the model. On one hand, the compressed instance matrix maintains local structural consistency with the original instances, eliminating noise in the feature. On the other hand, dynamic label correlation guidance is designed to help classifier training by dynamically exploring the potential label correlations, which encourages relevant labels to obtain similar classifiers. After extensive experiments and analyses, we conclude that the proposed PML-DNDC is superior to the state-of-the-art methods.
更多查看译文
关键词
Correlation,Matrix decomposition,Training,Phase locked loops,Laplace equations,Task analysis,Sun,Partial multi-label learning,noise elimination,label correlations,dynamic Laplacian matrix
AI 理解论文
溯源树
样例
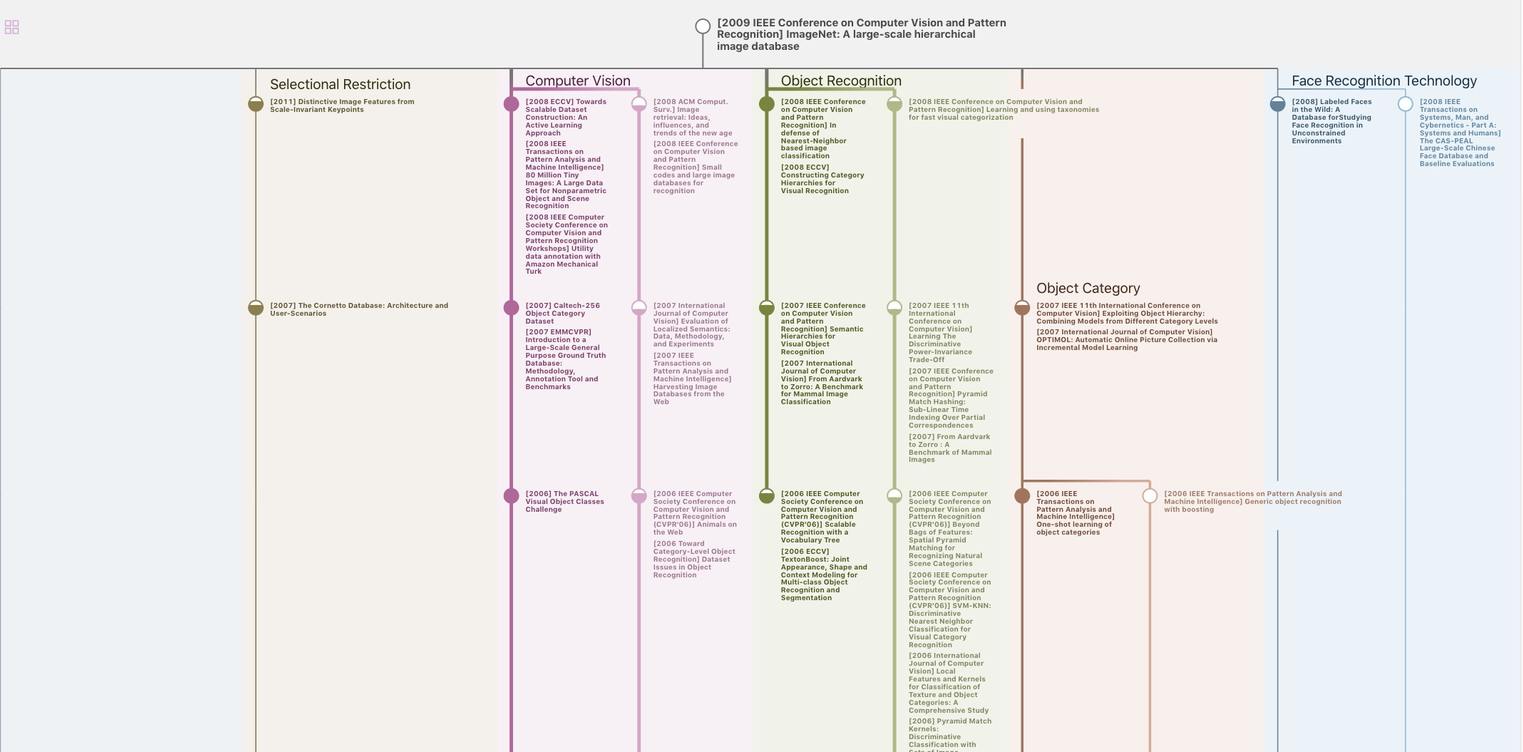
生成溯源树,研究论文发展脉络
Chat Paper
正在生成论文摘要