Bayesian Optimization Based Neural Architecture Search for Classification of Gases/Odors Mixtures
IEEE Sensors Journal(2024)
摘要
The present work demonstrates the potential of the proposed Bayesian optimization (BO). It is based on neural architecture search (NAS) for the classification of gases/odors for data-sets of diverse applications and complexities in terms of the number of analytes, features and data-points. NAS is an aspect of automated machine learning (AutoML), which explores and exploits a bounded search space of hyper-parameters to obtain optimal neural network architectures. Herein, Gaussian distribution has been used to model the search space as a probabilistic distribution and Bayesian optimization has been used as an acquisition function to guide the search process in the modeled search space. NAS has been implemented by iteratively minimizing the loss function i.e., categorical cross entropy. Furthermore, the excellent classification accuracies obtained, in hazardous gas detection, beef quality examination and vehicular exhaust monitoring are calculated to be 100%, 99.68%, and 99.46% respectively. The innovative approach establishes the robustness of the proposed BO aided NAS in obtaining optimal gases/odors classification for diverse gaseous mixture data-sets.
更多查看译文
关键词
Neural Architecture Search,Gaussian Process,Sensor Array,Bayesian Optimization,Gaseous Mixture,Over-fitting
AI 理解论文
溯源树
样例
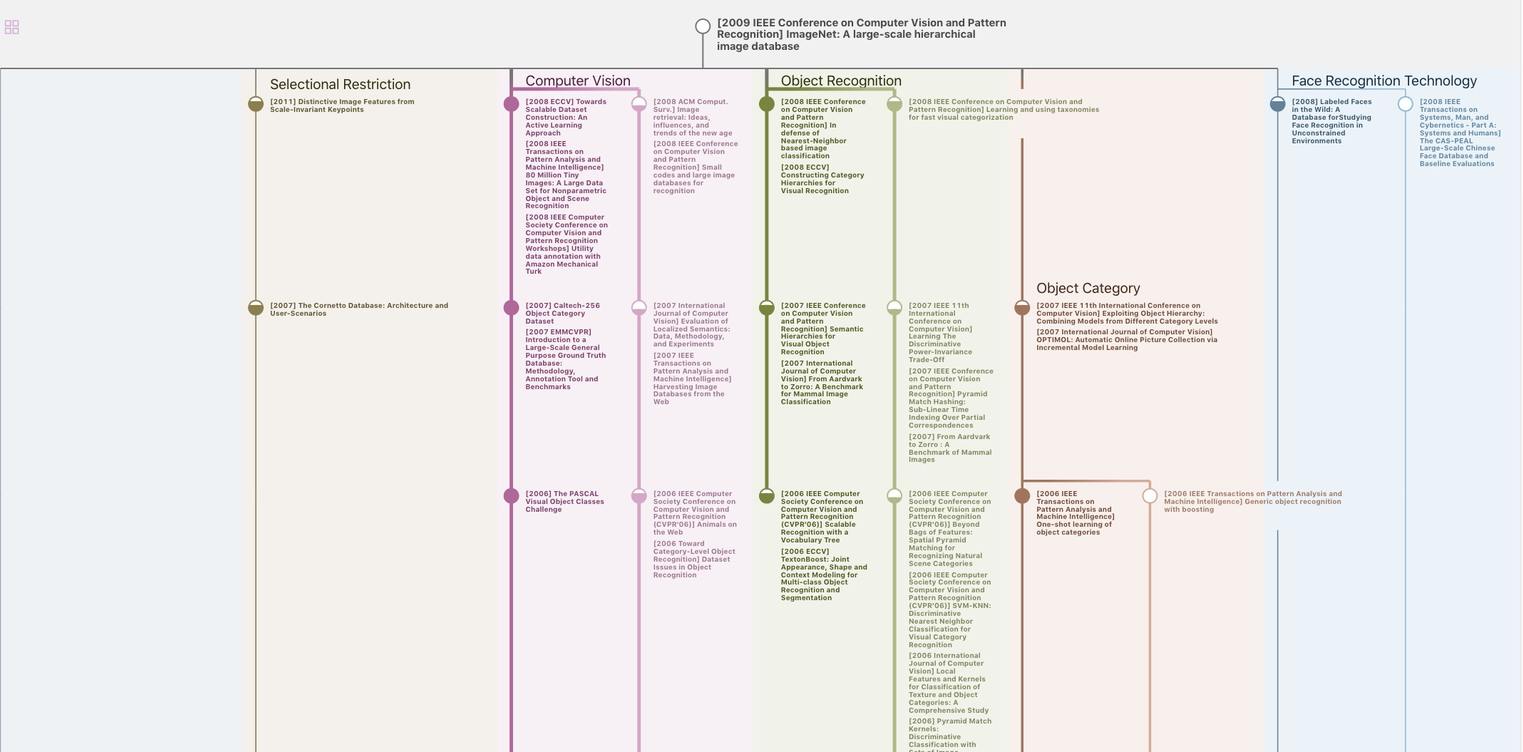
生成溯源树,研究论文发展脉络
Chat Paper
正在生成论文摘要