Enhanced Periodic Group Sparsity via Adaptive Period Estimation and Parameter Optimization for Rolling Bearing Fault Diagnosis
IEEE Sensors Journal(2023)
Abstract
Fault pulses of rolling bearings (RBs) are vulnerable to interference from noise and random shocks, which makes it exceedingly challenging to diagnose fault characteristics. To effectively diagnose the faults of the RBs, an enhanced periodic group sparsity (EPGS) method is developed based on the periodic overlapping group shrinkage (POGS) algorithm. Specifically, the proposed EPGS consists of three steps. First, according to the characteristic that the fault pulses of the RBs are periodic, an adaptive fault period estimation (AdaFPE) strategy is proposed, using which the period of the fault pulses (PFP) can be accurately predicted. Second, the proposed AdaFPE is embedded into the iterative process of the POGS for achieving dynamic update of the PFP, and then the EPGS method is constructed. Finally, a regularization parameter selection method is proposed, using which suitable regularization parameters can be matched according to the noise level of fault signal, which in turn improves the generalization of the EPGS to different fault signals. The proposed EPGS is validated using simulated and experimental signals. Simulation and experimental results demonstrate that the EPGS can extract the fault characteristics of the RBs from noisy vibration signals, which possesses superior estimation precision and diagnostic performance than the existing state-of-the-art methods, and has certain value for engineering application.
MoreTranslated text
Key words
Periodic group sparsity,Period estimation strategy,Parameter optimization,Rolling bearing,Fault diagnosis
AI Read Science
Must-Reading Tree
Example
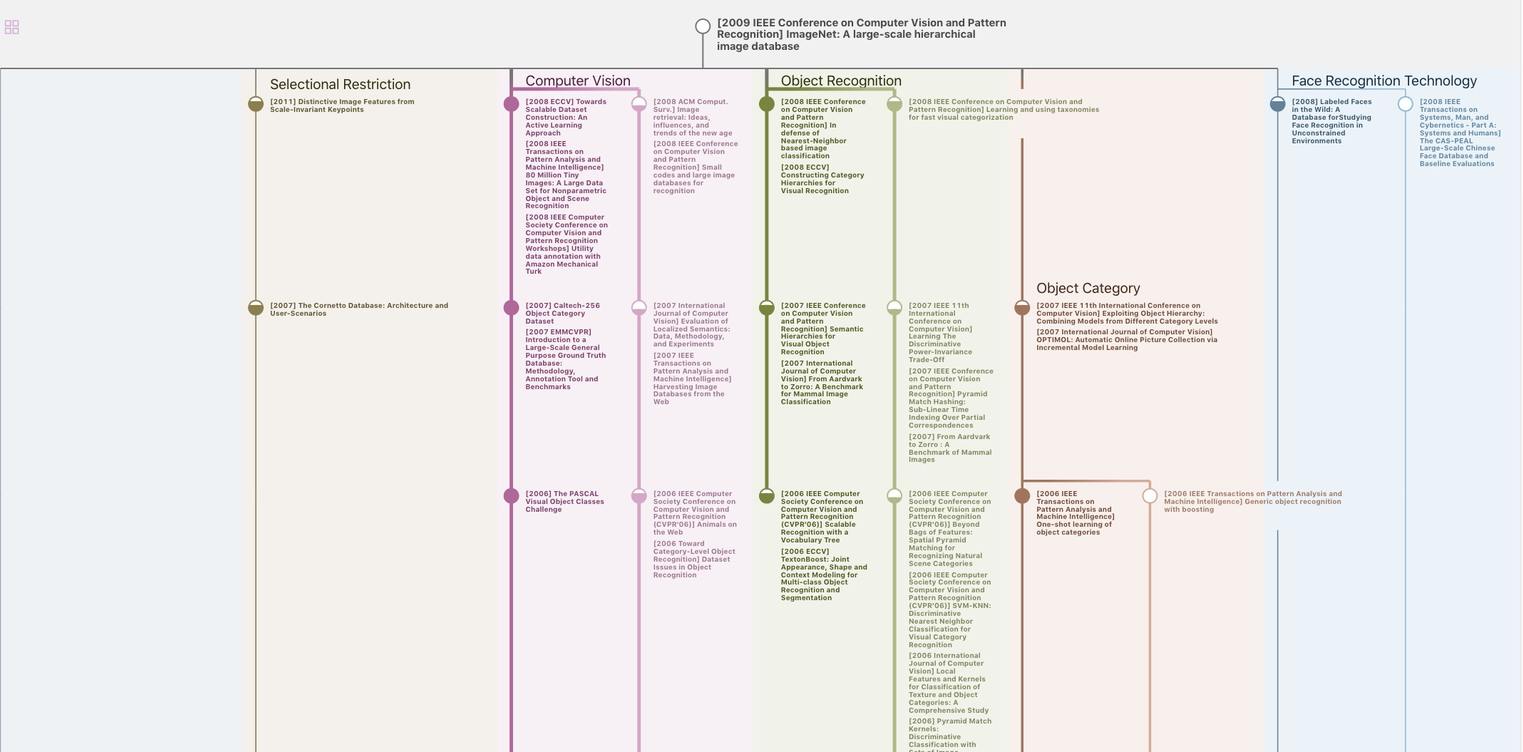
Generate MRT to find the research sequence of this paper
Chat Paper
Summary is being generated by the instructions you defined