Multiclass and Multilabel Classifications by Consensus and Complementarity-Based Multiview Latent Space Projection
IEEE TRANSACTIONS ON SYSTEMS MAN CYBERNETICS-SYSTEMS(2024)
摘要
The fusion of multiview data sets, in which features of each sample are categorized into distinct groups, is increasingly important in the big data era. Successful multiview learning approaches have mechanisms to enforce consensus and/or complementarity among views. This article introduces a framework called the consensus and complementarity-based multiview latent space projection (MVLSP-2C) that enforces both principles simultaneously. Consensus is established by extracting and representing information shared by all views in a shared latent space, whereas complementarity among views is achieved by the representation in view-specific spaces. As the diversity of the multiview feature representation benefits classification performance, MVLSP-2C minimizes the similarity between the shared and view-specific representations, thereby improving diversity. The driving principle of MVLSP-2C is that the latent space representation is obtained by optimally projecting it to match the original feature space representation on a view-by-view basis. Unlike pairwise consensus methods that enforce consistency between two views, matching on a view-by-view basis allows extensions to settings with more than two views. A related and important advantage of this per-view matching design is that a class view can be readily incorporated to learn a supervised representation that facilitates subsequent classification. As the class view is added without an assumption on the exclusivity of classes, MVLSP-2C is equally applicable to multiclass single-label and multilabel classifications. MVLSP-2C further optimizes the integration of latent variables based on their correlation. Extensive experiments in multiclass and multiview image datasets show that MVLSP-2C produces more accurate classification results as compared to state-of-the-art methods.
更多查看译文
关键词
Latent variable correlation,multiclass classification,multilabel classification,multiview learning,shared latent space,view-specific latent space
AI 理解论文
溯源树
样例
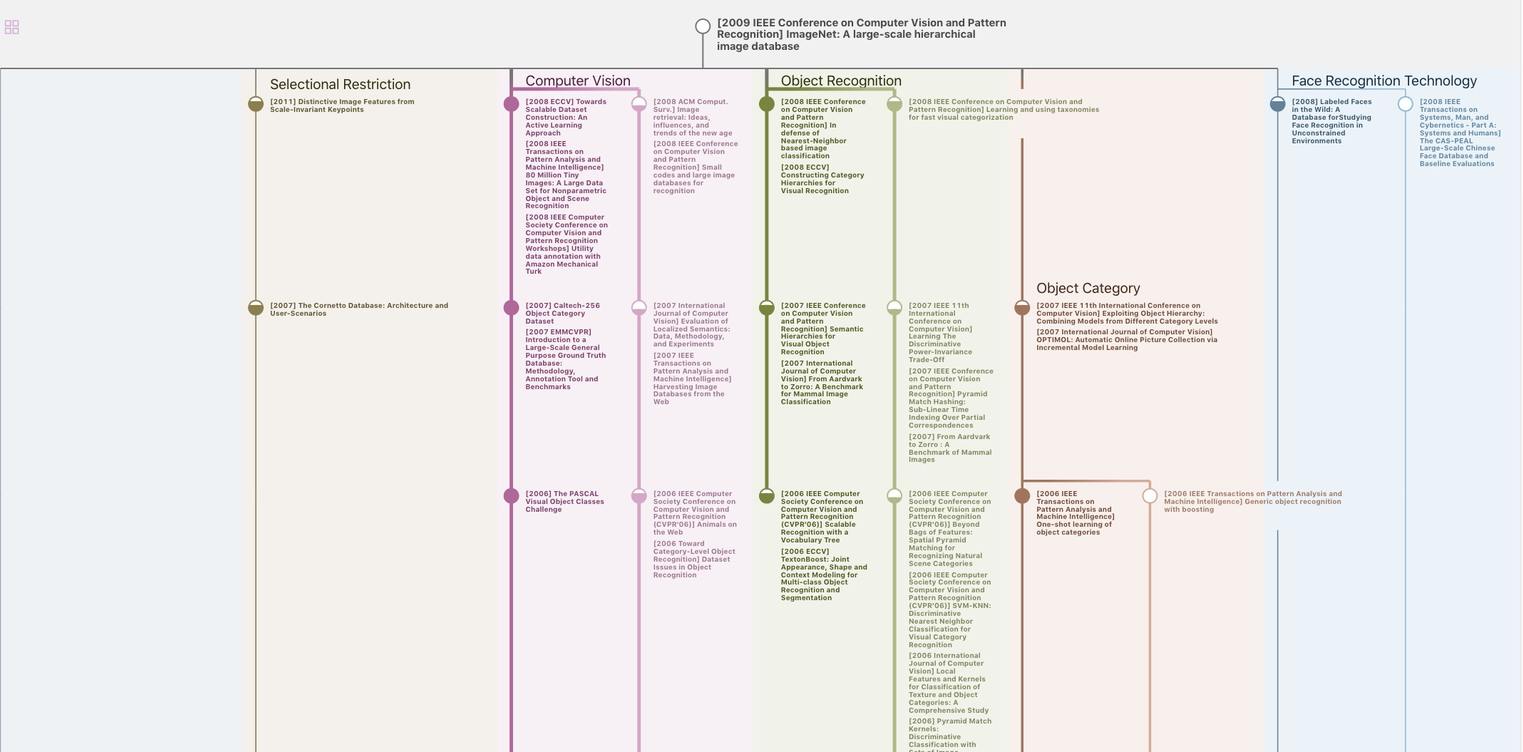
生成溯源树,研究论文发展脉络
Chat Paper
正在生成论文摘要