CGTD-Net: Channel-Wise Global Transformer-Based Dual-Branch Network for Industrial Strip Steel Surface Defect Detection
IEEE SENSORS JOURNAL(2024)
摘要
Surface defects directly affect the mechanical properties of industrial strip steel products. To evaluate the integrity of the strip steel surface, a channel-wise global Transformer-based dual-branch network (CGTD-Net) for strip steel surface defect detection, dubbed CGTD-Net, is proposed in this study. First, the strip steel surface images are preprocessed using saturation adjustment and random flipping strategies to remove unnecessary background information and improve network generalization. Second, the Swin Transformer is employed at the end of the backbone network and the negative impacts of a single channel are then mitigated by using the multichannel feature pyramid networks via Transformer, which improves the extraction ability of the global semantic information for tiny or narrow defects. Third, an edge detection branch network is constructed with a spatial-channel global attention (SCGA) module to further enhance the feature extraction on both spatial and channel information. Finally, the CGTD-Net is compared with 11 state-of-the-art methods on the NEU-DET dataset, and ablation experiments are also implemented. The comparison results, conducted on a single 3090Ti GPU, reveal that the CGTD-Net achieves a mean intersection over union (mIoU) of 75.16% at 178 frames/s, outperforming other methods. The ablation experiment demonstrates that the CGTD-Net improves the mIoU by 7.83% and the F-score by 6.3% compared to the baseline.
更多查看译文
关键词
Strips,Steel,Transformers,Image edge detection,Surface morphology,Feature extraction,Sensors,Deep learning,image segmentation,mechanical property,strip steel surface defect,Transformer
AI 理解论文
溯源树
样例
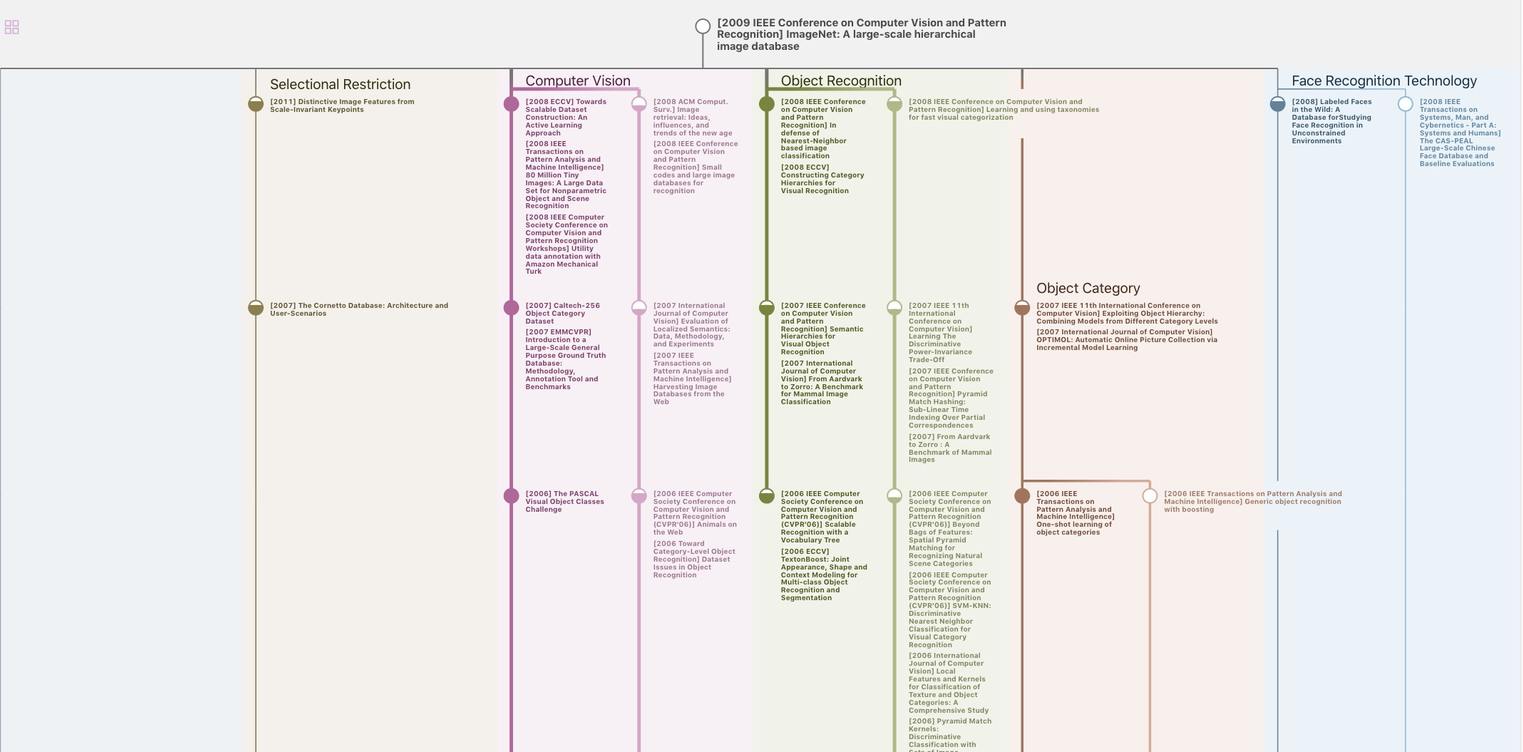
生成溯源树,研究论文发展脉络
Chat Paper
正在生成论文摘要