Adaptive Machine Learning Head Model Across Different Head Impact Types Using Unsupervised Domain Adaptation and Generative Adversarial Networks
IEEE Sensors Journal(2024)
摘要
Machine learning head models (MLHMs) are developed to estimate brain deformation from sensor-based kinematics for early detection of traumatic brain injury (TBI). However, the overfitting to simulated impacts and the decreasing accuracy caused by distributional shift of different head impact datasets hinders the broad clinical applications of current MLHMs. We propose a new MLHM configuration that integrates unsupervised domain adaptation with a deep neural network to predict whole-brain maximum principal strain (MPS) and MPS rate (MPSR). With 12,780 simulated head impacts, we performed unsupervised domain adaptation on target head impacts from 302 college football (CF) impacts and 457 mixed martial arts (MMA) impacts using domain regularized component analysis (DRCA) and cycle-GAN-based methods. The new model improved the MPS/MPSR estimation accuracy, with the DRCA method outperforming other domain adaptation methods in prediction accuracy: MPS mean absolute error (MAE): 0.017 (CF) and 0.020 (MMA); MPSR MAE: 4.09 s
-1
(CF) and 6.61 s
-1
(MMA). On another two hold-out test sets with 195 college football impacts and 260 boxing impacts, the DRCA model outperformed the baseline model without domain adaptation in MPS and MPSR estimation MAE. The DRCA domain adaptation approach reduces the error of MPS/MPSR estimation to be well below previously reported TBI thresholds, enabling accurate brain deformation estimation to detect TBI in future clinical applications.
更多查看译文
关键词
Traumatic brain injury,unsupervised domain adaptation,strain and strain rate,kinematics sensor informatics,domain regularized component analysis
AI 理解论文
溯源树
样例
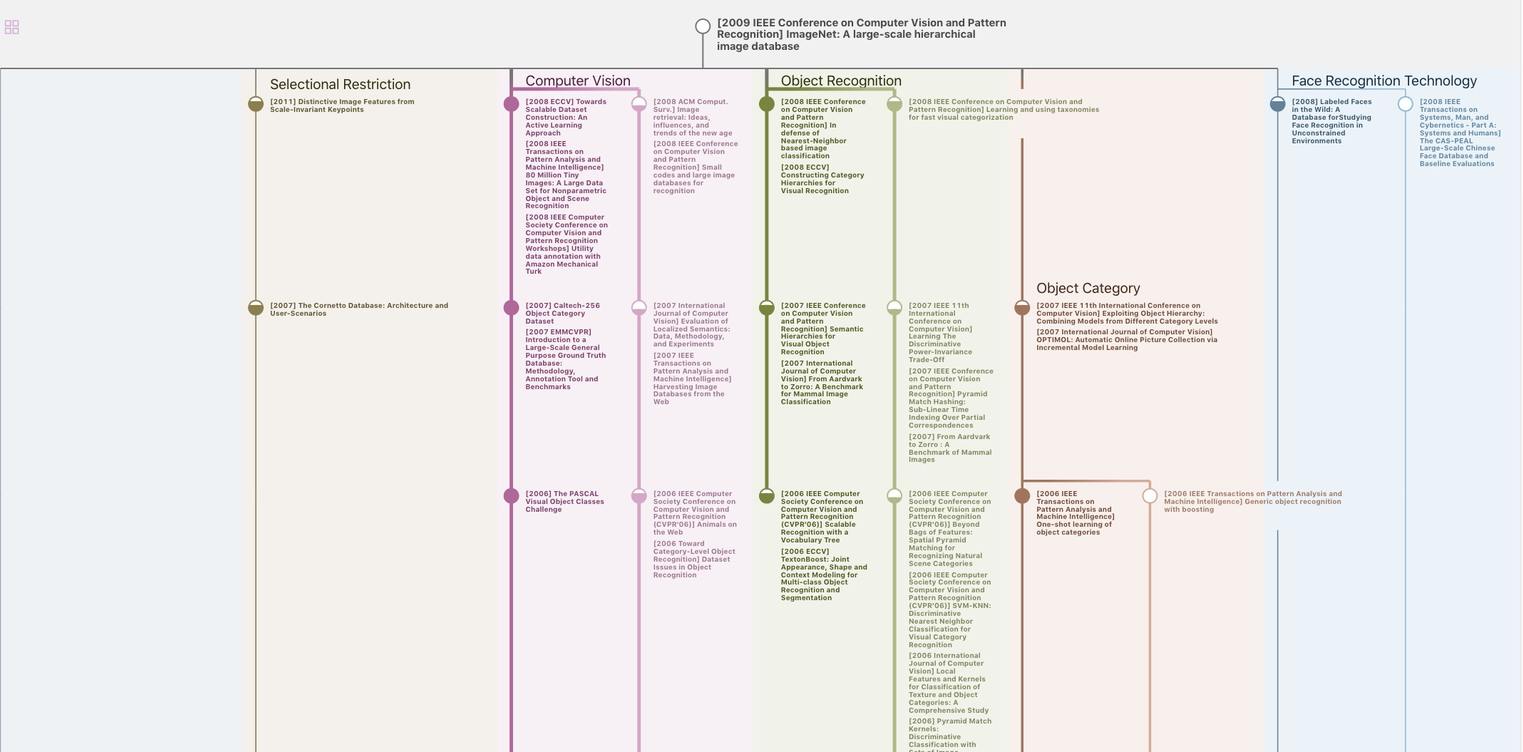
生成溯源树,研究论文发展脉络
Chat Paper
正在生成论文摘要