Intensity Prediction Model Based on Machine Learning for Regional Earthquake Early Warning
IEEE Sensors Journal(2024)
摘要
Seismic intensity plays a crucial role in influencing the decision-making process of users utilizing earthquake early warning systems (EEWs) upon receiving warning information. Improving intensity warnings’ speed and accuracy is vital. We present a straightforward and dependable model for predicting intensity, which is based only on location and magnitude information. We use the catalog of intensity data from the Japan Meteorological Agency (JMA) released as a dataset, totaling 944,877 intensity instances. To address the issue of imbalanced dataset distribution, we employ the Synthetic Minority Over-Sampling Technique (SMOTE) as a means to improve this situation. Considering the distribution of high intensity data and the importance of features in the model, we construct and jointly apply intensity prediction models for magnitude below 5.7 and above 5.7, respectively. Further, we analyze the robustness of the model by adding random errors for magnitude and location information. We test the transfer capability of the proposed model with four earthquake events in China. Further, we use 466 seismic events (20,542 intensity instances) without published intensity data from the Kyoshin network (K-NET) as the application dataset. We simulate the phenomenon of underestimation of large earthquakes and overestimation of small earthquakes, which is used to analyze the possible application of the proposed model to EEWs. The findings indicate that the model achieves an accuracy of 97.77% when subjected to a magnitude error of 0.3 and a location error of 0.2°. Finally, we analyze the timeliness of the proposed model with a magnitude 7.4 event in 2022.
更多查看译文
关键词
Earthquake early warning,earthquake engineering,earthquake intensity,machine learning,seismic signal processing
AI 理解论文
溯源树
样例
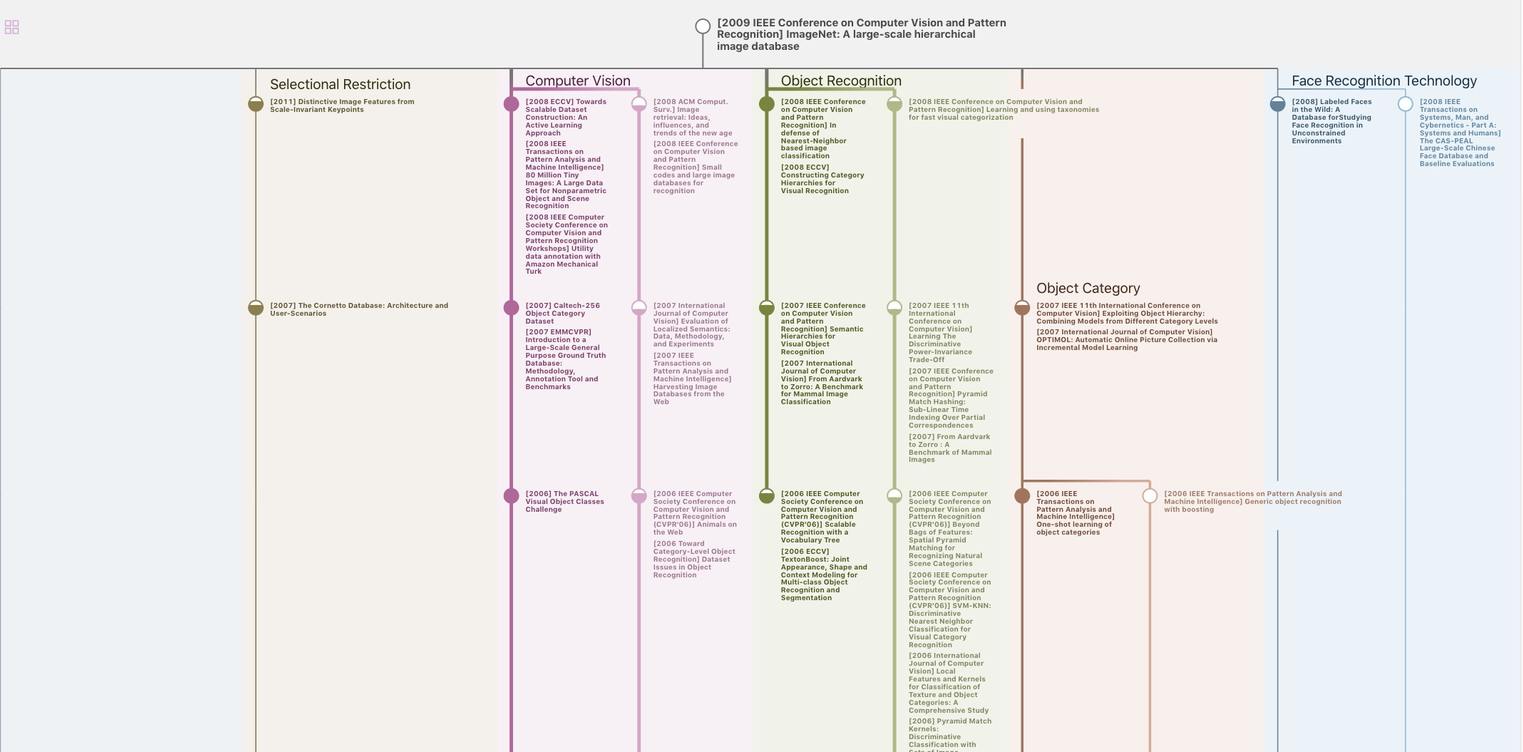
生成溯源树,研究论文发展脉络
Chat Paper
正在生成论文摘要