L-TLA: A Lightweight Driver Distraction Detection Method Based on Three-Level Attention Mechanisms
IEEE TRANSACTIONS ON RELIABILITY(2024)
摘要
Driver distraction is a significant factor leading to traffic accidents. Detecting driver distraction is crucial for the development of advanced driver assistance systems (ADAS). With the development of deep learning techniques, advanced computer vision technologies have been continuously applied for driver distraction detection. To date, most distraction detection approaches cannot well be adapted to the distraction behaviors that are not included in the training dataset. To address this problem, we propose a lightweight driver distraction detection method using semisupervised contrastive learning. Unlike other studies that rely on large-scale models, a lightweight vision transformer with convolutional neural network (CNN) obtained by knowledge distillation is adapted to extract features, and the design of the dual-stream backbone network increases the generalization ability without increasing the computational burden. Furthermore, the combination of three-level attention mechanisms (i.e., channel-level, spatial-level, and batch-level) enhances the representative power of the model. Both depth and RGB datasets are used to train and test our proposed method. The experimental results show that our method shows superior performance in comparison with other state-of-the-art methods. Its lightweight architecture is suitable for practical applications. This study contributes to the development of ADAS and provides a new perspective on driver distraction detection.
更多查看译文
关键词
Autonomous vehicles,dual-stream network,lightweight vision transformer,semisupervised contrastive learning
AI 理解论文
溯源树
样例
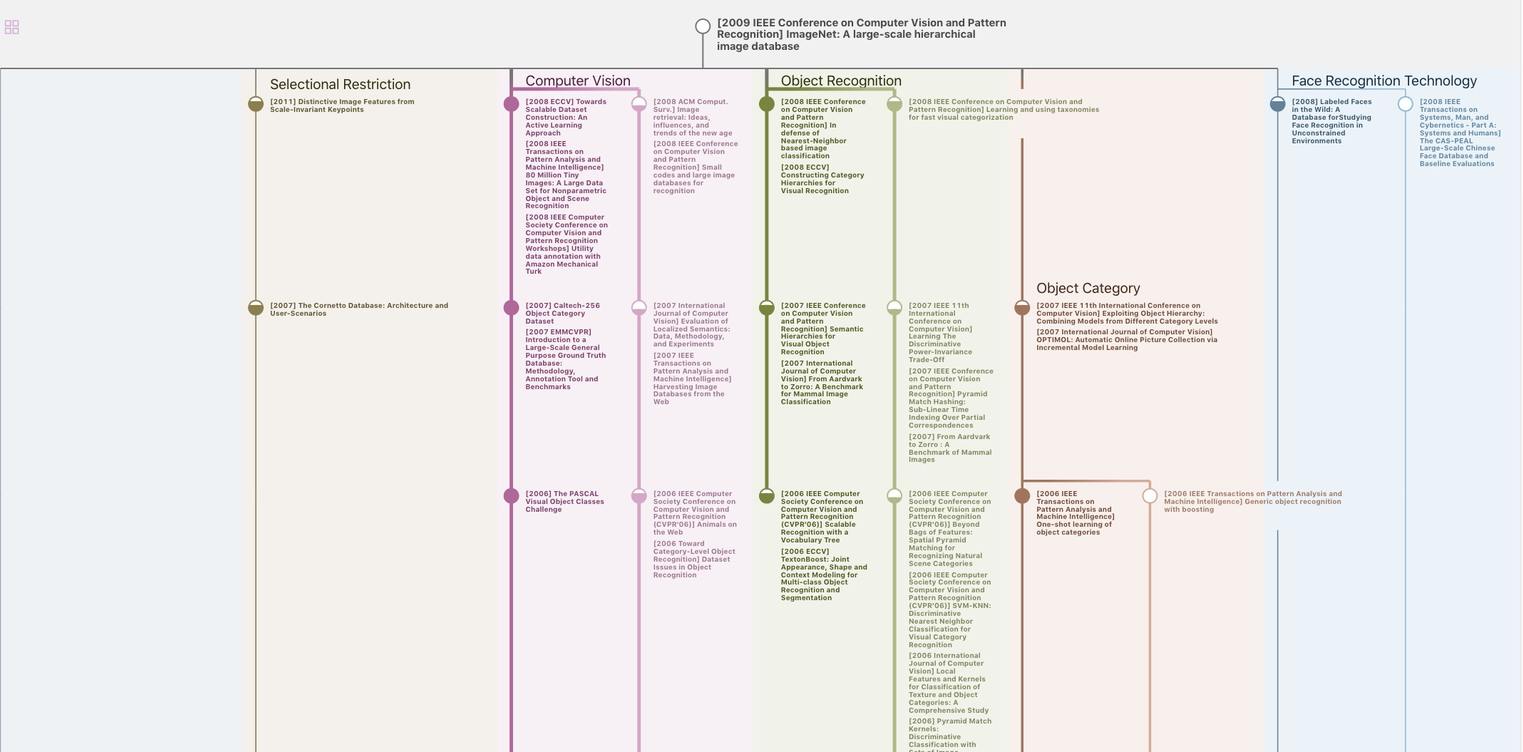
生成溯源树,研究论文发展脉络
Chat Paper
正在生成论文摘要