Collaborative Tag-Aware Graph Neural Network for Long-Tail Service Recommendation
IEEE Transactions on Services Computing(2024)
摘要
Long-tail service recommendation provides an unexpected but reasonable experience for potential developers when they construct mashups. However, the lack of available information makes it difficult to recommend highly relevant long-tail services for target mashups. Collaborative tagging systems employ extensive tag records to replenish the available information of long-tail services, whereas existing tag-aware approaches are unable to learn multi-aspect embeddings from graphs with different structures and relationships for long-tail services. To this end, we present a novel approach, namely collaborative tag-aware graph neural network, to recommend satisfactory long-tail services by extracting multi-aspect embeddings. Firstly, a tensor decomposition is executed to parameterize mashups, tags, and services as low-dimensional vector representations, respectively. Then, an interaction-aware heterogeneous neighbor aggregation is presented to aggregate both neighboring node features and interaction strength to enhance the embedding quality of long-tail services. Next, a diffusion-aware homogeneous neighbor aggregation is proposed to assign higher weights for long-tail neighboring nodes so as to reduce the influence of popular neighboring nodes during the aggregation process. Furthermore, a type-aware attention network is employed to update the final node embedding by aggregating multi-aspect embeddings. Experimental results on two real-world Web service datasets indicate that the proposed approach generates superior accuracy and diversity than state-of-the-art approaches in the aspect of long-tail service recommendation.
更多查看译文
关键词
Attention mechanism,collaborative tagging,graph neural network,long-tail service recommendation
AI 理解论文
溯源树
样例
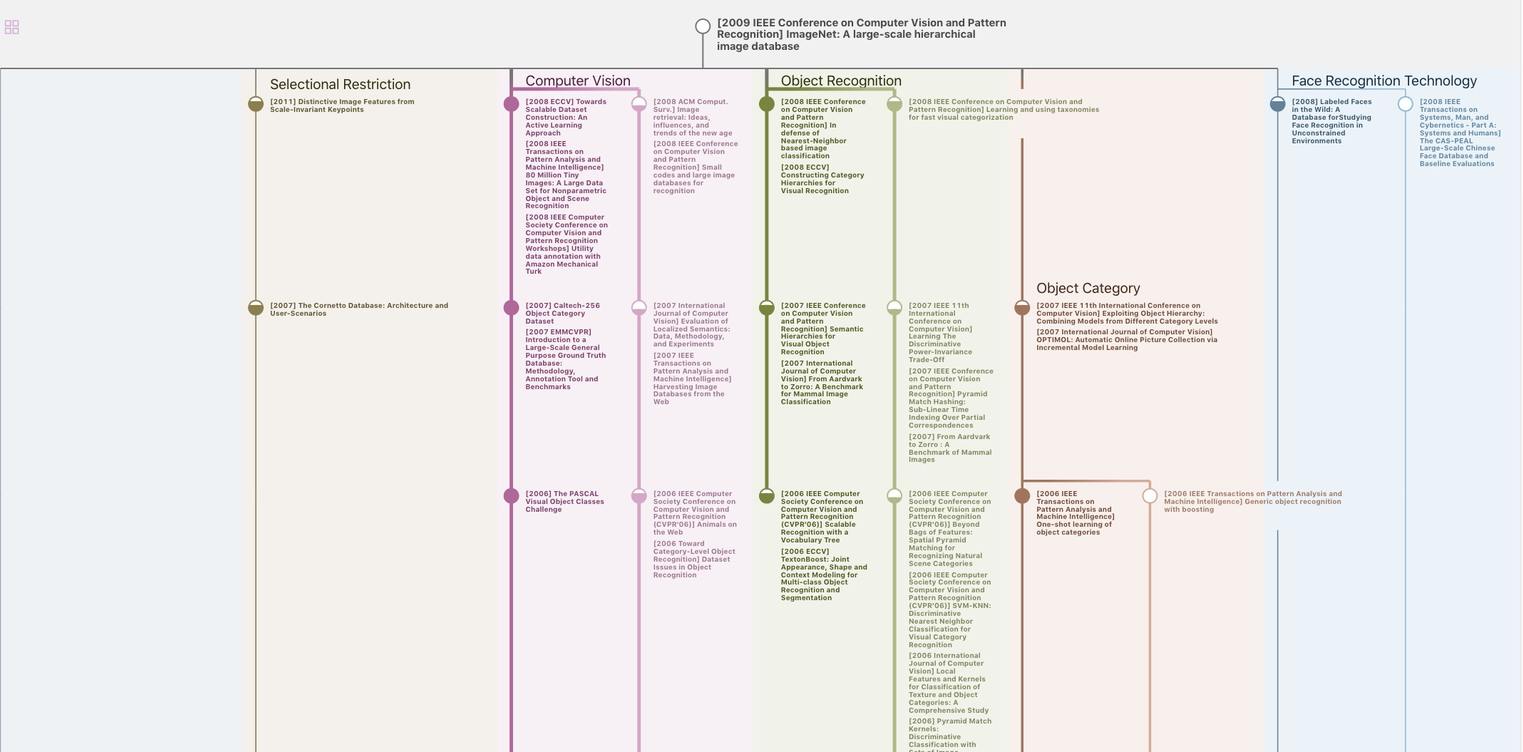
生成溯源树,研究论文发展脉络
Chat Paper
正在生成论文摘要